Efficient discovery of contrast subspaces for object explanation and characterization
Knowledge and Information Systems(2015)
摘要
We tackle the novel problem of mining contrast subspaces. Given a set of multidimensional objects in two classes C_+ and C_- and a query object o , we want to find the top- k subspaces that maximize the ratio of likelihood of o in C_+ against that in C_- . Such subspaces are very useful for characterizing an object and explaining how it differs between two classes. We demonstrate that this problem has important applications, and, at the same time, is very challenging, being MAX SNP-hard. We present CSMiner, a mining method that uses kernel density estimation in conjunction with various pruning techniques. We experimentally investigate the performance of CSMiner on a range of data sets, evaluating its efficiency, effectiveness, and stability and demonstrating it is substantially faster than a baseline method.
更多查看译文
关键词
Contrast subspace, Kernel density estimation, Likelihood contrast
AI 理解论文
溯源树
样例
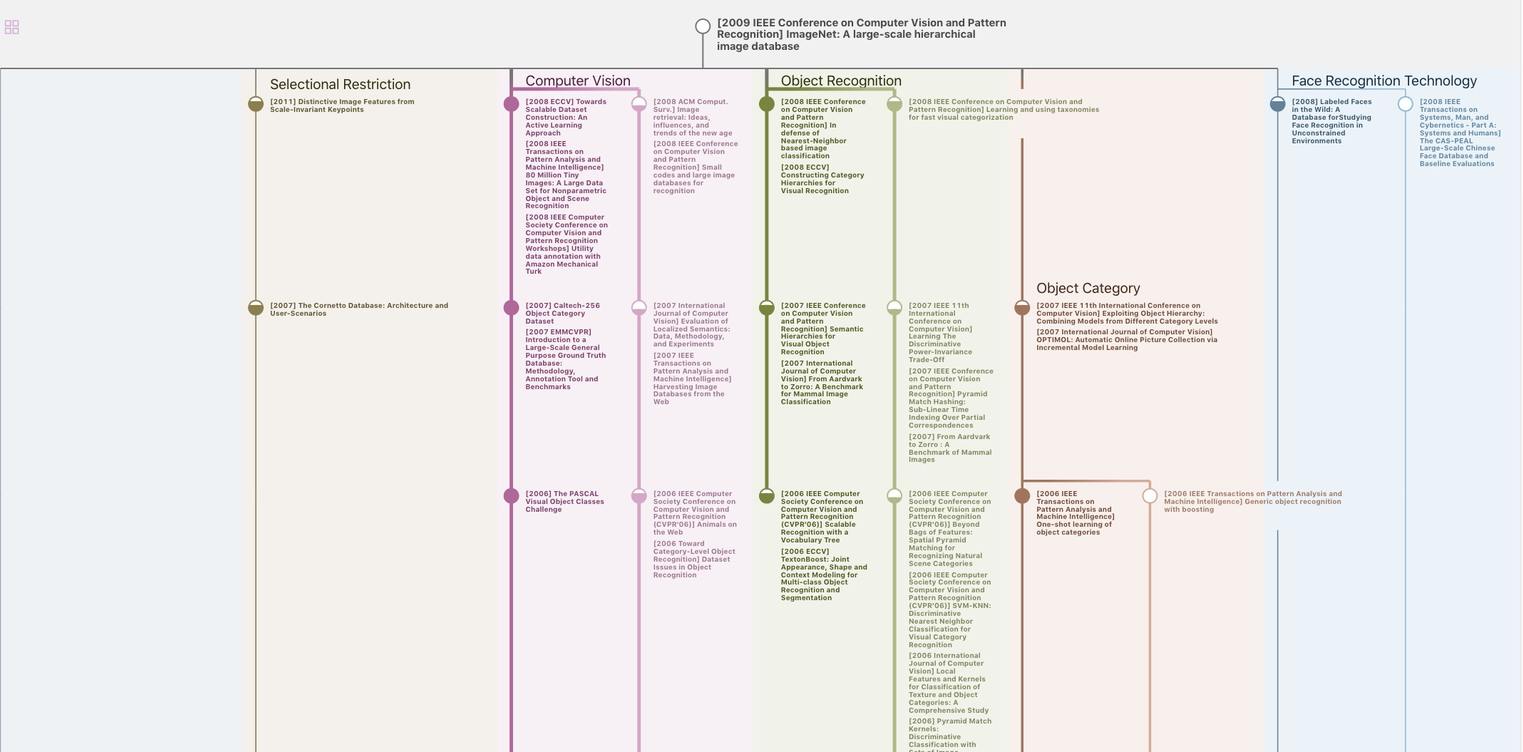
生成溯源树,研究论文发展脉络
Chat Paper
正在生成论文摘要