N-dimensional Markov random field prior for cold-start recommendation.
Neurocomputing(2016)
摘要
A recommender system is a commonly used technique to improve user experience in e-commerce applications. One of the popular recommender methods is Matrix Factorization (MF) that learns the latent profile of both users and items. However, if the historical ratings are not available, the latent profile will draw from a zero-mean Gaussian prior, resulting in uninformative recommendations. To deal with this issue, we propose using an n-dimensional Markov random field as the prior of matrix factorization (called mrf-MF). In the Markov random field, the attribute (such as age, occupation of users and genre, release year of items) is considered as the site and the latent profile, the random variable. Through the prior, new users or items will be recommended according to its neighbors. The proposed model is suitable for three types of cold-start recommendation: (1) recommend new items to existing users; (2) recommend new users for existing items; (3) recommend new items to new users. The proposed model is assessed on two movie datasets, Movielens 100K and Movielens 1M. Experimental results show that it can effectively solve each of the three cold-start problems and outperforms several matrix factorization based methods.
更多查看译文
关键词
Recommender system,Cold-start,Markov Random Field
AI 理解论文
溯源树
样例
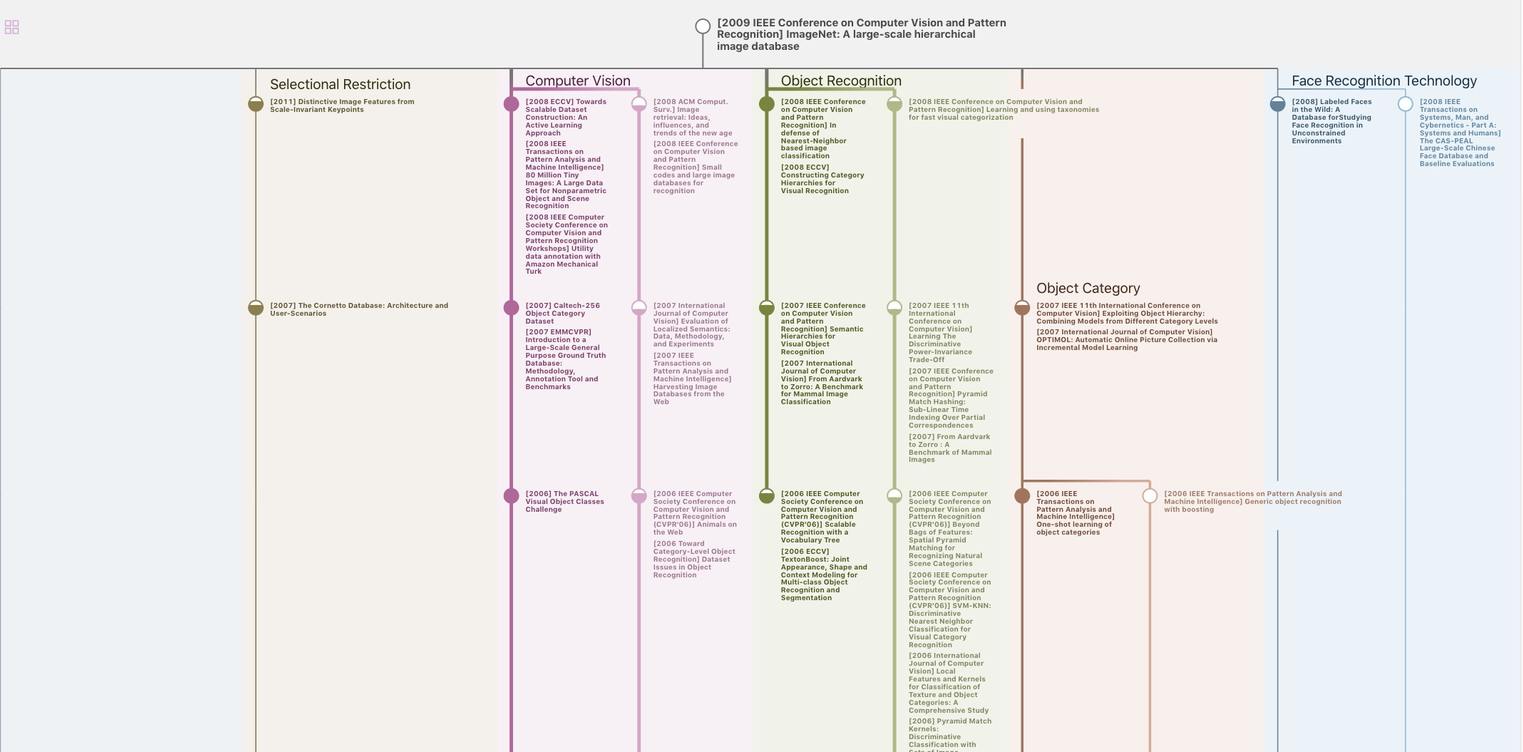
生成溯源树,研究论文发展脉络
Chat Paper
正在生成论文摘要