An efficient concept detection system via sparse ensemble learning.
Neurocomputing(2015)
摘要
In this paper, we present an efficient concept detection system based on a novel bag of words extraction method and sparse ensemble learning. The presented system can efficiently build the concept detectors upon large scale image dataset, and achieve real-time concept detection on unseen images with the state-of-the-arts accuracy. To do so, we first develop an efficient bag of visual words (BoW) construction method based on sparse non-negative matrix factorization (NMF) and GPU enabled SIFT feature extraction. We then develop a sparse ensemble learning method to build the detection model, which drastically reduces learning time in order of magnitude over traditional methods like Support Vector Machine. To overcome the difficulty of manual annotation of training dataset, we construct a large training set with both pseudo relevance feedback of negative samples and interactive feedback of positive samples. Experiments on TRECVID 2012 dataset and MIRFlickr-1M dataset show both efficiency and effectiveness of our system.
更多查看译文
关键词
Ensemble learning,Visual concept detection,Bag of visual words,Sparse coding,Pseudo relevance feedback
AI 理解论文
溯源树
样例
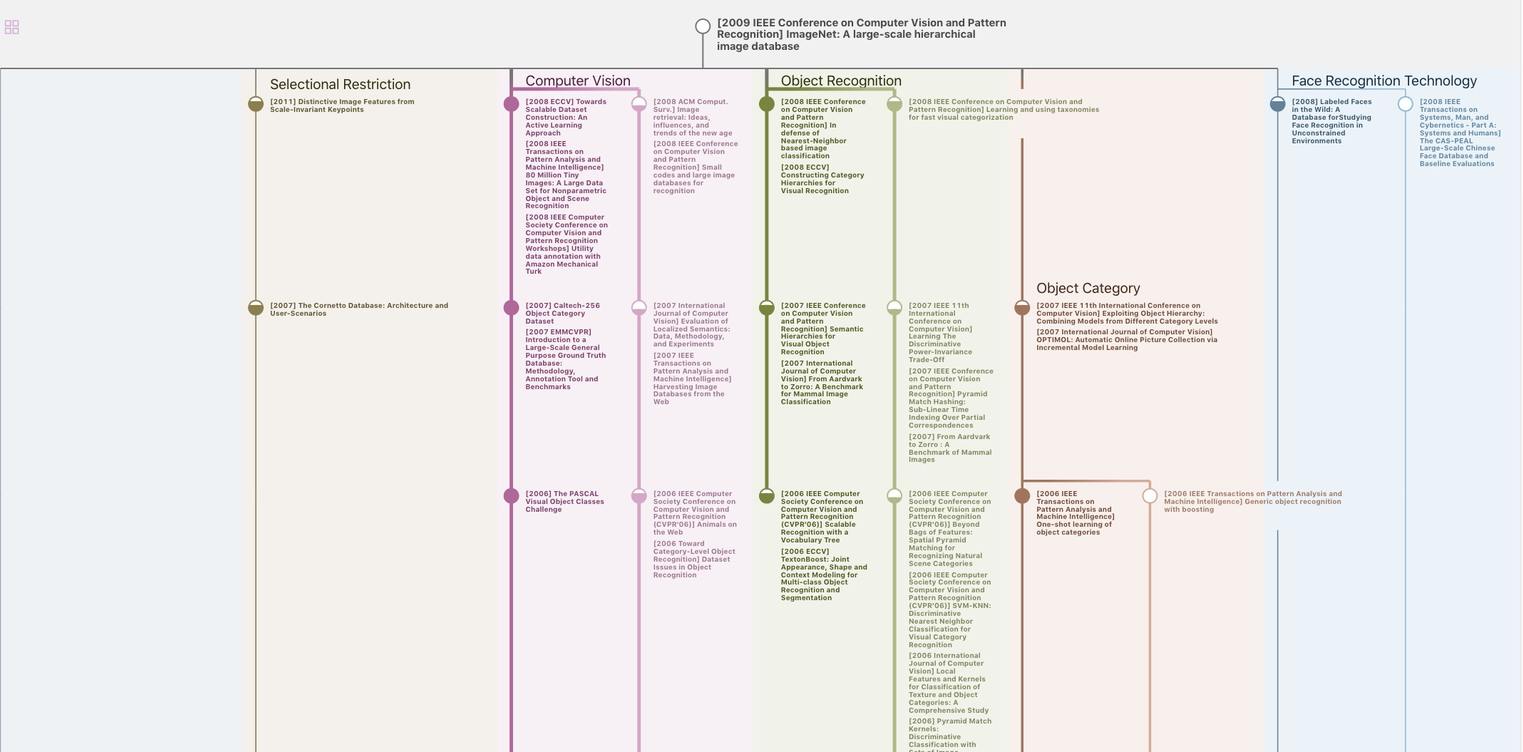
生成溯源树,研究论文发展脉络
Chat Paper
正在生成论文摘要