Predicting primary categories of business listings for local search ranking
Neurocomputing(2015)
摘要
We consider the problem of identifying primary categories of a business listing among the categories provided by the owner of the business, in order to enhance local search and browsing. The category information submitted by business owners cannot be trusted with absolute certainty since they may purposefully add some secondary or irrelevant categories to increase recall in local search results, which makes category search very challenging for local search engines. Thus, identifying primary categories of a business is a crucial problem in local search. This problem can be cast as a multi-label classification problem with a large number of categories. However, the large scale of the problem makes it infeasible to use conventional supervised-learning-based text categorization approaches.We propose a large-scale classification framework that leverages multiple types of classification labels to produce a highly accurate classifier with fast training time. We effectively combine the complementary label sources to refine prediction. The experimental results indicate that our framework achieves very high precision and recall and outperforms a competitive baseline using a centroid-based method.We also propose a new ranking feature based on the mapping of queries and documents to category space and show that the new feature leads to ranking relevance improvements for local search.
更多查看译文
关键词
Vertical search,Text categorization,Primary category,Ranking relevance
AI 理解论文
溯源树
样例
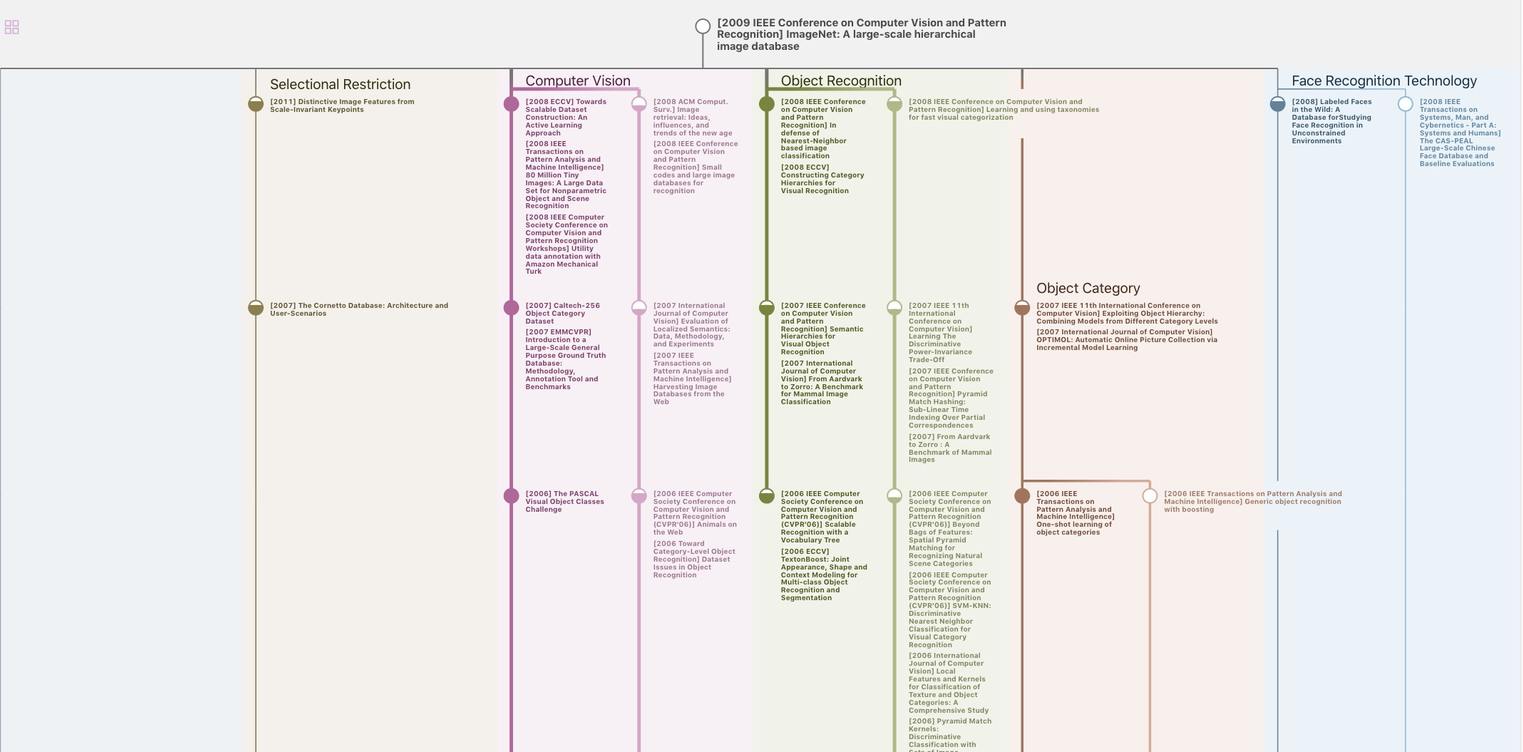
生成溯源树,研究论文发展脉络
Chat Paper
正在生成论文摘要