Non-negative dictionary based sparse representation classification for ear recognition with occlusion.
Neurocomputing(2016)
摘要
By introducing an identity occlusion dictionary to encode the occluded part on the source image, sparse representation based classification has shown good performance on ear recognition under partial occlusion. However, large number of atoms of the conventional occlusion dictionary brings expensive computational load to the SRC model solving. In this paper, we propose a non-negative dictionary based sparse representation and classification scheme for ear recognition. The non-negative dictionary includes the Gabor features dictionary extracted from the ear images, and non-negative occlusion dictionary learned from the identity occlusion dictionary. A test sample with occlusion can be sparsely represented over the Gabor feature dictionary and the occlusion dictionary. The sparse coding coefficients are noted with non-negativity and much more sparsity, and the non-negative dictionary has shown increasing discrimination ability. Experimental results on the USTB ear database show that the proposed method performs better than existing ear recognition methods under partial occlusion based on SRC.
更多查看译文
关键词
Sparse representation classification,Non-negative occlusion dictionary,Ear recognition with occlusion
AI 理解论文
溯源树
样例
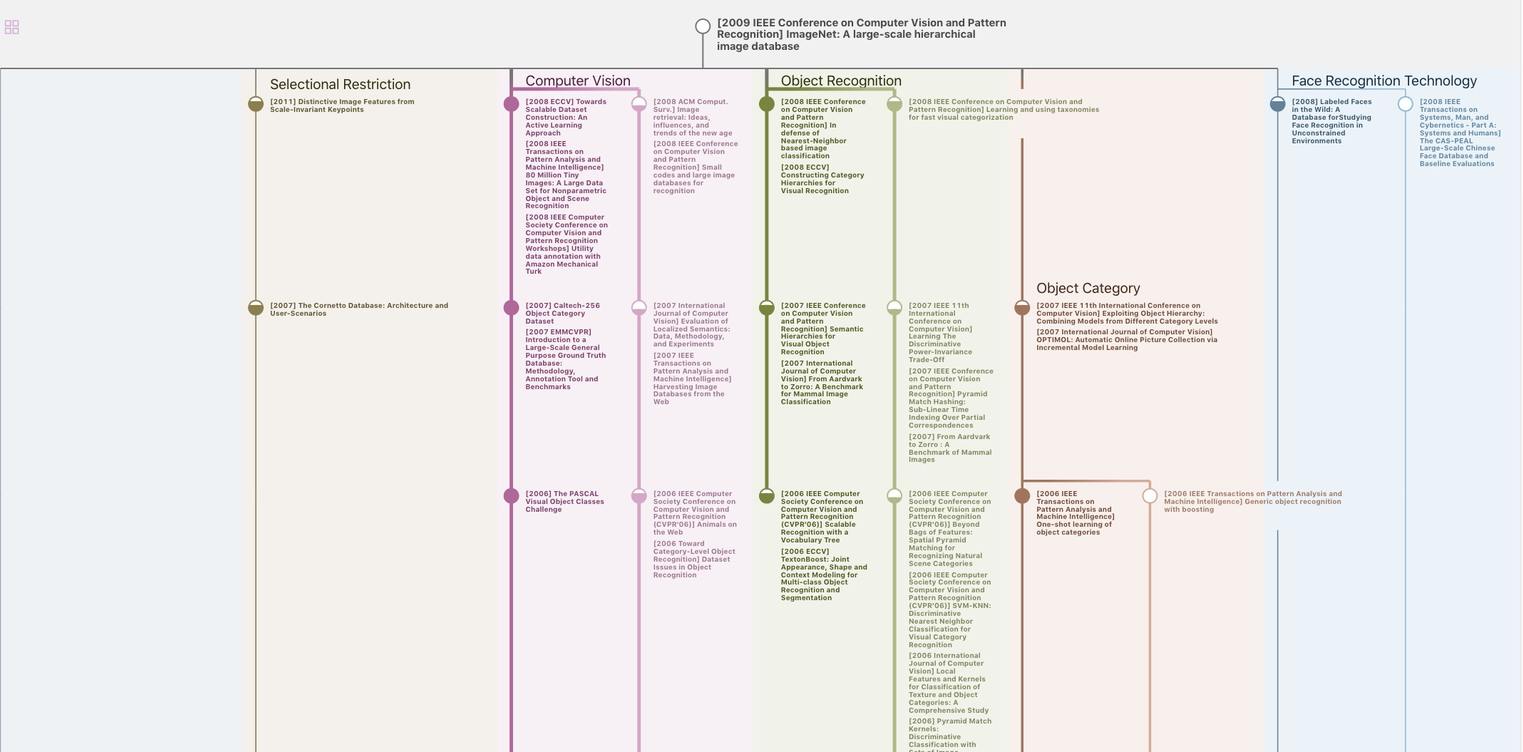
生成溯源树,研究论文发展脉络
Chat Paper
正在生成论文摘要