Improving Random Forest With Ensemble of Features and Semisupervised Feature Extraction
IEEE Geoscience and Remote Sensing Letters(2015)
摘要
In this letter, we propose a novel approach for improving Random Forest (RF) in hyperspectral image classification. The proposed approach combines the ensemble of features and the semisupervised feature extraction (SSFE) technique. The main contribution of our approach is to construct an ensemble of RF classifiers. In this way, the feature space is divided into several disjoint feature subspaces. Then, the feature subspaces induced by the SSFE technique are used as the input space to an RF classifier. This method is compared with a regular RF and an RF with the reduced features by the SSFE on two real hyperspectral data sets, showing an improved performance in ill-posed, poor-posed, and well-posed conditions. An additional study shows that the proposed method is less sensitive to the parameters.
更多查看译文
关键词
Classification,ensemble learning,hyperspectral image,Random Forest (RF),semisupervised feature extraction (SSFE)
AI 理解论文
溯源树
样例
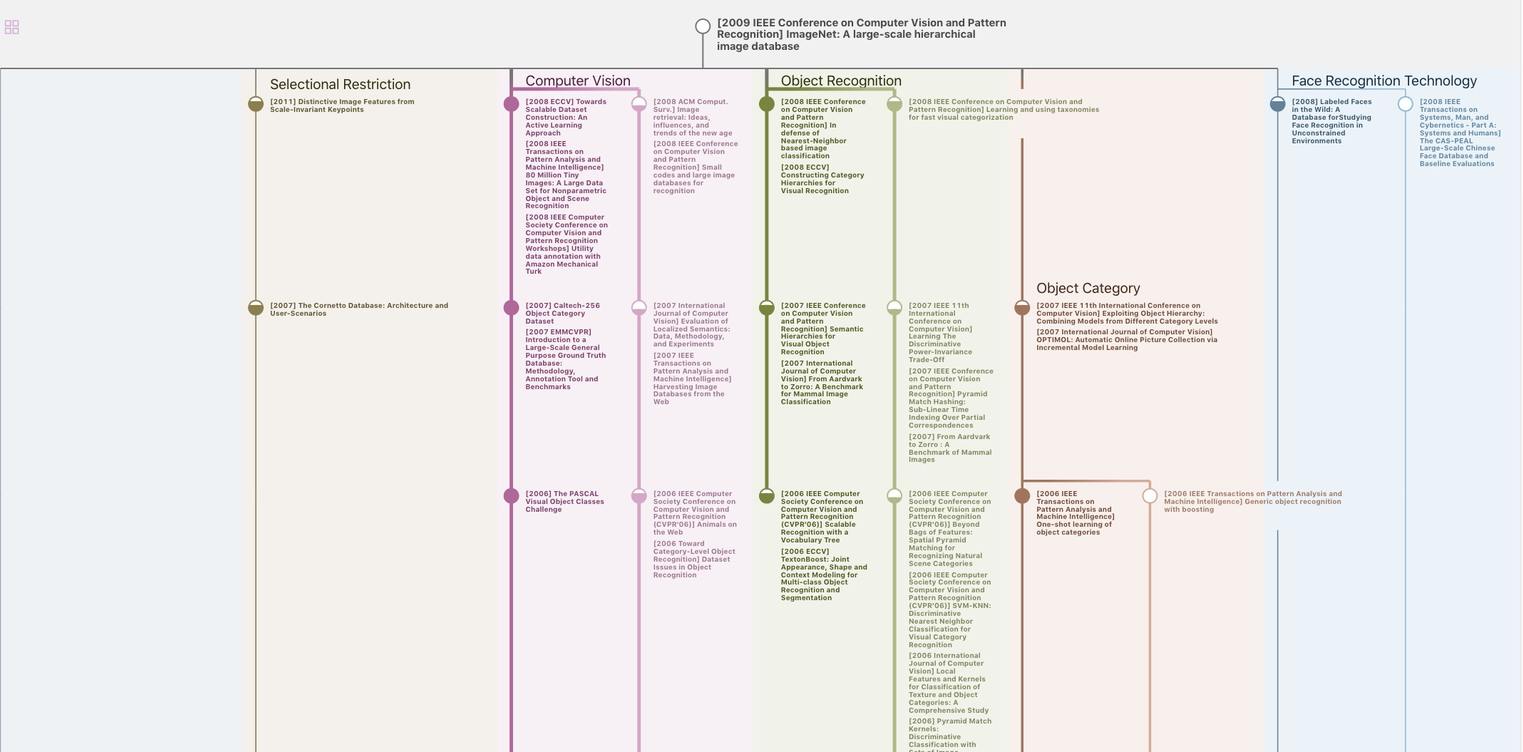
生成溯源树,研究论文发展脉络
Chat Paper
正在生成论文摘要