Challenges in clinical natural language processing for automated disorder normalization
Journal of Biomedical Informatics(2015)
摘要
Display Omitted Disorder normalization in clinical text has wide-ranging applications.Clinical normalizers must handle ad-hoc formatting, jargon, and ambiguous acronyms.Disorder vocabulary is richer in clinical text than biomedical abstracts.Normalization with pairwise learning to rank handles rich vocabulary.Further normalization improvements require improved named entity recognition. BackgroundIdentifying key variables such as disorders within the clinical narratives in electronic health records has wide-ranging applications within clinical practice and biomedical research. Previous research has demonstrated reduced performance of disorder named entity recognition (NER) and normalization (or grounding) in clinical narratives than in biomedical publications. In this work, we aim to identify the cause for this performance difference and introduce general solutions. MethodsWe use closure properties to compare the richness of the vocabulary in clinical narrative text to biomedical publications. We approach both disorder NER and normalization using machine learning methodologies. Our NER methodology is based on linear-chain conditional random fields with a rich feature approach, and we introduce several improvements to enhance the lexical knowledge of the NER system. Our normalization method - never previously applied to clinical data - uses pairwise learning to rank to automatically learn term variation directly from the training data. ResultsWe find that while the size of the overall vocabulary is similar between clinical narrative and biomedical publications, clinical narrative uses a richer terminology to describe disorders than publications. We apply our system, DNorm-C, to locate disorder mentions and in the clinical narratives from the recent ShARe/CLEF eHealth Task. For NER (strict span-only), our system achieves precision=0.797, recall=0.713, f-score=0.753. For the normalization task (strict span+concept) it achieves precision=0.712, recall=0.637, f-score=0.672. The improvements described in this article increase the NER f-score by 0.039 and the normalization f-score by 0.036. We also describe a high recall version of the NER, which increases the normalization recall to as high as 0.744, albeit with reduced precision. DiscussionWe perform an error analysis, demonstrating that NER errors outnumber normalization errors by more than 4-to-1. Abbreviations and acronyms are found to be frequent causes of error, in addition to the mentions the annotators were not able to identify within the scope of the controlled vocabulary. ConclusionDisorder mentions in text from clinical narratives use a rich vocabulary that results in high term variation, which we believe to be one of the primary causes of reduced performance in clinical narrative. We show that pairwise learning to rank offers high performance in this context, and introduce several lexical enhancements - generalizable to other clinical NER tasks - that improve the ability of the NER system to handle this variation. DNorm-C is a high performing, open source system for disorders in clinical text, and a promising step toward NER and normalization methods that are trainable to a wide variety of domains and entities. (DNorm-C is open source software, and is available with a trained model at the DNorm demonstration website: http://www.ncbi.nlm.nih.gov/CBBresearch/Lu/Demo/tmTools/#DNorm.)
更多查看译文
关键词
Electronic health records,Information extraction,Natural language processing
AI 理解论文
溯源树
样例
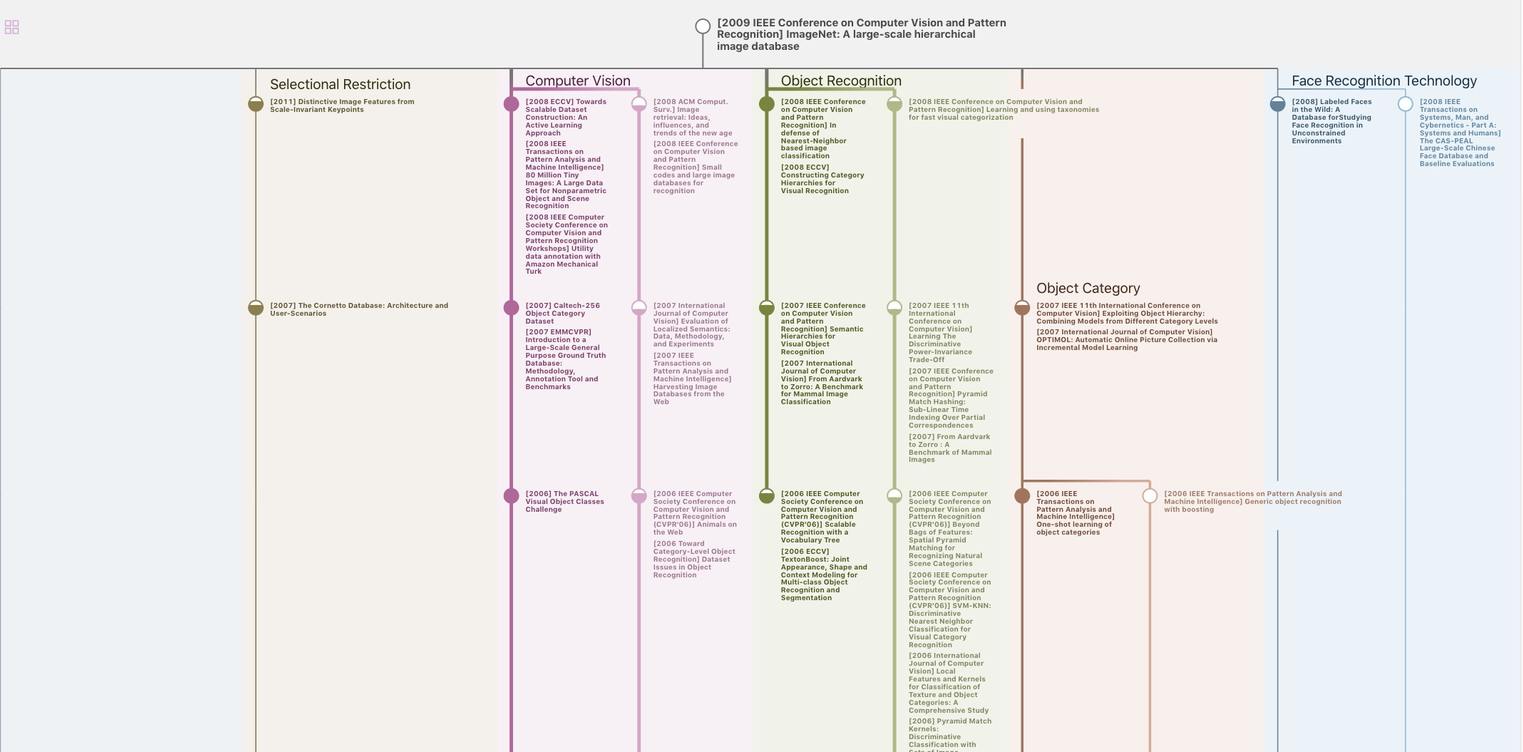
生成溯源树,研究论文发展脉络
Chat Paper
正在生成论文摘要