Scalable Data-Coupled Clustering for Large Scale WSN
IEEE Trans. Wireless Communications(2015)
摘要
Self-Organizing Algorithms (SOAs) for Wireless Sensor Networks (WSNs) usually seek to increase the lifetime, to minimize unnecessary transmissions or to maximize the transport capacity. The goal left out in the design of this type of algorithms is the capability of the WSN to ensure an accurate reconstruction of the sensed field while maintaining the selforganization. In this work, we formulate a general framework where the data from the resulting clusters ensures the wellposedness of the signal processing problem in the cluster. We develop the Second-Order Data-Coupled Clustering (SODCC) algorithm and the Distributed Compressive-Projections Principal Component Analysis (D-CPPCA) algorithm, that use secondorder statistics. The condition to form a cluster is that D-CPCCA does not fail to resolve the Principal Components in any given cluster. We show that SODCC is scalable and has similar or better message complexity than other well-known SOAs. We validate these results with extensive computer simulations using an actual LS-WSN. We also show that the performance of SODCC is, comparative to other state-of-the-art SOAs, better at any compression rate and needs no prior adjustment of any parameter. Finally, we show that SODCC compares well to other energy efficient clustering algorithms in terms of energy consumption while excelling in data reconstruction Average SNR.
更多查看译文
关键词
WSN, self-organization, data-coupled clustering, compressed projections, principal component analysis
AI 理解论文
溯源树
样例
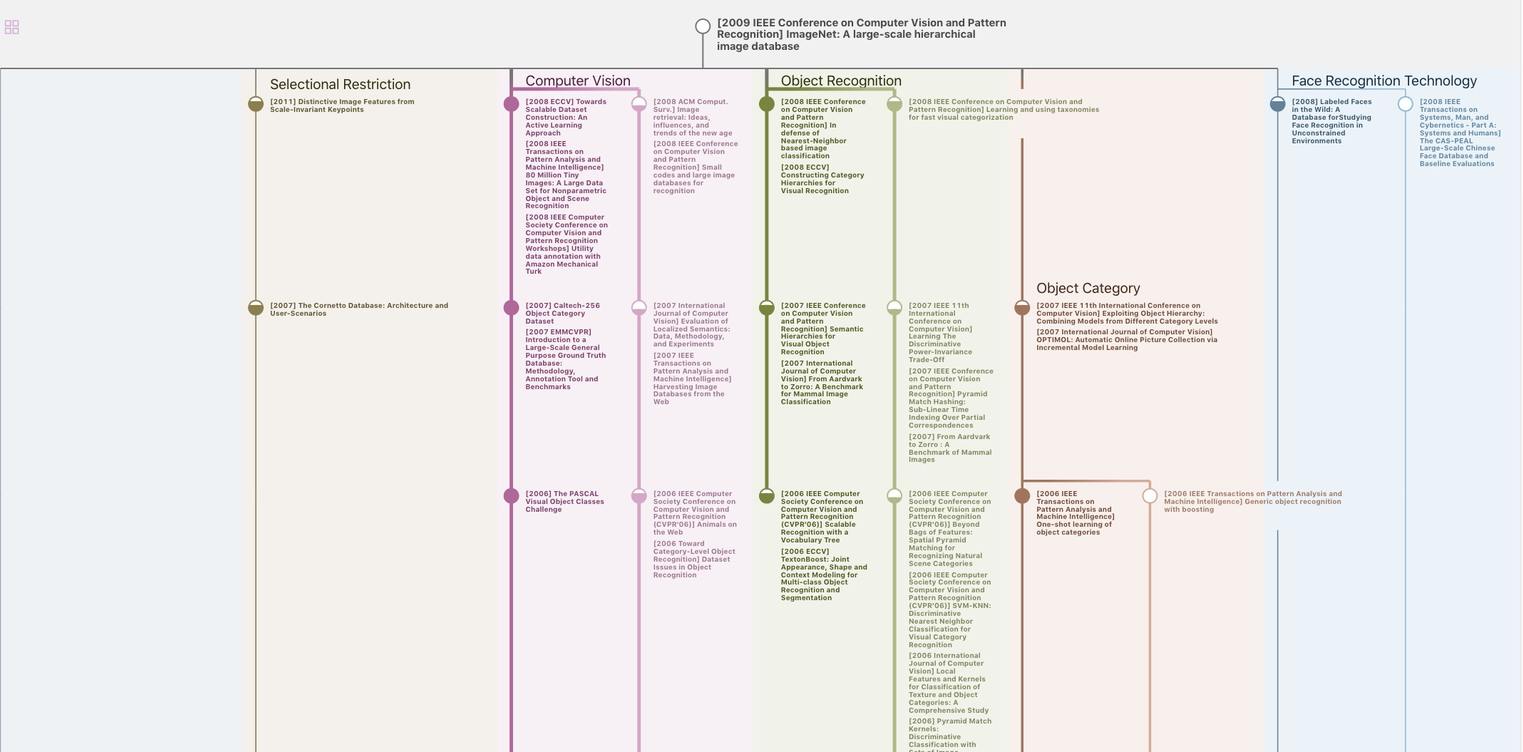
生成溯源树,研究论文发展脉络
Chat Paper
正在生成论文摘要