Using multiobjective optimization for biclustering microarray data
Applied Soft Computing(2015)
摘要
Graphical abstractDisplay Omitted HighlightsA new multiobjective modeling for the biclustering problem.A new hybrid multiobjective algorithm gradually conceived for best results.Extracting relevant biclusters with large sizes compared to classical methods. Microarray data analysis is a challenging problem in the data mining field. Actually, it represents the expression levels of thousands of genes under several conditions. The analysis of this data consists on discovering genes that share similar expression patterns across a sub-set of conditions. In fact, the extracted informations are submatrices of the microarray data that satisfy a coherence constraint. These submatrices are called biclusters, while the process of extracting them is called biclustering.Since its first application to the analysis of microarray 1], many modeling and algorithms have been proposed to solve it. In this work, we propose a new multiobjective model and a new metaheuristic HMOBIibea for the biclustering problem. Results of the proposed method are compared to those of other existing algorithms and the biological relevance of the extracted information is validated. The experimental results show that our method extracts very relevant biclusters, with large sizes with respect to existing methods.
更多查看译文
关键词
Biclustering problem,Gene expression data,Evolutionary algorithm,Multiobjective combinatorial optimization
AI 理解论文
溯源树
样例
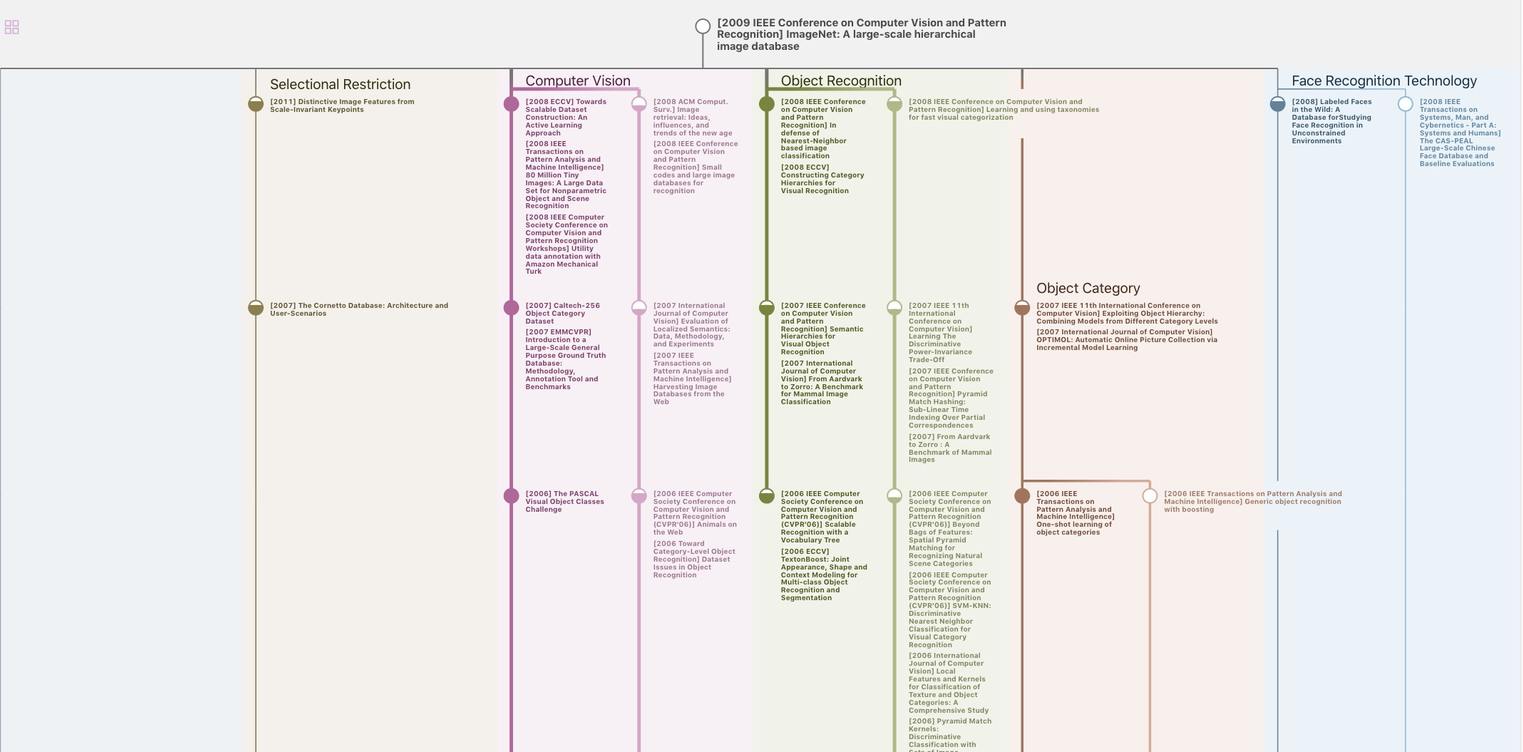
生成溯源树,研究论文发展脉络
Chat Paper
正在生成论文摘要