Gray Wolf Optimizer for hyperspectral band selection.
Applied Soft Computing(2016)
摘要
In this paper, we propose a new optimization-based framework to reduce the dimensionality of hyperspectral images. One of the most problems in hyperspectral image classification is the Hughes phenomenon caused by the irrelevant spectral bands and the high correlation between the adjacent bands. The problematic is how to find the relevant bands to classify the pixels of hyperspectral image without reducing the classification accuracy rate. We propose to reformulate the problem of band selection as a combinatorial problem by modeling an objective function based on class separability measures and the accuracy rate. We use the Gray Wolf Optimizer, which is a new meta-heuristic algorithm more efficient than Practical Swarm Optimization, Gravitational Search Algorithm, Differential Evolution, Evolutionary Programming and Evolution Strategy. The experimentations are performed on three widely used benchmark hyperspectral datasets. Comparisons with the state-of-the-art approaches are also conducted. The analysis of the results proves that the proposed approach can effectively investigate the spectral band selection problem and provides a high classification accuracy rate by using a few samples for training. (C) 2015 Elsevier B.V. All rights reserved.
更多查看译文
关键词
Band selection,Hyperspectral image classification,Gray Wolf Optimizer,Class separability,Hausdorff distance,Jeffries-Matusita distance
AI 理解论文
溯源树
样例
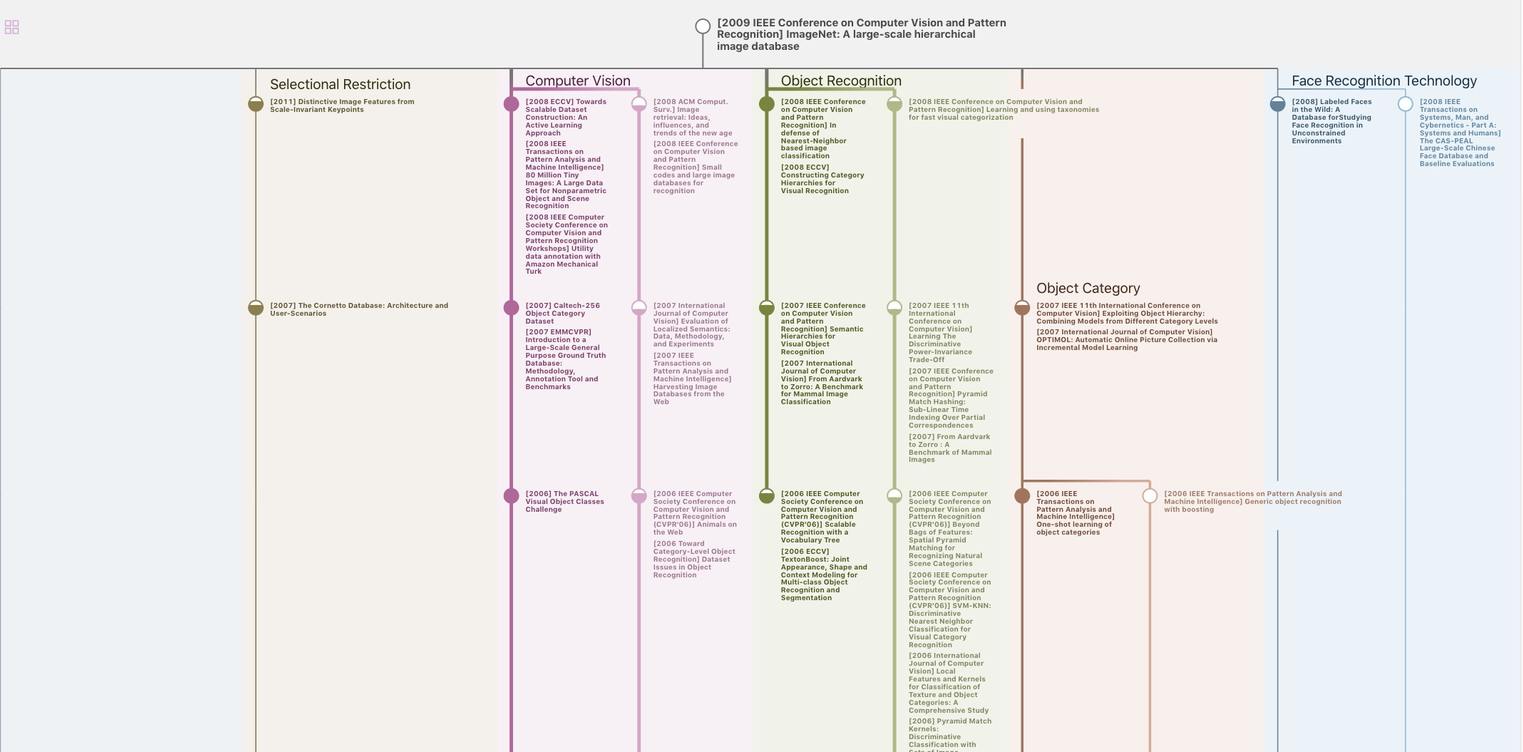
生成溯源树,研究论文发展脉络
Chat Paper
正在生成论文摘要