Lagrange Programming Neural Network Approach for Target Localization in Distributed MIMO Radar
IEEE Transactions on Signal Processing(2016)
摘要
In this paper, the problem of source localization in distributed multiple-input multiple-output (MIMO) radar using bistatic range measurements, which correspond to the sum of transmitter-to-target and target-to-receiver distances, is addressed. Our solution is based on the Lagrange programming neural network (LPNN), which is an analog neural computational technique for solving nonlinear constrained optimization problems according to the Lagrange multiplier theory. The local stability of the proposed positioning algorithm is also investigated. Furthermore, we have extended the LPNN based approach to more challenging scenarios, namely, when time synchronization among all antennas cannot be fulfilled, and there are position uncertainties in the MIMO radar transmit and receive elements. The optimality of the developed algorithms is demonstrated by comparing with the Cramér-Rao lower bound via computer simulations.
更多查看译文
关键词
MIMO radar,neural nets,radar computing,radar signal processing,Cramer-Rao lower bound,LPNN based approach,Lagrange programming neural network approach,bistatic range measurements,distributed MIMO radar,distributed multiple-input multiple-output radar,positioning algorithm,source localization,Bistatic range,Karush-Kuhn-Tucker (KKT) conditions,Lagrange programming neural network (LPNN),multiple-input multiple-output (MIMO) radar,nonlinear constrained optimization,target localization
AI 理解论文
溯源树
样例
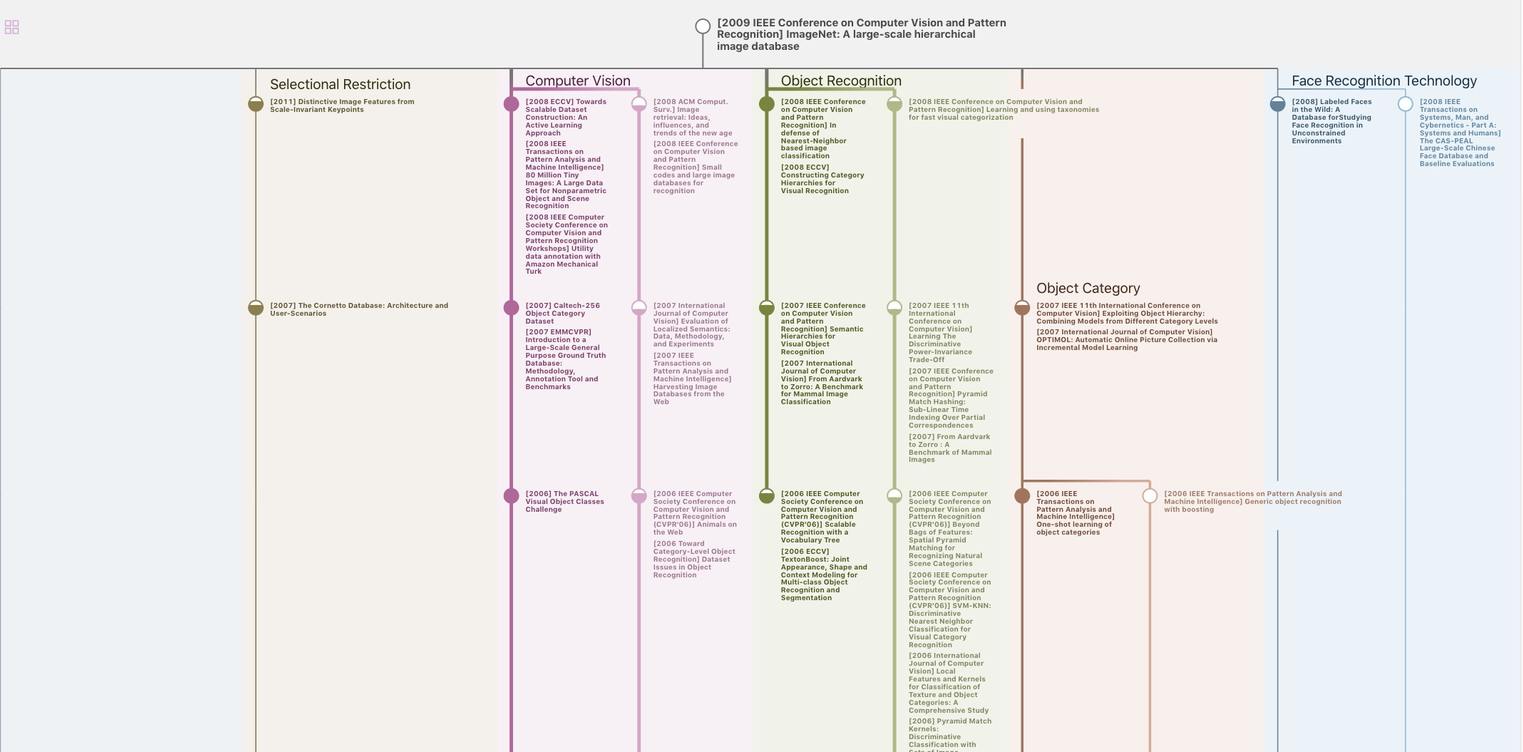
生成溯源树,研究论文发展脉络
Chat Paper
正在生成论文摘要