Superpixel Meshes For Fast Edge-Preserving Surface Reconstruction
2015 IEEE CONFERENCE ON COMPUTER VISION AND PATTERN RECOGNITION (CVPR)(2015)
摘要
Multi-View-Stereo (MVS) methods aim for the highest detail possible, however, such detail is often not required. In this work, we propose a novel surface reconstruction method based on image edges, superpixels and second-order smoothness constraints, producing meshes comparable to classic MVS surfaces in quality but orders of magnitudes faster. Our method performs per-view dense depth optimization directly over sparse 3D Ground Control Points (GCPs), hence, removing the need for view pairing, image rectification, and stereo depth estimation, and allowing for full per-image parallelization. We use Structure-from-Motion (SfM) points as GCPs, but the method is not specific to these, e.g. LiDAR or RGB-D can also be used. The resulting meshes are compact and inherently edge-aligned with image gradients, enabling good-quality lightweight per-face flat renderings. Our experiments demonstrate on a variety of 3D datasets the superiority in speed and competitive surface quality.
更多查看译文
关键词
superpixel meshes,fast edge-preserving surface reconstruction,multiview-stereo methods,MVS methods,image edges,second-order smoothness constraints,per-view dense depth optimization,sparse 3D ground control points,sparse 3D GCPs,full per-image parallelization,structure-from-motion points,SfM points,LiDAR,RGB-D,image gradients,lightweight per-face flat renderings
AI 理解论文
溯源树
样例
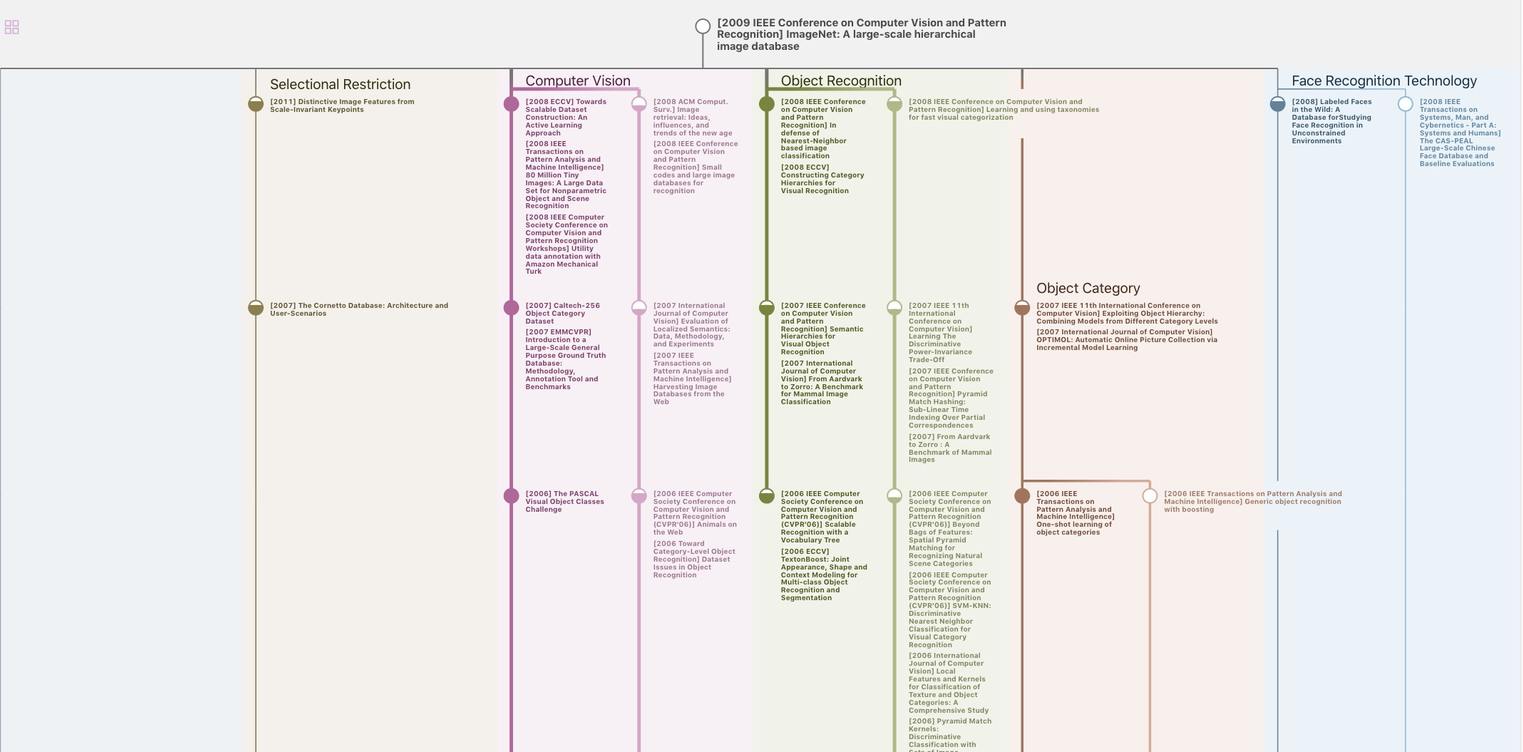
生成溯源树,研究论文发展脉络
Chat Paper
正在生成论文摘要