TU‐A‐BRB‐06: Evolving and Optimizing IMRT Treatment Plans with PARETO ‐ a Novel Treatment Planning System Based on a Multi‐Objective Genetic Algorithm
MEDICAL PHYSICS(2011)
摘要
Purpose: We introduce a novel multi‐objective treatment planning system called PARETO (Pareto‐Aware Radiotherapy Evolutionary Treatment Optimization), which simultaneously optimizes beam angles and fluence patterns by treating the PTV conformity and dose to OARs as separate objectives that are optimized by a powerful multi‐objective parallel genetic algorithm (GA).Methods: PARETO treats radiotherapytreatment planning as a single monolithic optimization problem, in which beam angle configurations and fluence patterns are explored to simultaneously optimize the PTV dose conformity and a dose objective for each OAR. We use a Pareto‐ranking scheme to discover necessary compromises between objectives. A final non‐dominated database of solutions is compiled from all plans evaluated during the run such that no solution in the database is superior to any other solution in all objectives. A graphical tool allows rapid navigation of the pre‐optimized database to select a final treatment option for the patient.Results: PARETO is at the stage of a working prototype. Solutions are of high quality, as judged by DVH curves and dose distribution, and consistent results are obtained between runs. Only minor differences in trade‐off surfaces result from four different fluence parameterizations of varying complexity, but they differ by a factor of ∼4 in speed. A novel feature allows optimization of the number of beams and a realistic test case shows that no further improvements in conformity are found for more than ∼9 beams. A newly implemented GPU‐based ray tracer, including convolution with a patient dose kernel, results in a speedup factor of ∼3 compared to CPU‐based computation. Conclusions: This work demonstrates PARETO's feasibility as a treatment‐planning tool, which replaces manual iterative optimization of treatment plans with a rapid graphical exploration of pre‐optimized solutions. Clinically acceptable run times of less than an hour appear within reach by combining several of our GPU‐based systems in parallel. J. Fiege discloses authorship of the Ferret GA (used by PARETO) and ownership of nQube Technical Computing Corporation, which distributes this optimization software.
更多查看译文
AI 理解论文
溯源树
样例
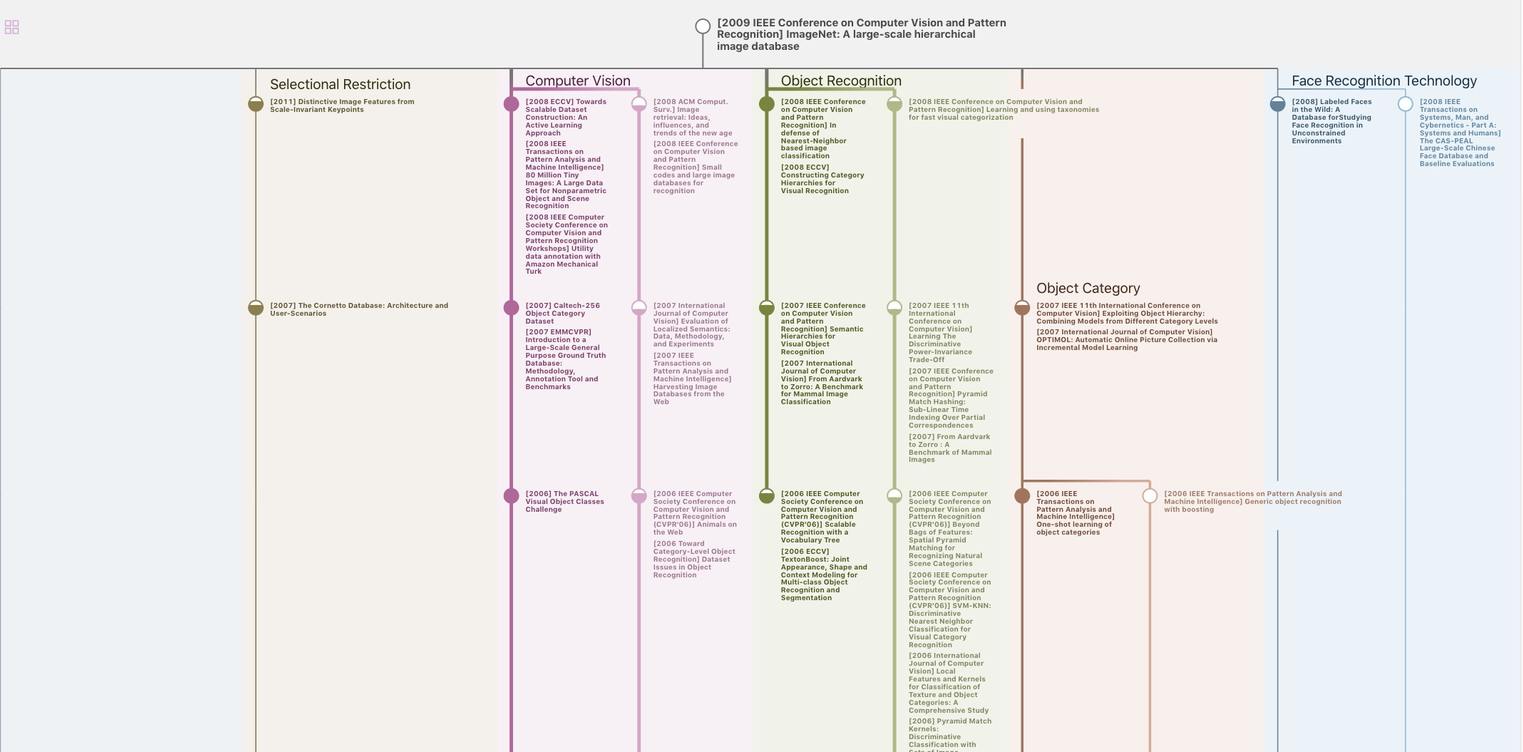
生成溯源树,研究论文发展脉络
Chat Paper
正在生成论文摘要