Unlearning of Mixed States in the Hopfield Model —Finite Loading Case—
JOURNAL OF THE PHYSICAL SOCIETY OF JAPAN(2015)
摘要
We study the unlearning of mixed states in the Hopfield model for the finite loading case, that is, alpha = p/N << 1, where N and p are the numbers of neurons and embedded patterns, respectively. In the general situation that any number of mixed states that exist in the model is unlearned, we derive the saddle point equations (SPEs) and evolution equations for overlaps by introducing sublattices. We postulate a condition that the solutions are stable in equilibrium, and prove that the static and dynamic stabilities are the same. We also prove that the stable state of the Hopfield model continuously changes and is statically and dynamically stable for sufficiently small unlearning coefficients. For p = 3, we perform detailed theoretical and numerical calculations. In the case that a single mixed state is unlearned, we determine phase boundaries using the Hessian matrix and by numerically integrating evolution equations. We performed Markov chain Monte Carlo simulations and find that the simulation results agree with the theoretical ones reasonably well. For general p, when all of the mixed states are unlearned with an equal unlearning coefficient eta, we derive the formulae for critical unlearning coefficients at the temperature T = 0 below which embedded patterns and mixed states exist and are stable as solutions of the SPEs. We found that there is an unlearning region of (T, eta) in which all patterns are retained and all mixed states are deleted, although tuning the parameters in this region is more difficult as p increases since the region shrinks. We numerically confirmed the theoretical results of the p dependences of the critical unlearning coefficients.
更多查看译文
AI 理解论文
溯源树
样例
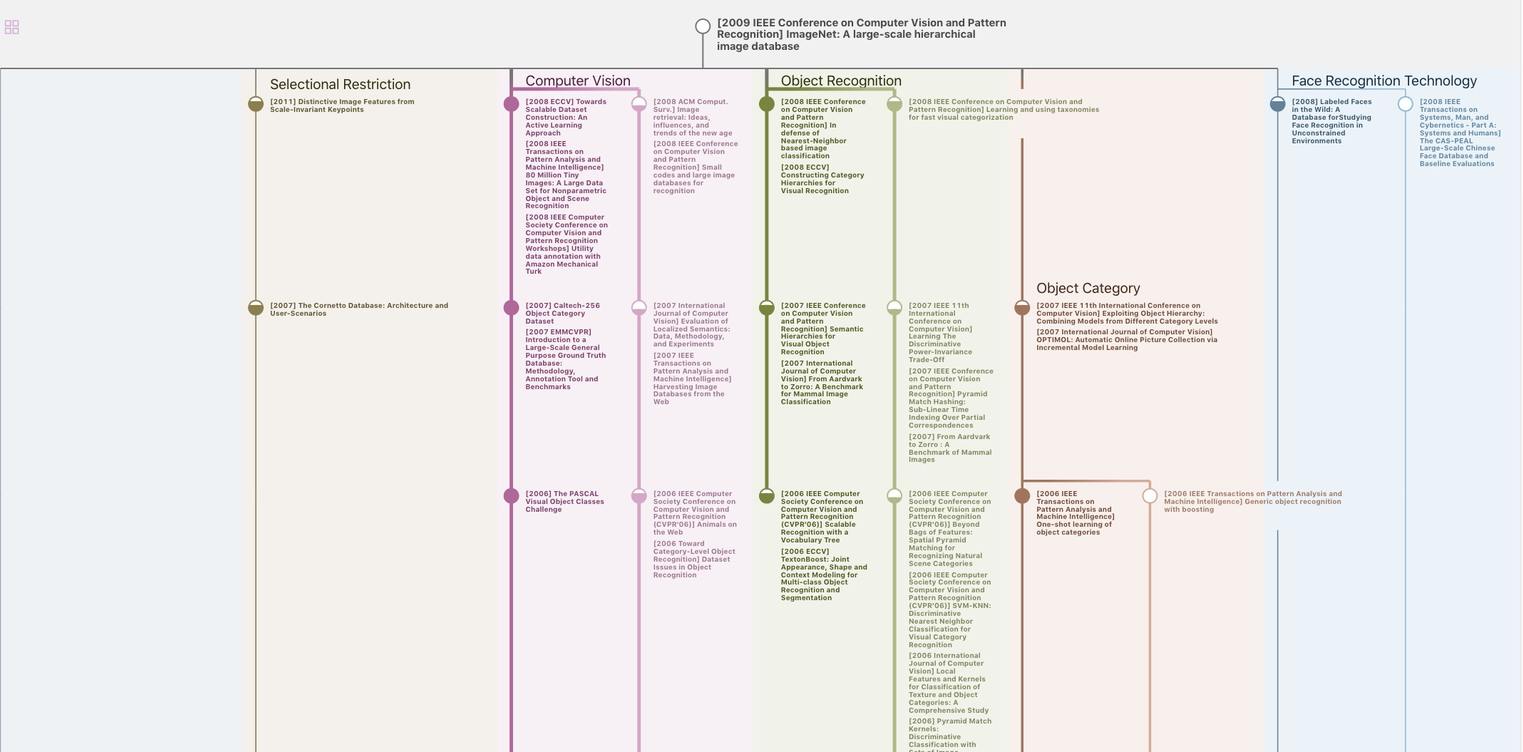
生成溯源树,研究论文发展脉络
Chat Paper
正在生成论文摘要