Teaching a Machine to Feel Postoperative Pain: Combining High‐Dimensional Clinical Data with Machine Learning Algorithms to Forecast Acute Postoperative Pain
PAIN MEDICINE(2015)
摘要
BackgroundGiven their ability to process highly dimensional datasets with hundreds of variables, machine learning algorithms may offer one solution to the vexing challenge of predicting postoperative pain. MethodsHere, we report on the application of machine learning algorithms to predict postoperative pain outcomes in a retrospective cohort of 8,071 surgical patients using 796 clinical variables. Five algorithms were compared in terms of their ability to forecast moderate to severe postoperative pain: Least Absolute Shrinkage and Selection Operator (LASSO), gradient-boosted decision tree, support vector machine, neural network, and k-nearest neighbor (k-NN), with logistic regression included for baseline comparison. ResultsIn forecasting moderate to severe postoperative pain for postoperative day (POD) 1, the LASSO algorithm, using all 796 variables, had the highest accuracy with an area under the receiver-operating curve (ROC) of 0.704. Next, the gradient-boosted decision tree had an ROC of 0.665 and the k-NN algorithm had an ROC of 0.643. For POD 3, the LASSO algorithm, using all variables, again had the highest accuracy, with an ROC of 0.727. Logistic regression had a lower ROC of 0.5 for predicting pain outcomes on POD 1 and 3. ConclusionsMachine learning algorithms, when combined with complex and heterogeneous data from electronic medical record systems, can forecast acute postoperative pain outcomes with accuracies similar to methods that rely only on variables specifically collected for pain outcome prediction.
更多查看译文
关键词
Machine Learning,Algorithm,Postoperative Pain,Pain Prediction
AI 理解论文
溯源树
样例
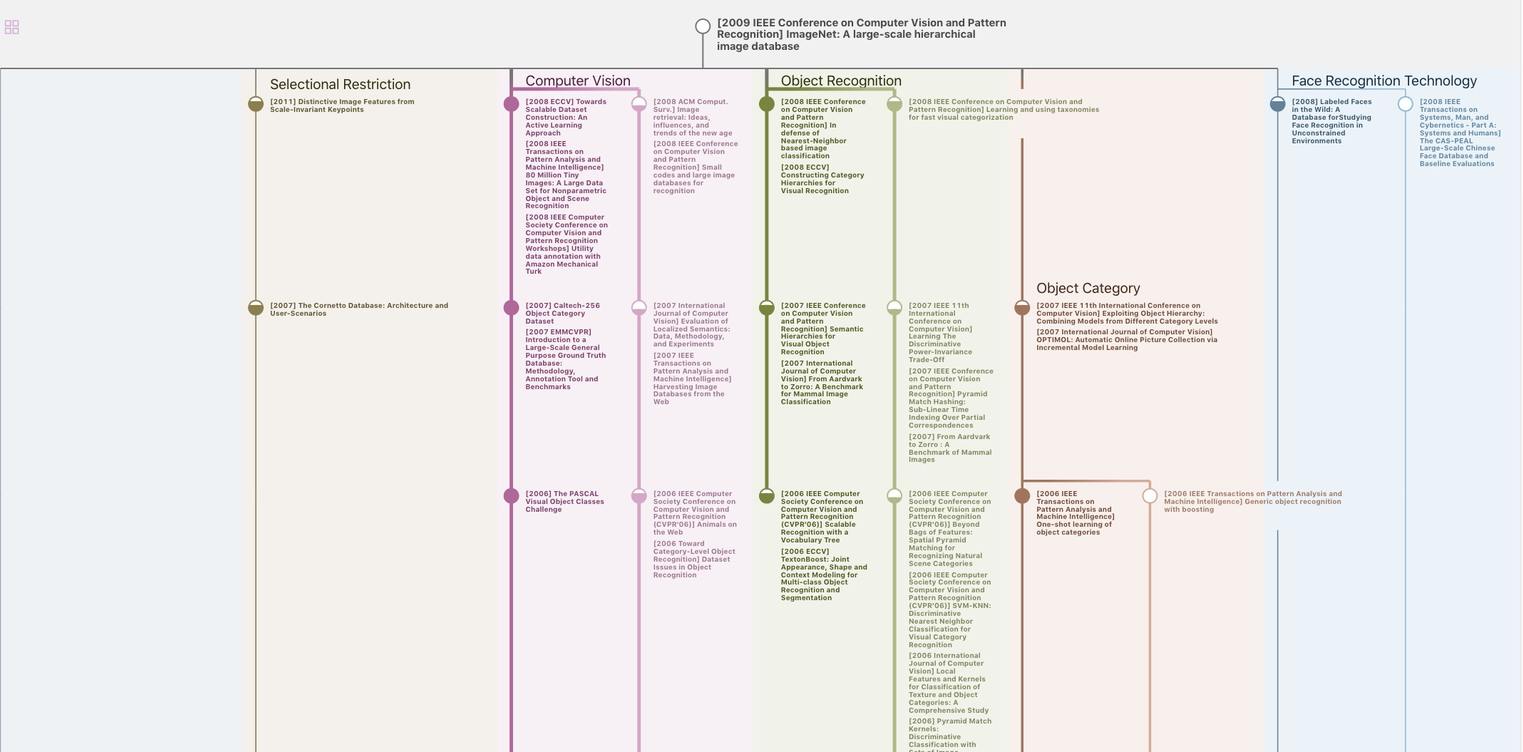
生成溯源树,研究论文发展脉络
Chat Paper
正在生成论文摘要