Hybrid support vector regression – Particle swarm optimization for prediction of compressive strength and RCPT of concretes containing metakaolin
Construction and Building Materials(2012)
摘要
This paper develops a hybrid support vector regression (SVR) - particle swarm optimization (PSO) model to predict the compressive strength (CS) and rapid chloride penetration test (RCPT) results of concretes containing metakaolin. The predictive accuracy of SVR models is highly dependent on their learning parameters. Therefore, PSO is exploited to seek the optimal hyper-parameters for SVR in order to improve its generalization capability. Moreover, a SVR-based sequential forward feature selection algorithm is proposed to disclose the most dominant input variables for the prediction of CS and RCPT results. The performance of the hybrid model is compared with the well-known system modeling method of adaptive neural-fuzzy inference system (ANFIS) by using 100 data samples with 25 different mix proportions established by experiments. The results show that the hybrid model has strong potential to predict material properties with high degree of accuracy and robustness. (C) 2012 Elsevier Ltd. All rights reserved.
更多查看译文
关键词
Support vector regression (SVR),Particle swarm optimization (PSO),Concrete,Metakaolin,Compressive strength,RCPT,Surface resistivity
AI 理解论文
溯源树
样例
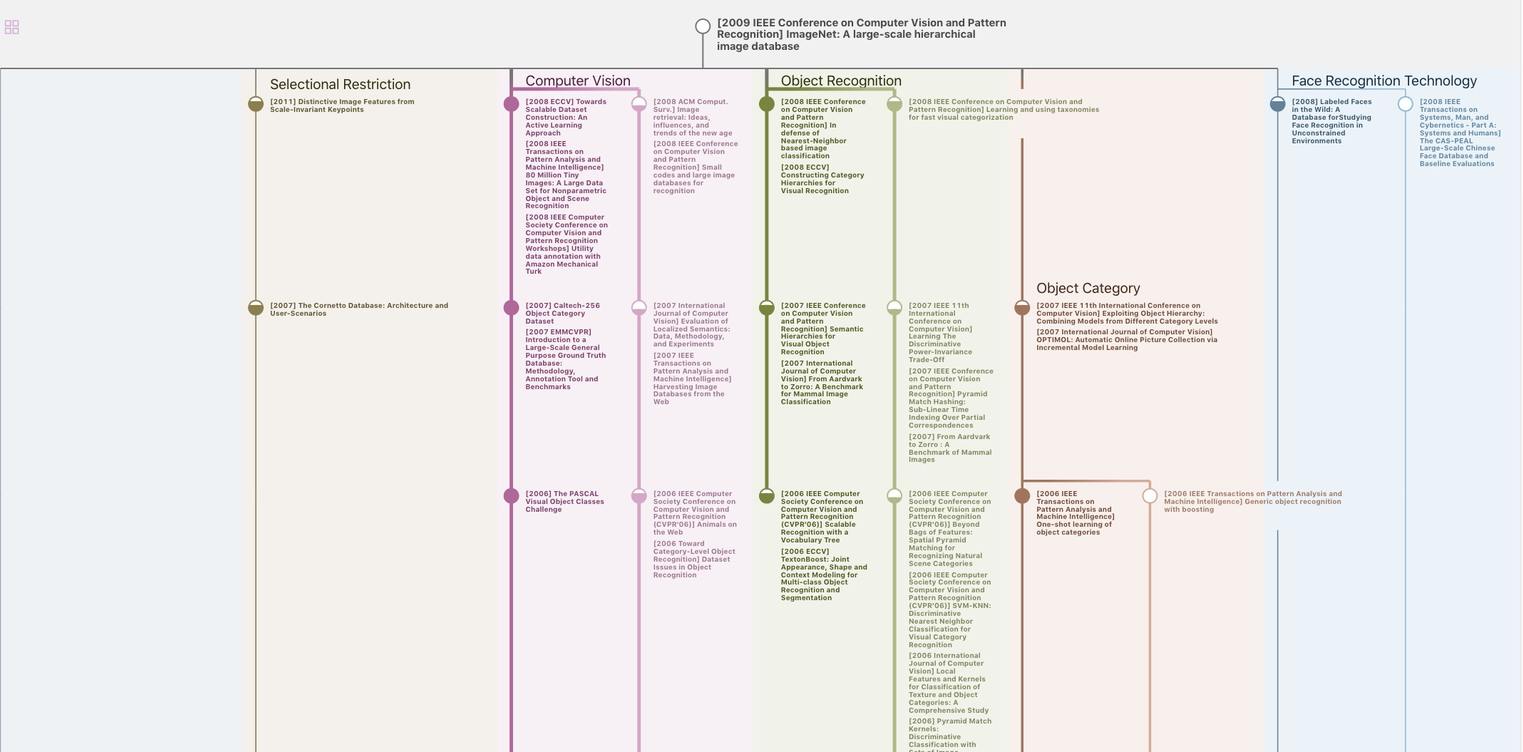
生成溯源树,研究论文发展脉络
Chat Paper
正在生成论文摘要