Collaborative filtering for massive multinomial data
JOURNAL OF APPLIED STATISTICS(2014)
摘要
Content recommendation on a webpage involves recommending content links (items) on multiple slots for each user visit to maximize some objective function, typically the click-through rate (CTR) which is the probability of clicking on an item for a given user visit. Most existing approaches to this problem assume user's response (click/no click) on different slots are independent of each other. This is problematic since in many scenarios CTR on a slot may depend on externalities like items recommended on other slots. Incorporating the effects of such externalities in the modeling process is important to better predictive accuracy. We therefore propose a hierarchical model that assumes a multinomial response for each visit to incorporate competition among slots and models complex interactions among (user, item, slot) combinations through factor models via a tensor approach. In addition, factors in our model are drawn with means that are based on regression functions of user/item covariates, which helps us obtain better estimates for users/items that are relatively new with little past activity. We show marked gains in predictive accuracy by various metrics.
更多查看译文
关键词
recommender systems,collaborative filtering,multinomial response,stochastic gradient descent,hierarchical modeling,tensor factorization
AI 理解论文
溯源树
样例
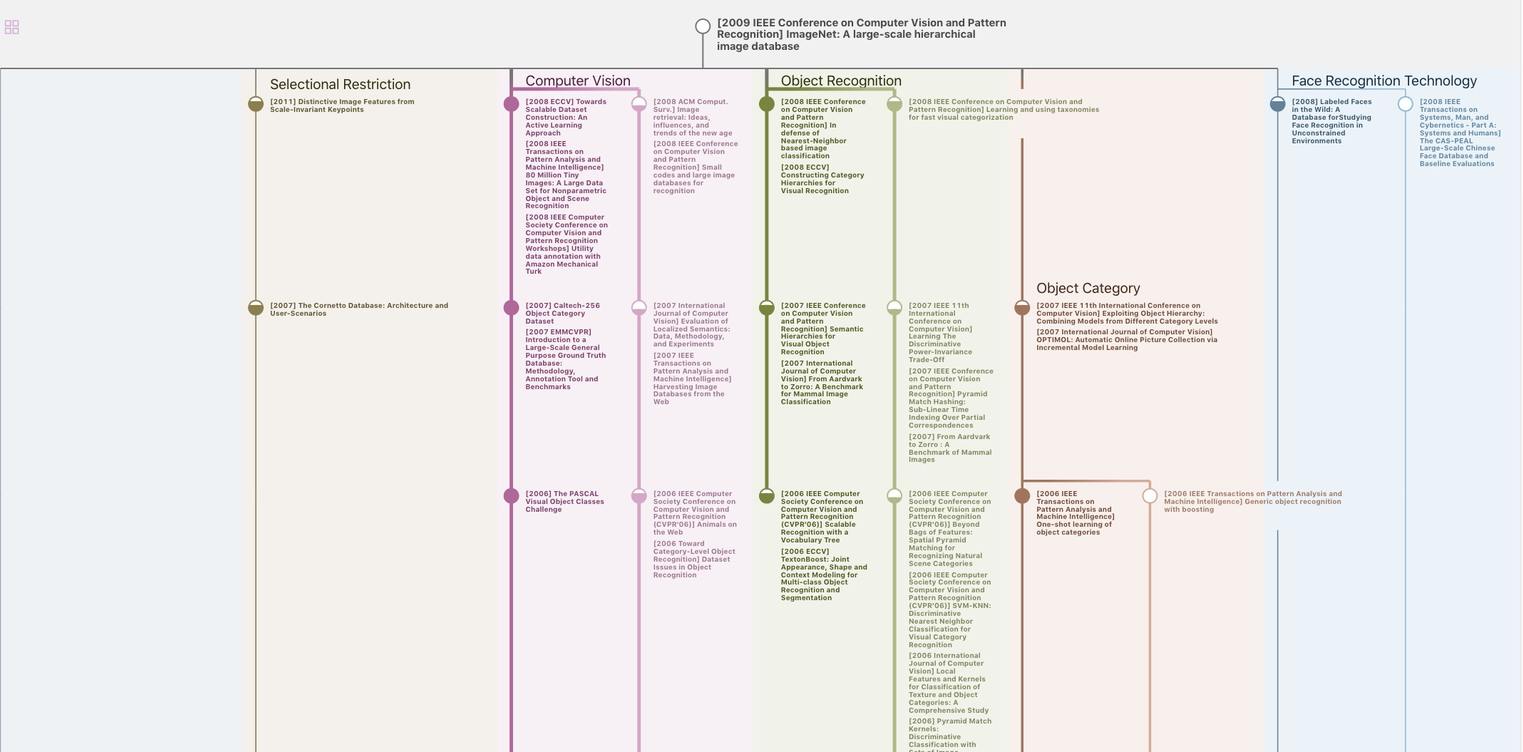
生成溯源树,研究论文发展脉络
Chat Paper
正在生成论文摘要