Computational modeling and prediction of the complex time-dependent phase behavior of lyotropic liquid crystals under in meso crystallization conditions
CRYSTAL GROWTH & DESIGN(2013)
摘要
Membrane-bound proteins comprise a very important class of drug targets. Solution of their structures by X-ray crystallography has been hampered by difficulties in crystallizing them in biologically relevant conformations. Novel amphiphilic materials that form bicontinuous cubic phases are being used to support the growth of crystals. However the cubic phase may transit to other lipidic mesophase structures under the influence of the different components within the crystallization screen. Furthermore the mesophases may evolve with time, a process that is poorly understood but potentially critical for controlled crystal growth. Recent advances in high-throughput screening of lipid systems have allowed us to generate a large body of data on the influence of screen components on the cubic phase. However it has been difficult to deconvolute individual effects in the multicomponent system present during a crystallization trial. We have therefore developed robust and predictive computational models that predict how the phase behavior of lyotropic liquid crystals changes over time and under the influence of crystallization additives. Our work demonstrates that the complex phase behavior of amphiphilic nanostructured nanoparticles can be captured with high accuracy using modern, robust machine learning methods. We predicted the existence of individual nanophases with accuracies of 98-99% and the complex coexistence of multiple phases to a similar accuracy using nonlinear models: linear models were not as effective and robust. This approach also allowed us to determine which crystallization screen components were most relevant to the temporal evolution of individual mesophases.
更多查看译文
关键词
high throughput screening,crystal growth,computational modeling,lyotropic liquid crystal,x ray crystallography,liquid crystals
AI 理解论文
溯源树
样例
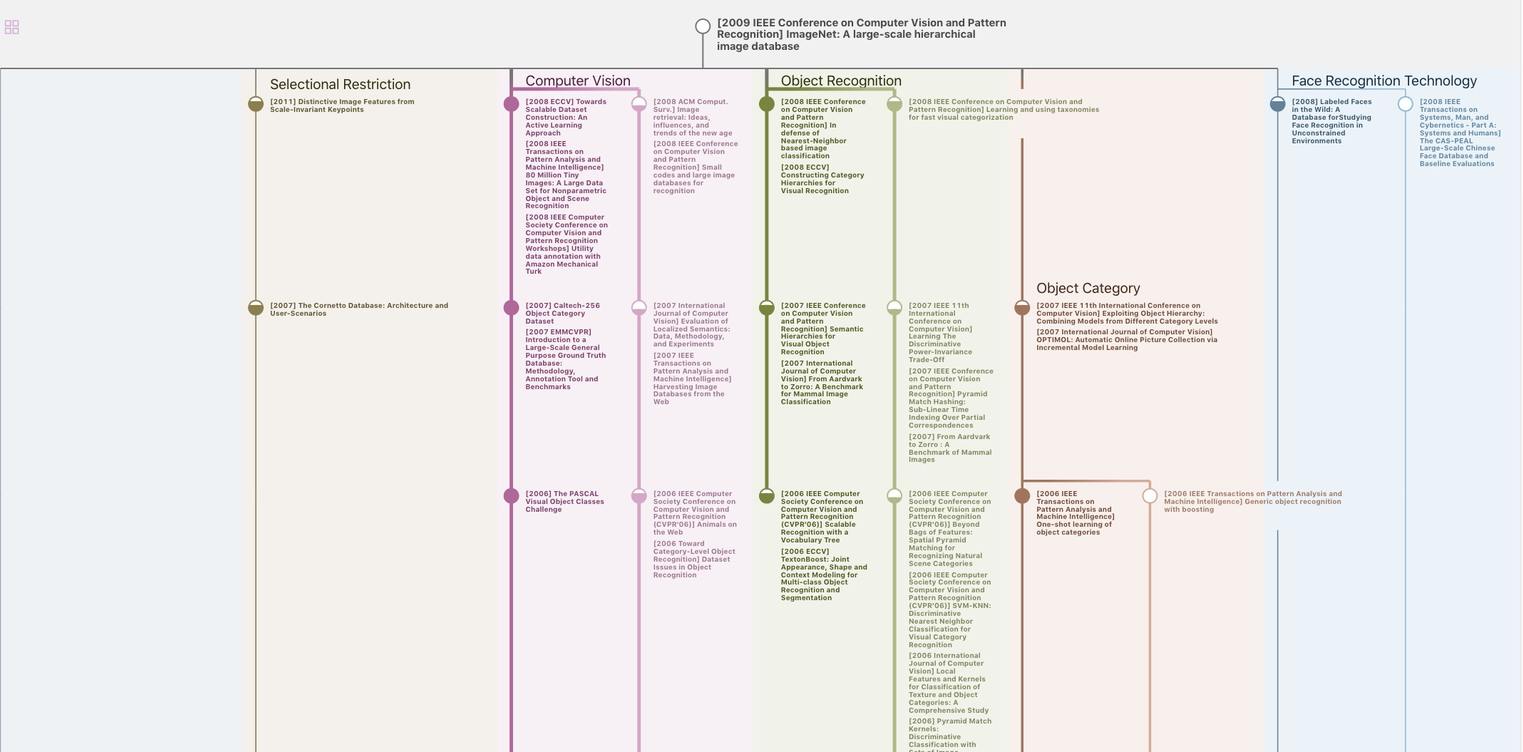
生成溯源树,研究论文发展脉络
Chat Paper
正在生成论文摘要