Optimizing spatial patterns with sparse filter bands for motor-imagery based brain–computer interface
Journal of Neuroscience Methods(2015)
摘要
Background: Common spatial pattern (CSP) has been most popularly applied to motor-imagery (MI) feature extraction for classification in brain-computer interface (BCI) application. Successful application of CSP depends on the filter band selection to a large degree. However, the most proper band is typically subject-specific and can hardly be determined manually. New method: This study proposes a sparse filter band common spatial pattern (SFBCSP) for optimizing the spatial patterns. SFBCSP estimates CSP features on multiple signals that are filtered from raw EEG data at a set of overlapping bands. The filter bands that result in significant CSP features are then selected in a supervised way by exploiting sparse regression. A support vector machine (SVM) is implemented on the selected features for MI classification. Results: Two public EEG datasets (BCI Competition III dataset IVa and BCI Competition IV lib) are used to validate the proposed SFBCSP method. Experimental results demonstrate that SFBCSP help improve the classification performance of MI. Comparison with existing methods: The optimized spatial patterns by SFBCSP give overall better MI classification accuracy in comparison with several competing methods. Conclusions: The proposed SFBCSP is a potential method for improving the performance of MI-based BCI. (C) 2015 Elsevier B.V. All rights reserved.
更多查看译文
关键词
Brain–computer interface (BCI),Common spatial pattern (CSP),Electroencephalogram (EEG),Motor imagery (MI),Sparse regression
AI 理解论文
溯源树
样例
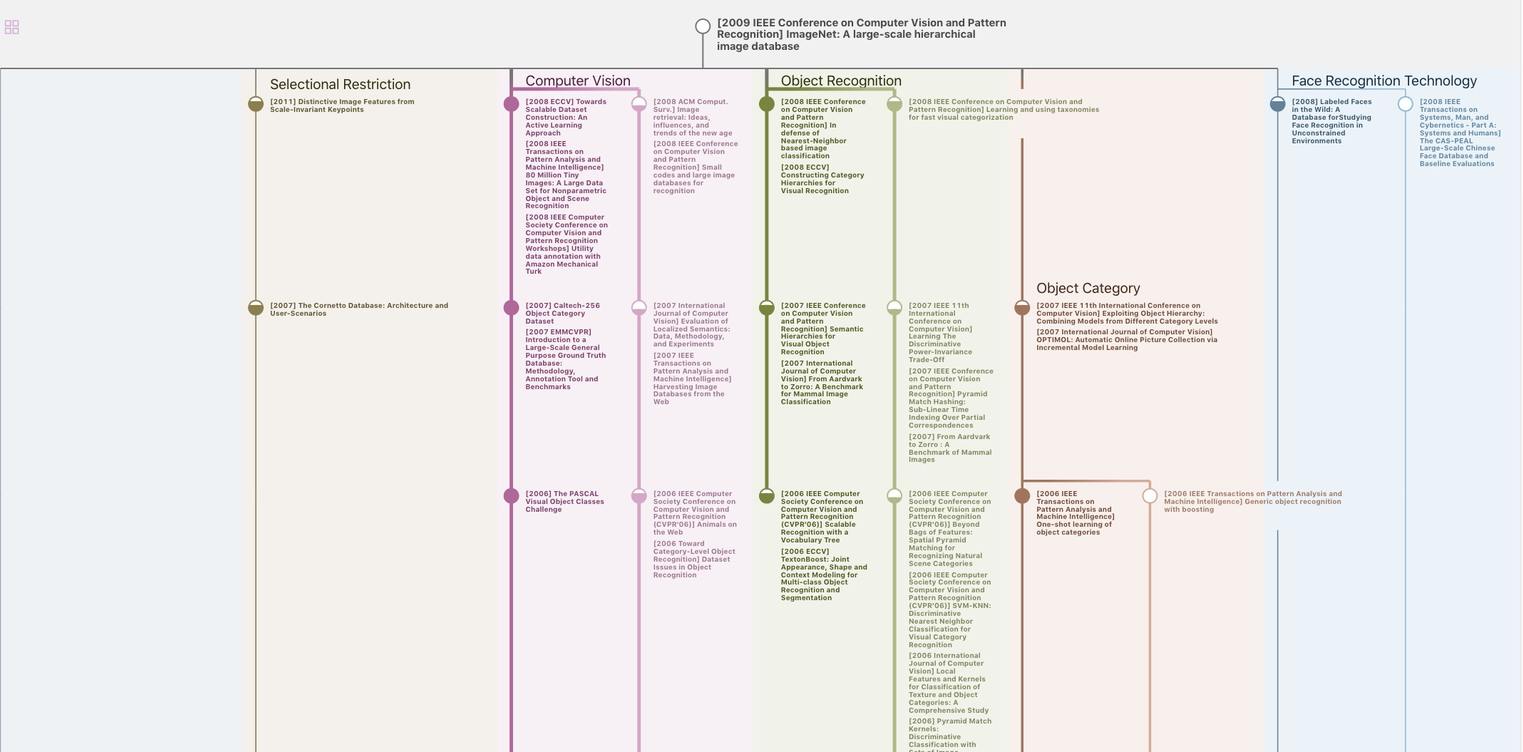
生成溯源树,研究论文发展脉络
Chat Paper
正在生成论文摘要