Bag of Naïve Bayes: biomarker selection and classification from genome-wide SNP data
BMC Bioinformatics(2012)
摘要
Background Multifactorial diseases arise from complex patterns of interaction between a set of genetic traits and the environment. To fully capture the genetic biomarkers that jointly explain the heritability component of a disease, thus, all SNPs from a genome-wide association study should be analyzed simultaneously. Results In this paper, we present Bag of Naïve Bayes (BoNB), an algorithm for genetic biomarker selection and subjects classification from the simultaneous analysis of genome-wide SNP data. BoNB is based on the Naïve Bayes classification framework, enriched by three main features: bootstrap aggregating of an ensemble of Naïve Bayes classifiers, a novel strategy for ranking and selecting the attributes used by each classifier in the ensemble and a permutation-based procedure for selecting significant biomarkers, based on their marginal utility in the classification process. BoNB is tested on the Wellcome Trust Case-Control study on Type 1 Diabetes and its performance is compared with the ones of both a standard Naïve Bayes algorithm and HyperLASSO, a penalized logistic regression algorithm from the state-of-the-art in simultaneous genome-wide data analysis. Conclusions The significantly higher classification accuracy obtained by BoNB, together with the significance of the biomarkers identified from the Type 1 Diabetes dataset, prove the effectiveness of BoNB as an algorithm for both classification and biomarker selection from genome-wide SNP data. Availability Source code of the BoNB algorithm is released under the GNU General Public Licence and is available at http://www.dei.unipd.it/~sambofra/bonb.html .
更多查看译文
关键词
Classification Performance,Marginal Utility,High Classification Performance,Total Computational Complexity,Ranking Step
AI 理解论文
溯源树
样例
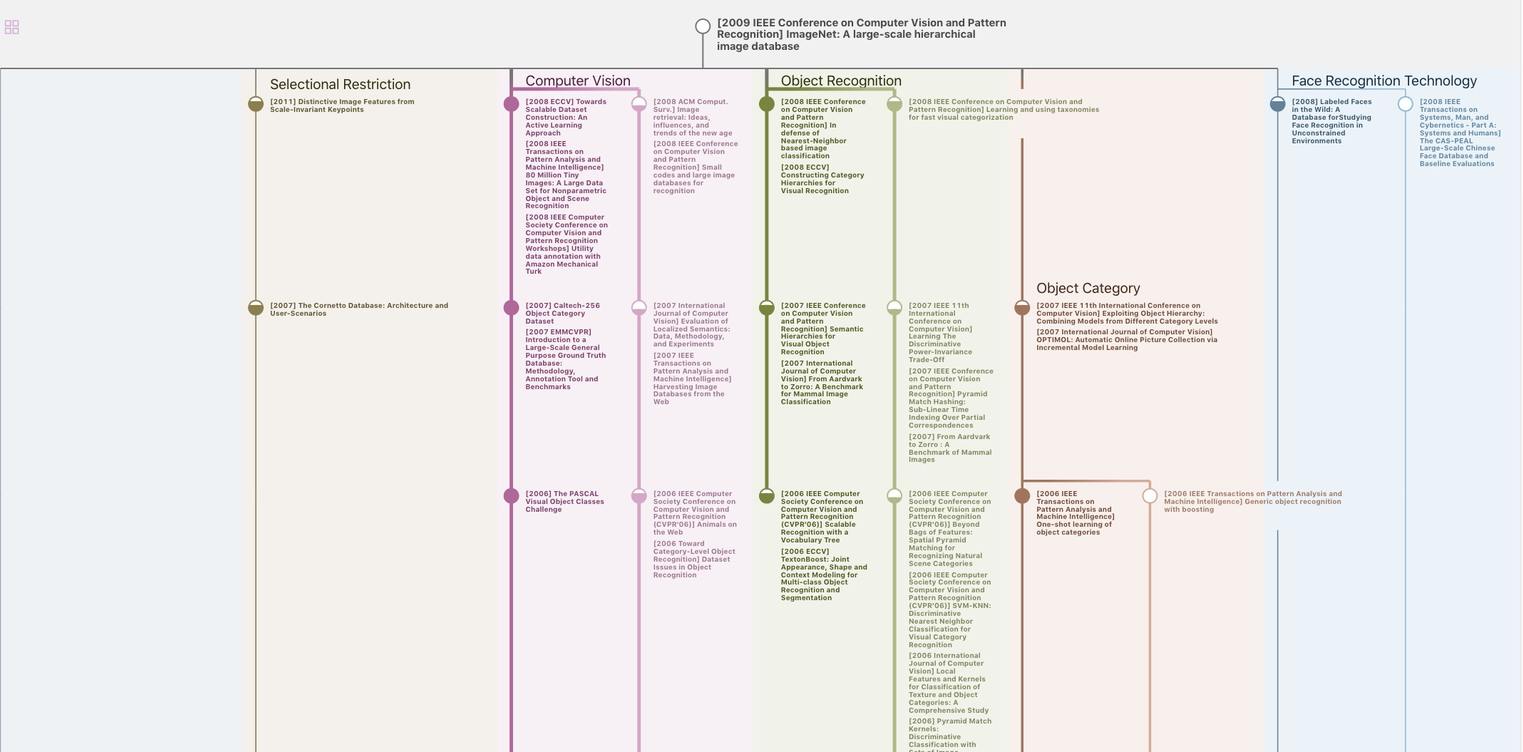
生成溯源树,研究论文发展脉络
Chat Paper
正在生成论文摘要