Hybrid cloud and error masking to improve the quality of deterministic satellite sea surface temperature retrieval and data coverage
Remote Sensing of Environment(2016)
摘要
In the infrared region, the quality of sea surface temperature (SST) retrievals critically depends on the cloud detection scheme. More than 5 million matchups, where the surface and top of atmosphere measurements are available, have been carefully analyzed to understand clouds related errors and to develop the advanced cloud detection scheme for improvement of satellite SST quality. The effectiveness of a Bayesian cloud detection (BCD) scheme, operationally implemented at the NOAA Office of Satellite Product Operations (OSPO) for the GOES-Imager, has been examined using an experimental filter and it is found that this scheme is not optimal. Thus, a new algorithm for cloud and error masking (CEM) scheme is proposed for physical SST retrievals. This is based on a quasi-deterministic approach combined with an approximated radiative transfer model and the functional spectral differences at pixel level. Although, traditionally the validation of cloud detection algorithms have often been reported qualitatively using visual inspection of imagery, we have made a quantitative validation of the cloud algorithm for its intended purpose by determining the quality of satellite SST retrievals against in situ data. Results show that CEM can reduce the root mean square error in SST by an average of 22% while increasing the data coverage by an average of 38% compared to the operationally implemented BCD at OSPO, as assessed over a period of fifty months.
更多查看译文
关键词
Cloud detection,Spectral differences method,Radiative transfer model,Bayesian cloud detection,Modified total least squares,Sea surface temperature,GOES-13 imager
AI 理解论文
溯源树
样例
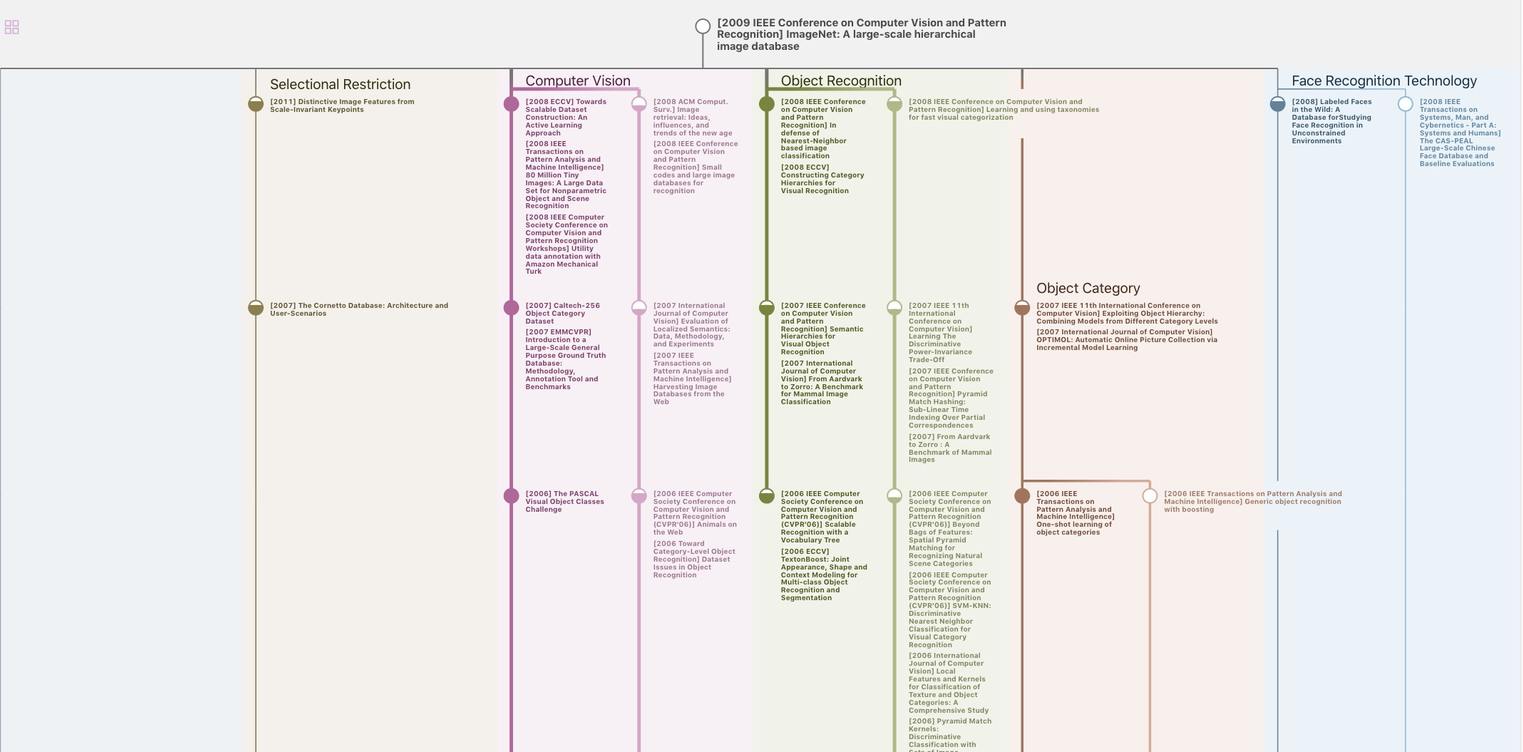
生成溯源树,研究论文发展脉络
Chat Paper
正在生成论文摘要