Distributed Gaussian Process Regression Under Localization Uncertainty
JOURNAL OF DYNAMIC SYSTEMS MEASUREMENT AND CONTROL-TRANSACTIONS OF THE ASME(2015)
摘要
In this paper, we propose distributed Gaussian process regression (GPR) for resource-constrained distributed sensor networks under localization uncertainty. The proposed distributed algorithm, which combines Jacobi over-relaxation (JOR) and discrete-time average consensus (DAC), can effectively handle localization uncertainty as well as limited communication and computation capabilities of distributed sensor networks. We also extend the proposed method hierarchically using sparse GPR to improve its scalability. The performance of the proposed method is verified in numerical simulations against the centralized maximum a posteriori (MAP) solution and a quick-and-dirty solution. We show that the proposed method outperforms the quick-and-dirty solution and achieve an accuracy comparable to the centralized solution.
更多查看译文
关键词
approximation,uncertainty,algorithms,sensor networks
AI 理解论文
溯源树
样例
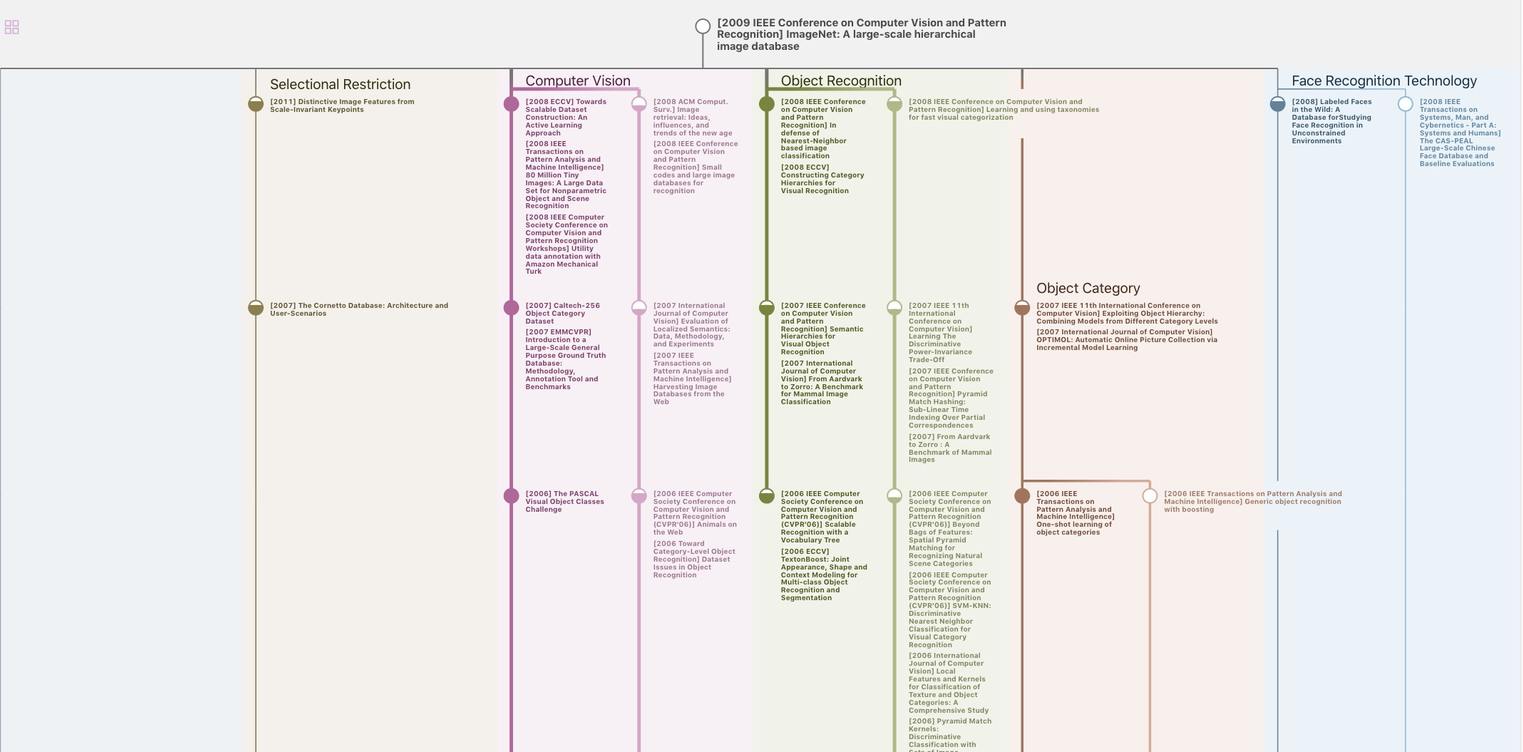
生成溯源树,研究论文发展脉络
Chat Paper
正在生成论文摘要