Renormalization group approach to 1D cellular automata with large updating neighborhoods
Complexity(2015)
摘要
We study self-similarity in one-dimensional probabilistic cellular automata PCA by applying a real-space renormalization technique to PCA with increasingly large updating neighborhoods. By studying the flow about the critical point of the renormalization, we may produce estimates of the spatial scaling properties of critical PCA. We find that agreement between our estimates and experimental values are improved by resolving correlations between larger blocks of spins, although this is not sufficient to converge to experimental values. However, applying the technique to PCA with larger neighborhoods, and, therefore, more renormalization parameters, results in further improvement. Our most refined estimate produces a spatial scaling exponent, found at the critical point of the five-neighbor PCA, of ﾿=1.056 which should be compared to the experimental value of ﾿=1.097. © 2014 Wiley Periodicals, Inc. Complexity 21: 206-213, 2015
更多查看译文
关键词
statistical mechanics,complex systems,renormalization,cellular automata
AI 理解论文
溯源树
样例
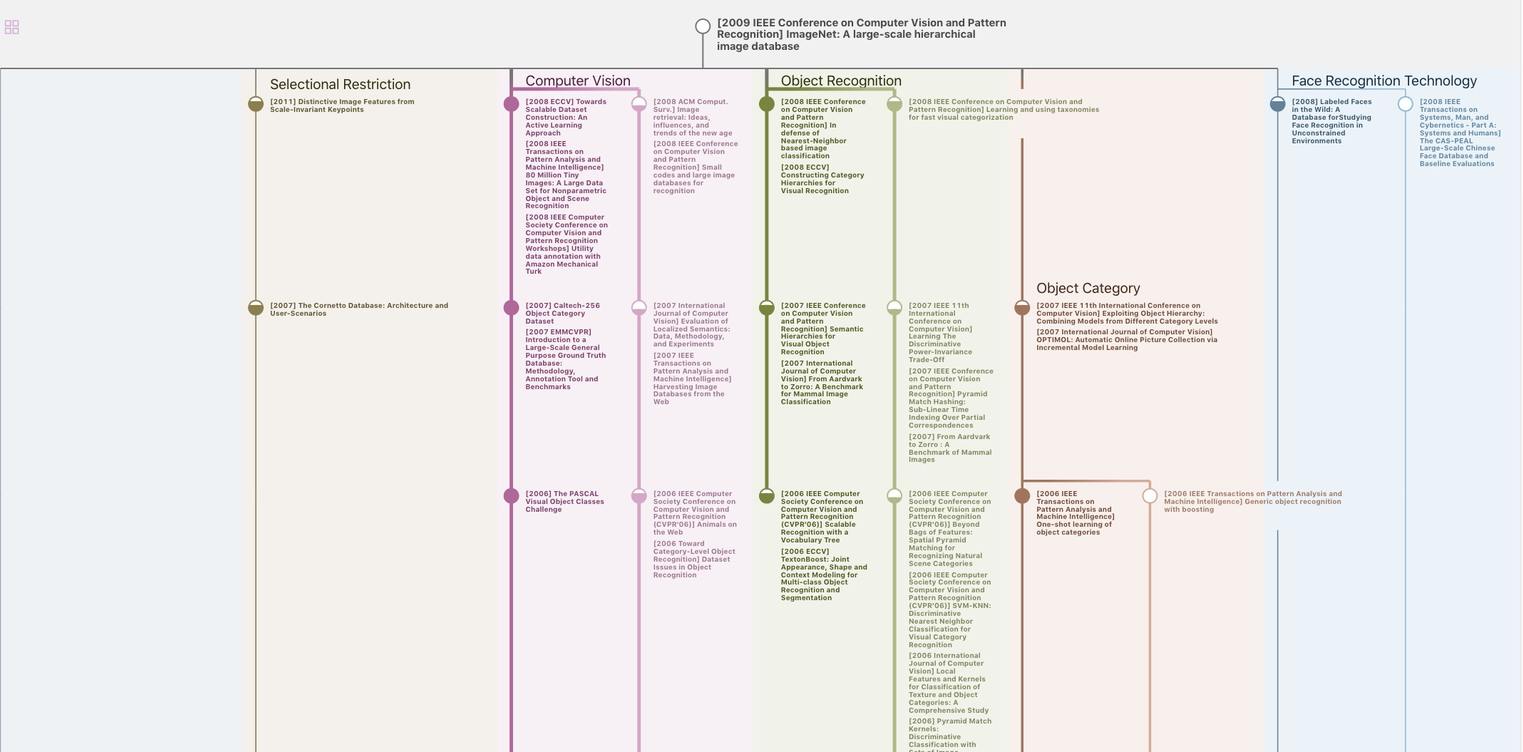
生成溯源树,研究论文发展脉络
Chat Paper
正在生成论文摘要