Topological Analysis of Variance and the Maxillary Complex
JOURNAL OF THE AMERICAN STATISTICAL ASSOCIATION(2012)
摘要
It is common to reduce the dimensionality of data before applying classical multivariate analysis techniques in statistics. Persistent homology, a recent development in computational topology, has been shown to be useful for analyzing high-dimensional (nonlinear) data. In this article, we connect computational topology with the traditional analysis of variance and demonstrate the value of combining these approaches on a three-dimensional orthodontic landmark dataset derived from the maxillary complex. Indeed, combining appropriate techniques of both persistent homology and analysis of variance results in a better understanding of the data's nonlinear features over and above what could have been achieved by classical means. Supplementary material for this article is available online.
更多查看译文
关键词
Barcodes,Betti numbers,Euclidean distance matrix analysis,Isometric feature mapping,Persistence diagrams,Persistent homology,Simplicial homology,Size-and-shape analysis,Wasserstein distance
AI 理解论文
溯源树
样例
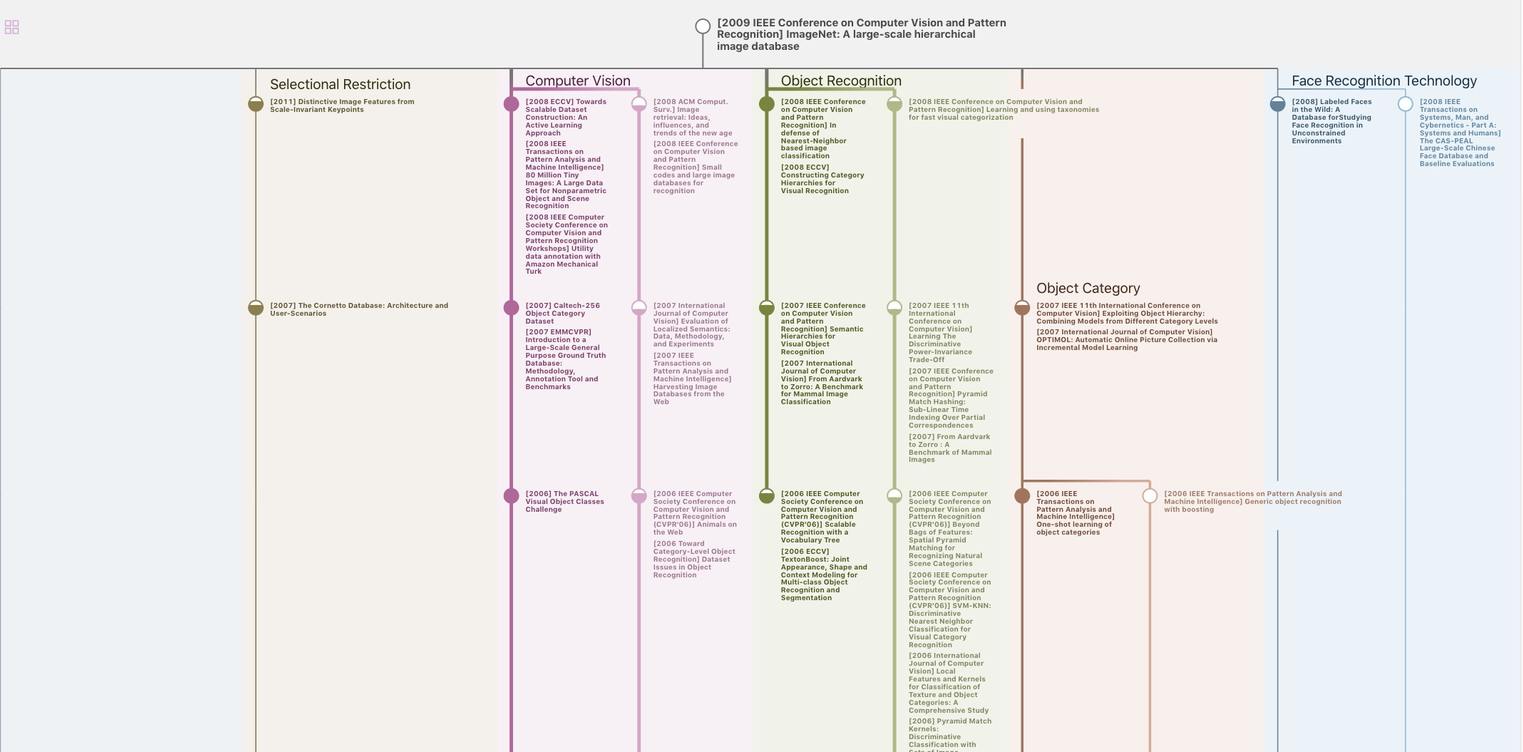
生成溯源树,研究论文发展脉络
Chat Paper
正在生成论文摘要