Land use regression models coupled with meteorology to model spatial and temporal variability of NO 2 and PM 10 in Changsha, China
Atmospheric Environment(2015)
摘要
Land use regression (LUR) models are widely used in epidemiological studies to assess exposure to air pollution. However, most of the existing LUR studies focus on estimating annual or monthly average concentration of air pollutants, with high spatial but low temporal resolution. In this paper, we combined LUR models with meteorological conditions to estimate daily nitrogen dioxide (NO2) and particulate matter (PM10) concentrations in the urban area of Changsha, China. Seventy-four sites for NO2 and thirty-six sites for PM10 were selected to build LUR models. The LUR models explained 51% and 62% of spatial variability for NO2 and PM10. The most important spatial explanatory variables included major roads, residential land and public facilities land, indicating that the spatial distributions of NO2 and PM10 are closely related to traffic conditions and human activities. Meteorological factors were introduced to model the temporal variability of NO2 and PM10 by using meteorological factors regression (MFR) and back propagation neural network (BPNN) modeling techniques. Important temporal explanatory variables included temperature, wind speed, cloud cover and percentage of haze. Pearson's r values between predicted and measured concentrations were much higher in BPNN models than in MFR models. The results demonstrate that the BPNN models showed a better performance than the MFR models in modeling temporal variation of NO2 and PM10. The approach of modeling spatial and temporal variation of air pollutants using LUR models coupled with meteorological conditions has potential usefulness for exposure assessment, especially for medium or short term exposure, in health studies.
更多查看译文
关键词
Land use regression,Temporal resolution,Meteorological factors,NO2,PM10
AI 理解论文
溯源树
样例
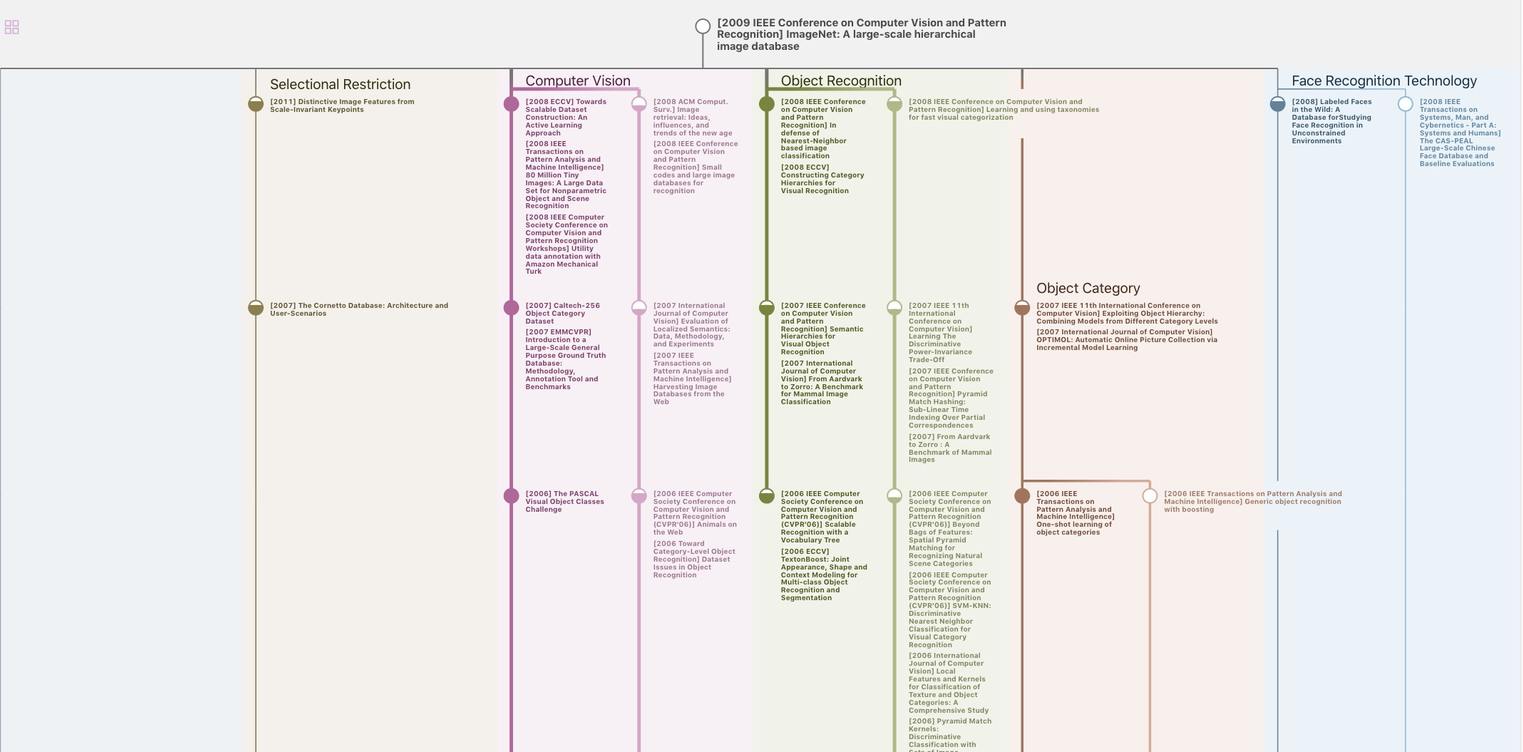
生成溯源树,研究论文发展脉络
Chat Paper
正在生成论文摘要