Supporting Shared Resource Usage For A Diverse User Community: The Osg Experience And Lessons Learned
INTERNATIONAL CONFERENCE ON COMPUTING IN HIGH ENERGY AND NUCLEAR PHYSICS 2012 (CHEP2012), PTS 1-6(2012)
摘要
The Open Science Grid (OSG) supports a diverse community of new and existing users in adopting and making effective use of the Distributed High Throughput Computing (DHTC) model. The LHC user community has deep local support within the experiments. For other smaller communities and individual users the OSG provides consulting and technical services through the User Support area. We describe these sometimes successful and sometimes not so successful experiences and analyze lessons learned that are helping us improve our services. The services offered include forums to enable shared learning and mutual support, tutorials and documentation for new technology, and troubleshooting of problematic or systemic failure modes. For new communities and users, we bootstrap their use of the distributed high throughput computing technologies and resources available on the OSG by following a phased approach. We first adapt the application and run a small production campaign on a subset of "friendly" sites. Only then do we move the user to run full production campaigns across the many remote sites on the OSG, adding to the community resources up to hundreds of thousands of CPU hours per day. This scaling up generates new challenges like no determinism in the time to job completion, and diverse errors due to the heterogeneity of the configurations and environments so some attention is needed to get good results. We cover recent experiences with image simulation for the Large Synoptic Survey Telescope (LSST), small-file large volume data movement for the Dark Energy Survey (DES), civil engineering simulation with the Network for Earthquake Engineering Simulation (NEES), and accelerator modeling with the Electron Ion Collider group at BNL. We will categorize and analyze the use cases and describe how our processes are evolving based on lessons learned.
更多查看译文
AI 理解论文
溯源树
样例
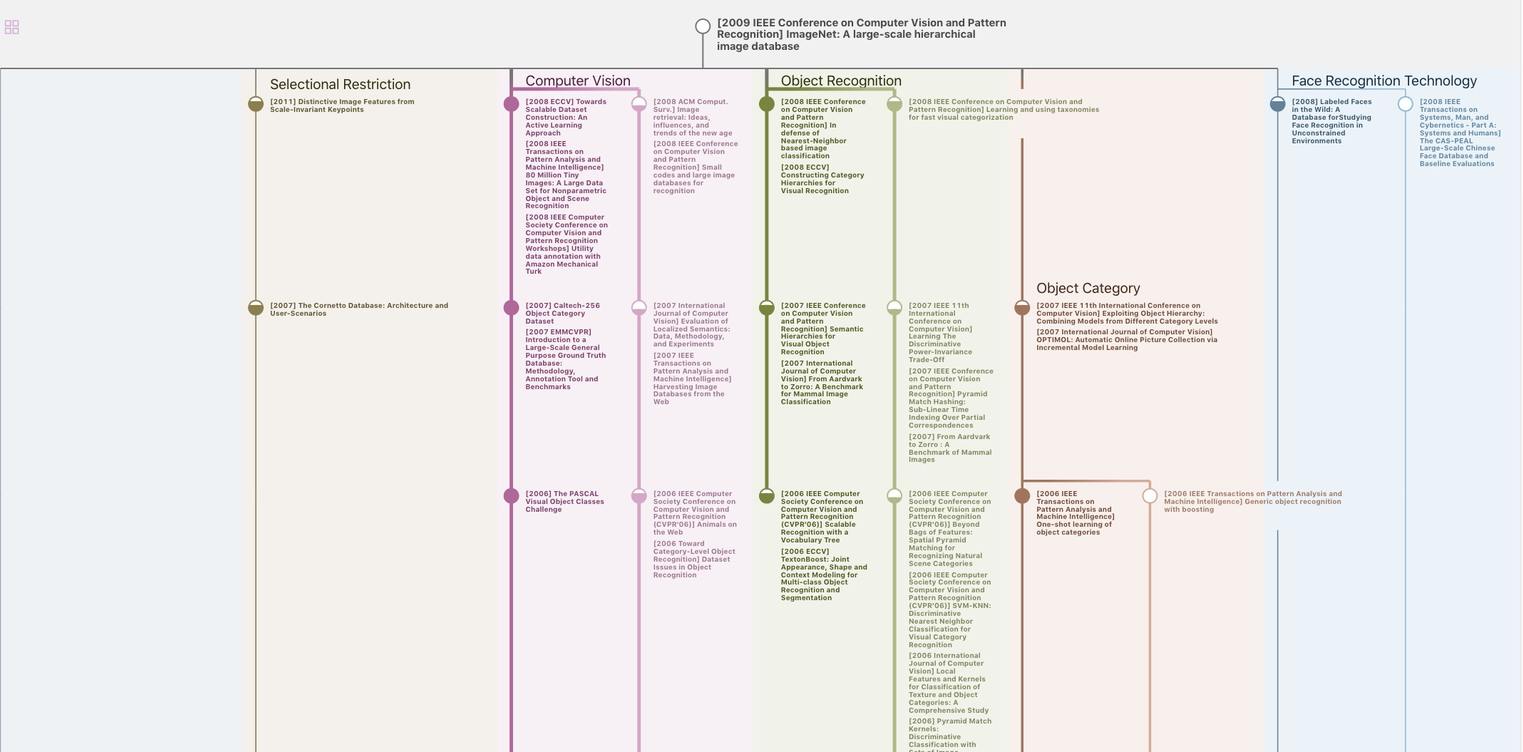
生成溯源树,研究论文发展脉络
Chat Paper
正在生成论文摘要