Split Bregman iteration solution for sparse optimization in image restoration
Optik(2014)
摘要
It is always a challenging task to develop effective and accurate models for robust image restoration. In this paper, the family of sparse and redundant representation frameworks is considered as an alternative for the above problem. The principle of the family is expatiated on the development and research progress. Two well-known denoising methods are presented and analyzed on their properties. The K-SVD algorithm is an effective method for sparse representation. The iteratively approximate algorithms are always used for the solution of sparse coding operations. Here, a convexification of the l0 norm to the l1 norm is adopted in the implementation of K-SVD method. Then a split Bregman iteration solution is proposed for l1 regularization problems in the performance of the sparse representation of the K-SVD algorithm. The split Bregman iterative method is well studied and fused into the famous K-SVD method. The PSNR (Peak Signal to Noise Ratio) and MSSIM (Mean Structural Similarity) are used to evaluate the performance of those methods. Experimental results on different types of images indicate that our proposed method not only achieve comparable results with the state of art methods, but also make the original method more efficient. Besides, it also provides a valuable and promising reference for image restoration techniques.
更多查看译文
关键词
Image restoration,Sparse and redundant representation,Split Bregman iteration,Dictionary learning
AI 理解论文
溯源树
样例
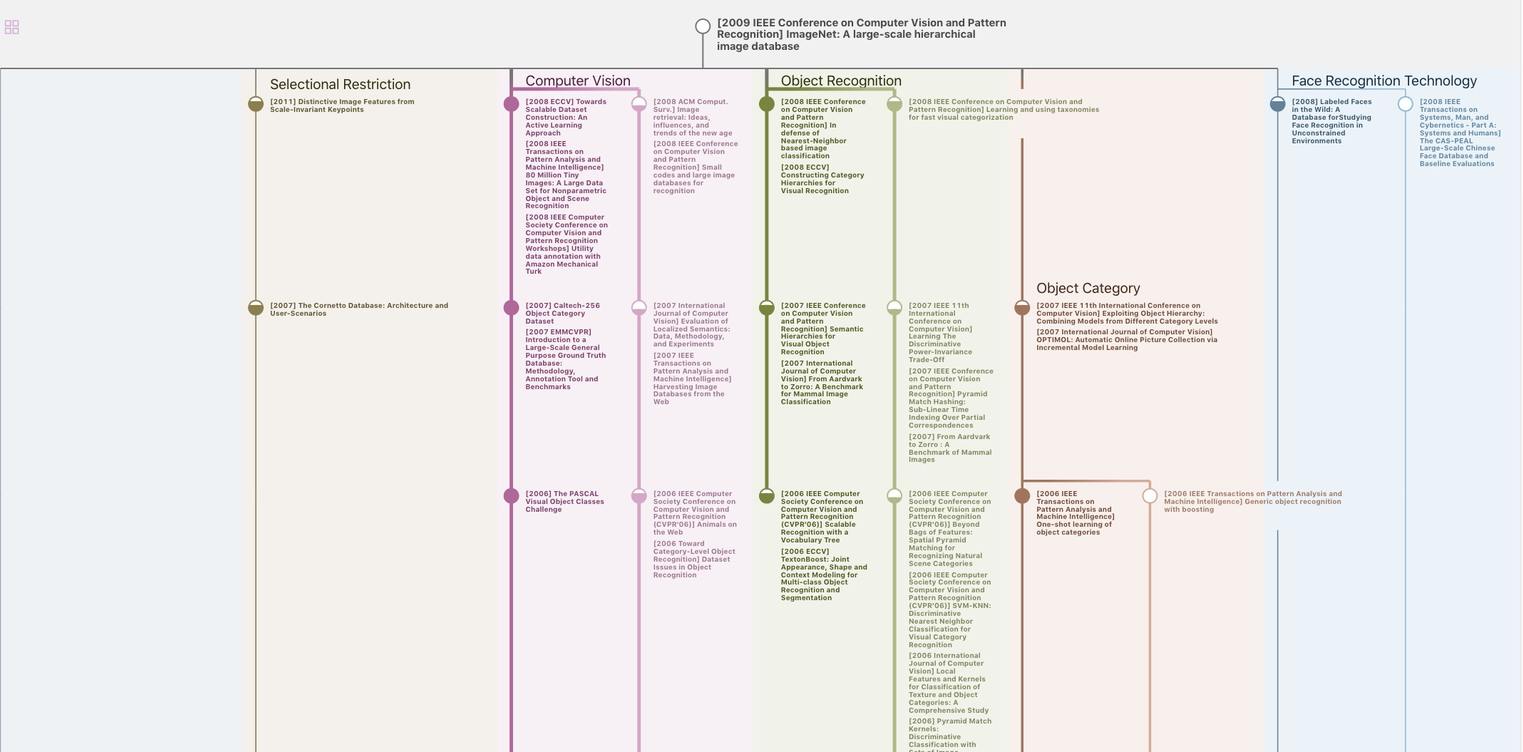
生成溯源树,研究论文发展脉络
Chat Paper
正在生成论文摘要