UPLC–MS retention time prediction: a machine learning approach to metabolite identification in untargeted profiling
Metabolomics(2015)
摘要
Metabolic profiling focuses on the analysis of a wide range of small endogenous molecules in order to understand the response of a living system to perturbations. Ultra high performance liquid chromatography–mass spectrometry is a widely employed profiling tool, but its application is limited by difficulties in identification of detected metabolites. Herein, we demonstrate how the prediction of retention time can help resolve this major issue. We describe a general approach that enables the generation of reliable quantitative structure retention relationship models tailored to specific chromatographic protocols. This methodology, applied to 442 experimentally characterised standards, employs a combination of random forest and support vector regression models with molecular interaction descriptors. In this unusual application, the Volsurf + molecular descriptors demonstrated a high ability to describe chromatographic retention. On external validation sets, and for a wide range of chemical classes, predicted values were in average within 13 % of the experimentally observed retention time. More importantly, the presented procedure reduced by more than 80 % the number of false putative identification, greatly improving metabolite identification. Furthermore, in 95 % of cases, the correct identification was promoted within the top three metabolite suggestions. This retention time prediction framework can be replicated by different laboratories to suit their profiling platforms and enhance the value of standard library by providing a new tool for compound identification.
更多查看译文
关键词
UPLC–MS, Retention time prediction, Support vector regression, Random forest, Self-organizing maps
AI 理解论文
溯源树
样例
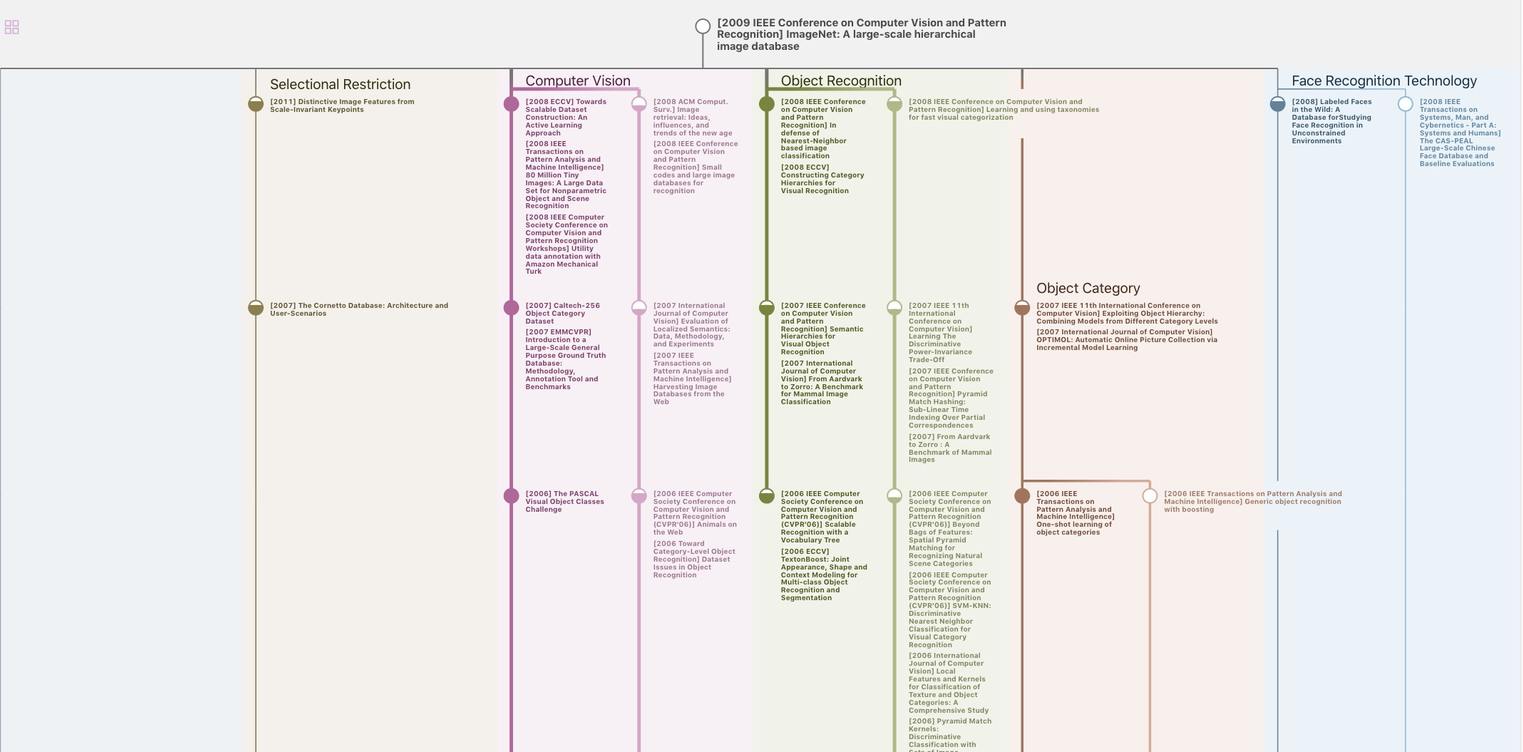
生成溯源树,研究论文发展脉络
Chat Paper
正在生成论文摘要