Controlling the quality of metabolomics data: new strategies to get the best out of the QC sample
Metabolomics(2014)
摘要
The type and use of quality control (QC) samples is a ‘hot topic’ in metabolomics. QCs are not novel in analytical chemistry; however since the evolution of using QCs to control the quality of data in large scale metabolomics studies (first described in 2011), the need for detailed knowledge of how to use QCs and the effects they can have on data treatment is growing. A controlled experiment has been designed to illustrate the most advantageous uses of QCs in metabolomics experiments. For this, samples were formed from a pool of plasma whereby different metabolites were spiked into two groups in order to simulate biological biomarkers. Three different QCs were compared: QCs pooled from all samples, QCs pooled from each experimental group of samples separately and QCs provided by an external source (QC surrogate). On the experimentation of different data treatment strategies, it was revealed that QCs collected separately for groups offers the closest matrix to the samples and improves the statistical outcome, especially for biomarkers unique to one group. A novel quality assurance plus procedure has also been proposed that builds on previously published methods and has the ability to improve statistical results for QC pool. For this dataset, the best option to work with QC surrogate was to filter data based only on group presence. Finally, a novel use of recursive analysis is portrayed that allows the improvement of statistical analyses with respect to the ratio between true and false positives.
更多查看译文
关键词
Quality control samples, Quality assurance procedure, False positives, Recursive analysis, In silico, QC surrogate
AI 理解论文
溯源树
样例
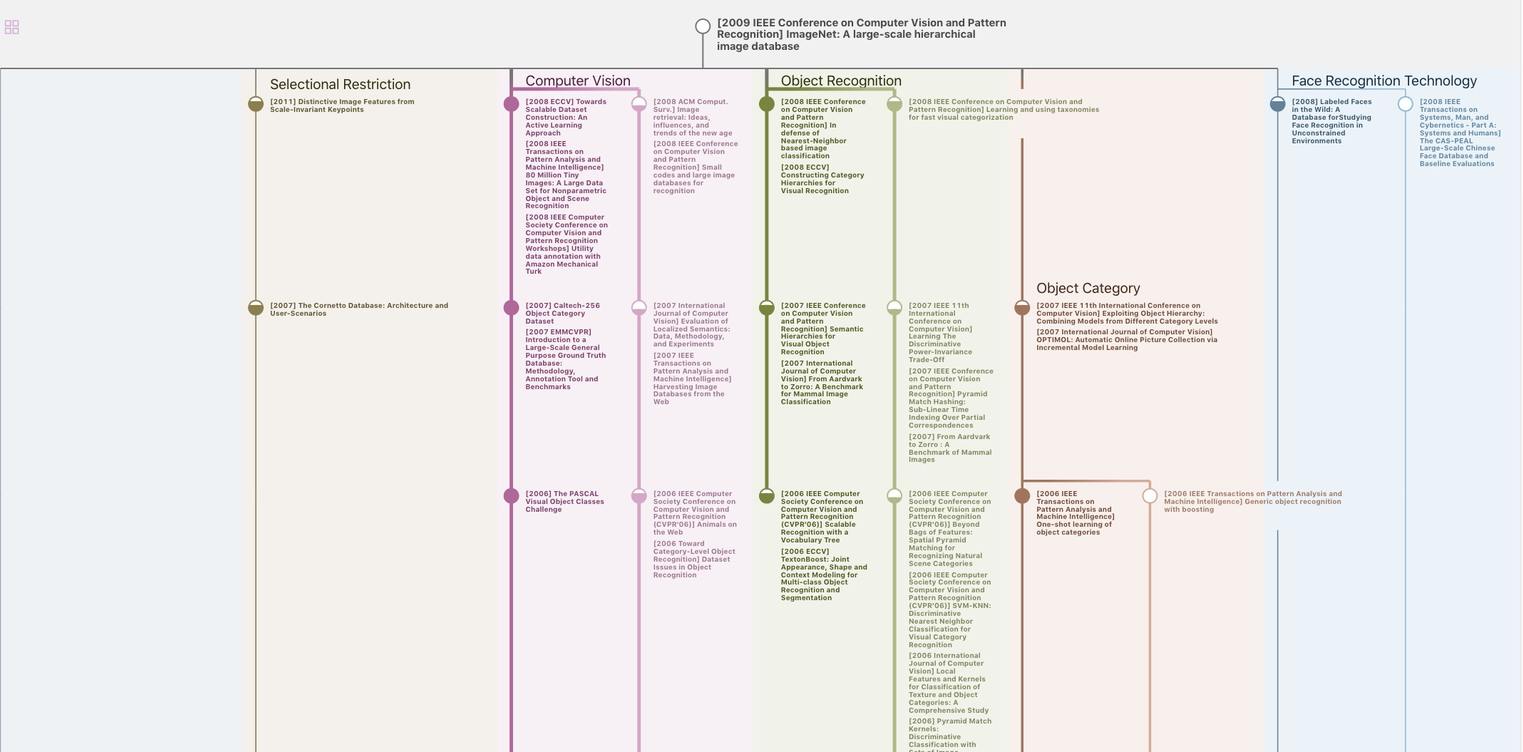
生成溯源树,研究论文发展脉络
Chat Paper
正在生成论文摘要