A Novel Multi-Feature Descriptor for Human Detection Using Cascaded Classifiers in Static Images
Journal of Signal Processing Systems(2014)
摘要
Combining multiple kinds of features is useful to achieve the state of the art performance for human detection. But combining more features will result in high dimensional feature descriptors, which is time-consuming for feature extraction and detection. How to exploit different kinds of features and reduce the dimension of feature descriptor are challenging problems. A novel multi-feature descriptor (MFD) combining Optimal Histograms of Oriented Gradients (OHOG), Local Binary Patterns (LBP) and Color Self-Similarity in Neighbor (NCSS) is proposed. Firstly, a discriminative feature selection and combination strategy is introduced to obtain distinctive local HOGs and construct OHOG feature. OHOG combines local discriminative and correlated information, which improves the classification performance compared with HOG. Besides, LBP describes texture feature of human appearance. Finally, a compact and lower dimensional feature NCSS is proposed to encode the self-similarity of color histograms in limited neighbor sub-regions instead of global regions. The proposed MFD describes human appearance from gradient, texture and color features, which can complement each other and improve the robustness of human description. To further improve detection speed without decreasing accuracy, we cascade early stages of Adaboost based on selected local HOGs and SVM classifier based on MFD. The former part can reject most non-human detection windows quickly and the final SVM classifier can guarantee a high accuracy. Experimental results on public dataset show that the proposed MFD and cascaded classifiers framework can achieve promising results both in accuracy and detection speed.
更多查看译文
关键词
Human detection,Feature extraction,HOG,Multi-feature,Cascaded classifiers
AI 理解论文
溯源树
样例
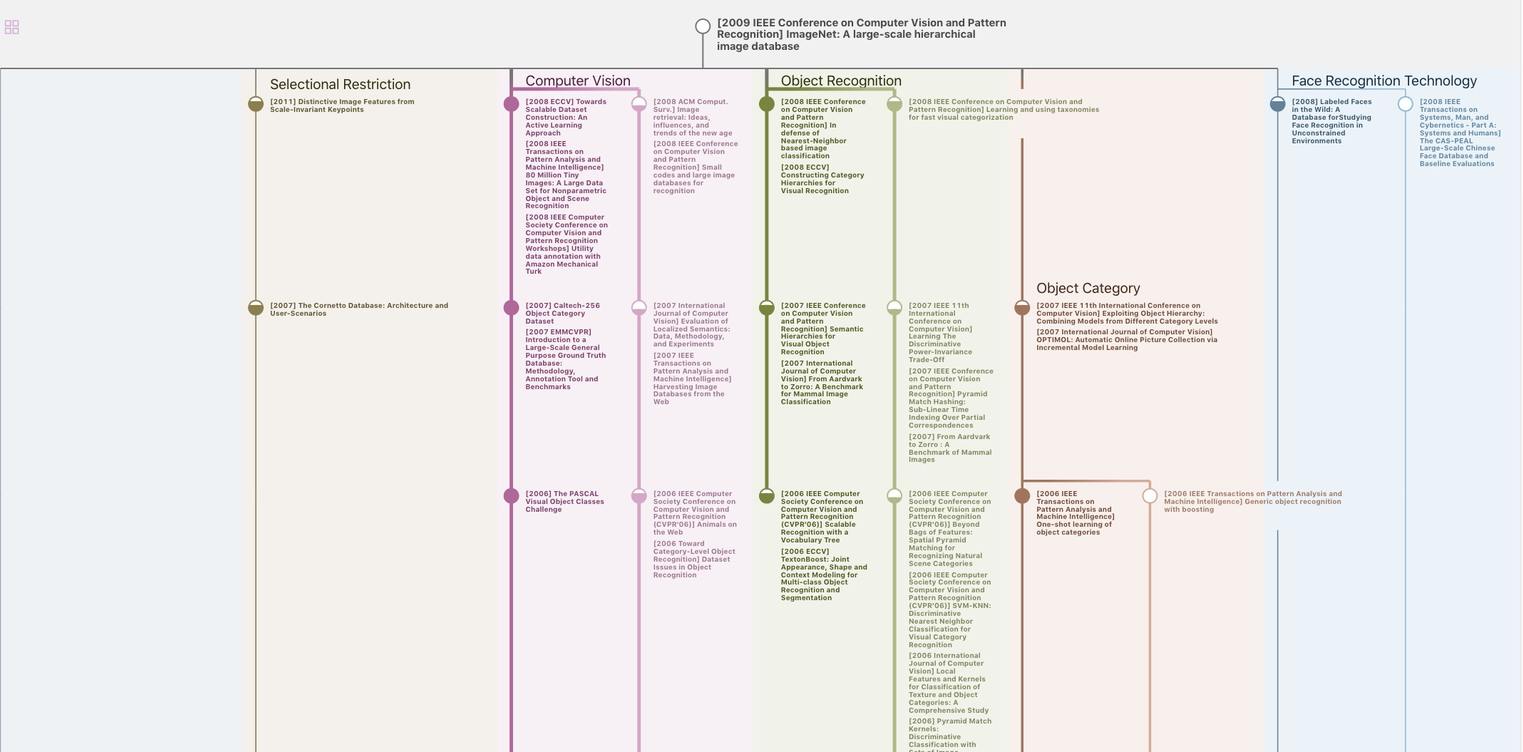
生成溯源树,研究论文发展脉络
Chat Paper
正在生成论文摘要