Interactive lesion segmentation on dynamic contrast enhanced breast MRI using a Markov model
Proceedings of SPIE(2006)
摘要
The purpose of this study is to develop a method for segmenting lesions on Dynamic Contrast-Enhanced (DCE) breast MRI. DCE breast MRI, in which the breast is imaged before, during, and after the administration of a contrast agent, enables a truly 3D examination of breast tissues. This functional angiogenic imaging technique provides noninvasive assessment of microcirculatory characteristics of tissues in addition to traditional anatomical structure information. Since morphological features and kinetic curves from segmented lesions are to be used for diagnosis and treatment decisions, lesion segmentation is a key pre-processing step for classification. In our study, the ROI is defined by a bounding box containing the enhancement region in the subtraction image, which is generated by subtracting the precontrast image from 1(st) post-contrast image. A maximum a posteriori (MAP) estimate of the class membership (lesion vs. non-lesion) for each voxel is obtained using the Iterative Conditional Mode (ICM) method. The prior distribution of the class membership is modeled as a multi-level logistic model, a Markov Random Field model in which the class membership of each voxel is assumed to depend upon its nearest neighbors only. The likelihood distribution is assumed to be Gaussian. The parameters of each Gaussian distribution are estimated from a dozen voxels manually selected as representative of the class. The experimental segmentation results demonstrate anatomically plausible breast tissue segmentation and the predicted class membership of voxels from the interactive segmentation algorithm agrees with the manual classifications made by inspection of the kinetic enhancement curves. The proposed method is advantageous in that it is efficient, flexible, and robust.
更多查看译文
关键词
Dynamic Contrast-Enhanced breast MRI,lesion segmentation,kinetic curve,morphological feature,region of interest (ROI),subtraction image,Markov Random Field,MAP estimate
AI 理解论文
溯源树
样例
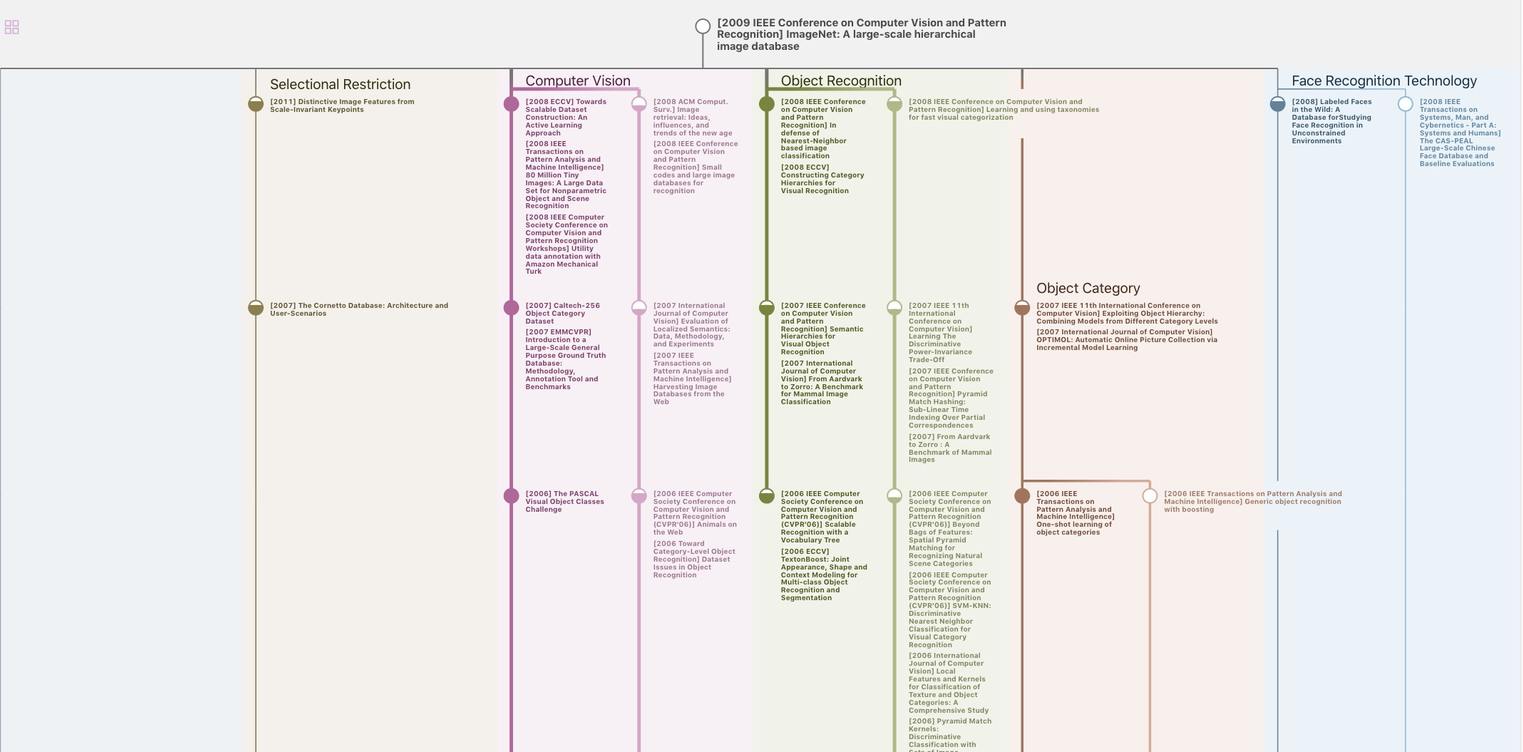
生成溯源树,研究论文发展脉络
Chat Paper
正在生成论文摘要