An Improved Hidden Markov Model Classifier For Sar Images
SIGNAL PROCESSING, SENSOR FUSION, AND TARGET RECOGNITION VIII(1999)
摘要
The study reported in this paper represents a continuation of our previous work on the application of Hidden Markov models (HMMs) to the translational and rotational invariant classification of SAR targets*. The traditional method of making classification decisions using an HMM does not achieve the desired objective of minimizing the number of misclassifications. We present a novel technique that minimizes the probability of misclassification error. This approach, which is an adaptation of an existing Minimum Classification Error (MCE) strategy, is globally optimal. The proposed method applies basic principles of pattern recognition to reduce the expected misclassification rate by dynamically perturbing the HMM parameters using a constraint on a cross-entropy measure and distance separation between pairs of HMM models. Like the traditional implementation of an HMM, our new formulation can still be implemented using an efficient forward-backward algorithm for estimating the HMM parameters. We tested our classifier on a public mixed-target MSTAR database and compared our approach to the original HMM approach trained by using a maximum likelihood criterion. The results indicate a significant improvement over the original HMM approach. Current scores from our method are about in excess of 90% on testing data sets.
更多查看译文
关键词
hidden Markov model, minimum classification error, synthetic aperture radar, cross-entropy, misclassification
AI 理解论文
溯源树
样例
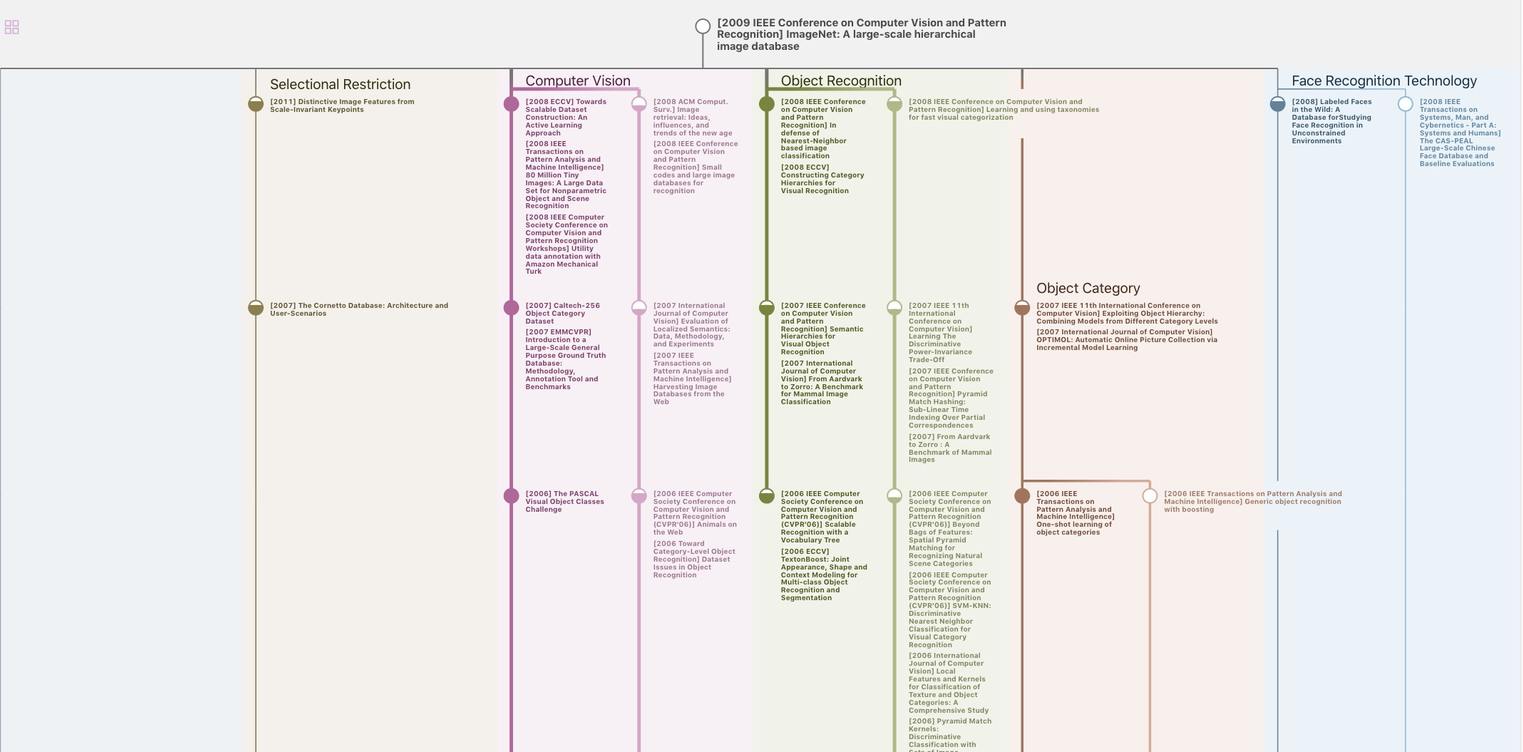
生成溯源树,研究论文发展脉络
Chat Paper
正在生成论文摘要