Progressively Consolidating Historical Visual Explorations For New Discoveries
VISUALIZATION AND DATA ANALYSIS 2014(2014)
摘要
A significant task within data mining is to identify data models of interest. While facilitating the exploration tasks, most visualization systems do not make use of all the data models that are generated during the exploration. In this paper, we introduce a system that allows the user to gain insights from the data space progressively by forming data models and consolidating the generated models on the fly. Each model can be a a computationally extracted or user-defined subset that contains a certain degree of interest and might lead to some discoveries. When the user generates more and more data models, the degree of interest of some portion of some models will either grow (indicating higher occurrence) or will fluctuate or decrease (corresponding to lower occurrence). Our system maintains a collection of such models and accumulates the interestingness of each model into a consolidated model. In order to consolidate the models, the system summarizes the associations between the models in the collection and identifies support (models reinforce each other), complementary (models complement each other), and overlap of the models. The accumulated interestingness keeps track of historical exploration and helps the user summarize their findings which can lead to new discoveries. This mechanism for integrating results from multiple models can be applied to a wide range of decision support systems. We demonstrate our system in a case study involving the financial status of US companies.
更多查看译文
关键词
multiple models, model consolidation
AI 理解论文
溯源树
样例
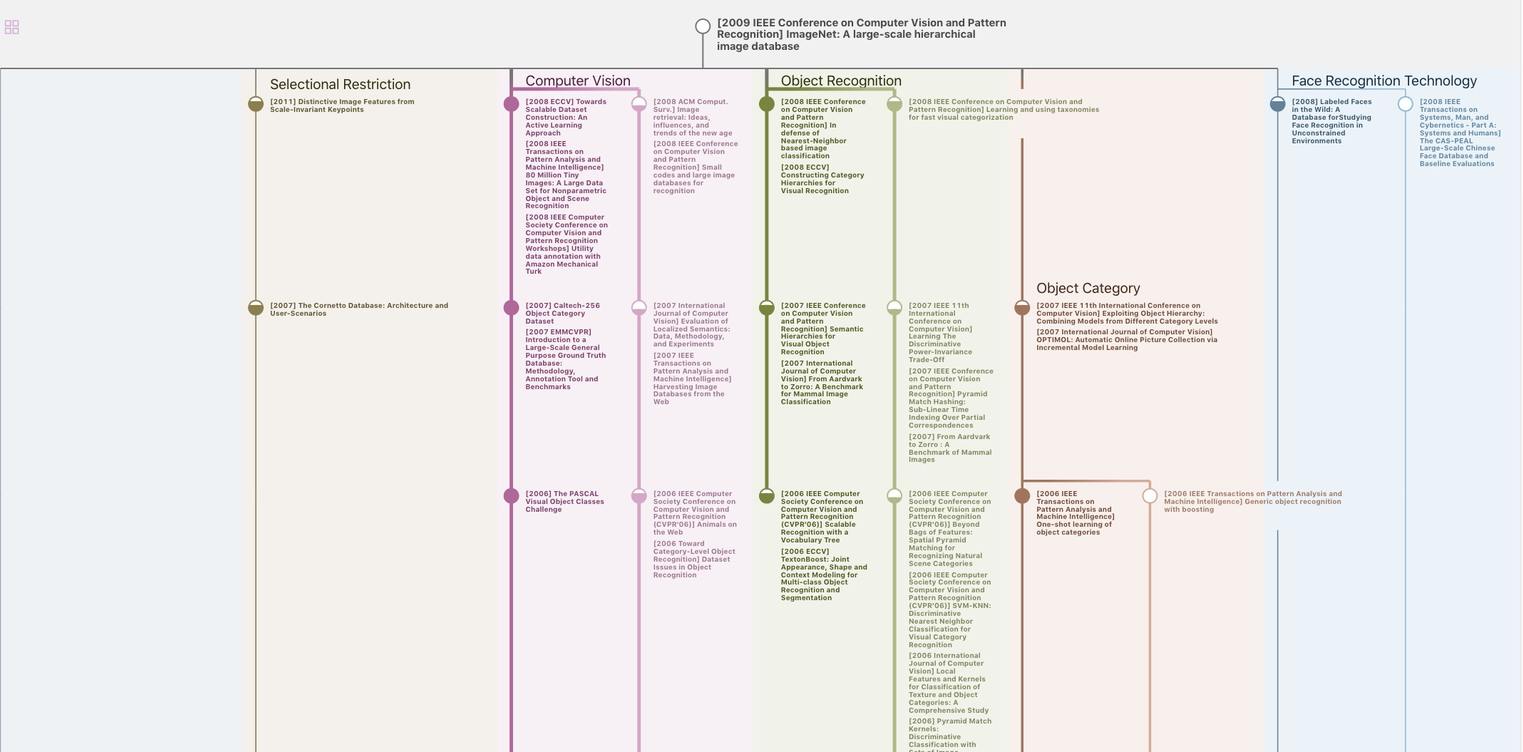
生成溯源树,研究论文发展脉络
Chat Paper
正在生成论文摘要