Four Color Theorem And Convex Relaxation For Image Segmentation With Any Number Of Regions
INVERSE PROBLEMS AND IMAGING(2013)
摘要
Image segmentation is an essential problem in imaging science. One of the most successful segmentation models is the piecewise constant Mumford-Shah minimization model. This minimization problem is however difficult to carry out, mainly due to the non-convexity of the energy. Recent advances based on convex relaxation methods are capable of estimating almost perfectly the geometry of the regions to be segmented when the mean intensity and the number of segmented regions are known a priori. The next important challenge is to provide a tight approximation of the optimal geometry, mean intensity and the number of regions simultaneously while keeping the computational time and memory usage reasonable. In this work, we propose a new algorithm that combines convex relaxation methods with the four color theorem to deal with the unsupervised segmentation problem. More precisely, the proposed algorithm can segment any a priori unknown number of regions with only four intensity functions and four indicator ("labeling") functions. The number of regions in our segmentation model is decided by one parameter that controls the regularization strength of the geometry, i.e., the total length of the boundary of all the regions. The segmented image function can take as many constant values as needed.
更多查看译文
关键词
Unsupervised segmentation, Mumford-Shah model, four color theorem, convex relaxation method
AI 理解论文
溯源树
样例
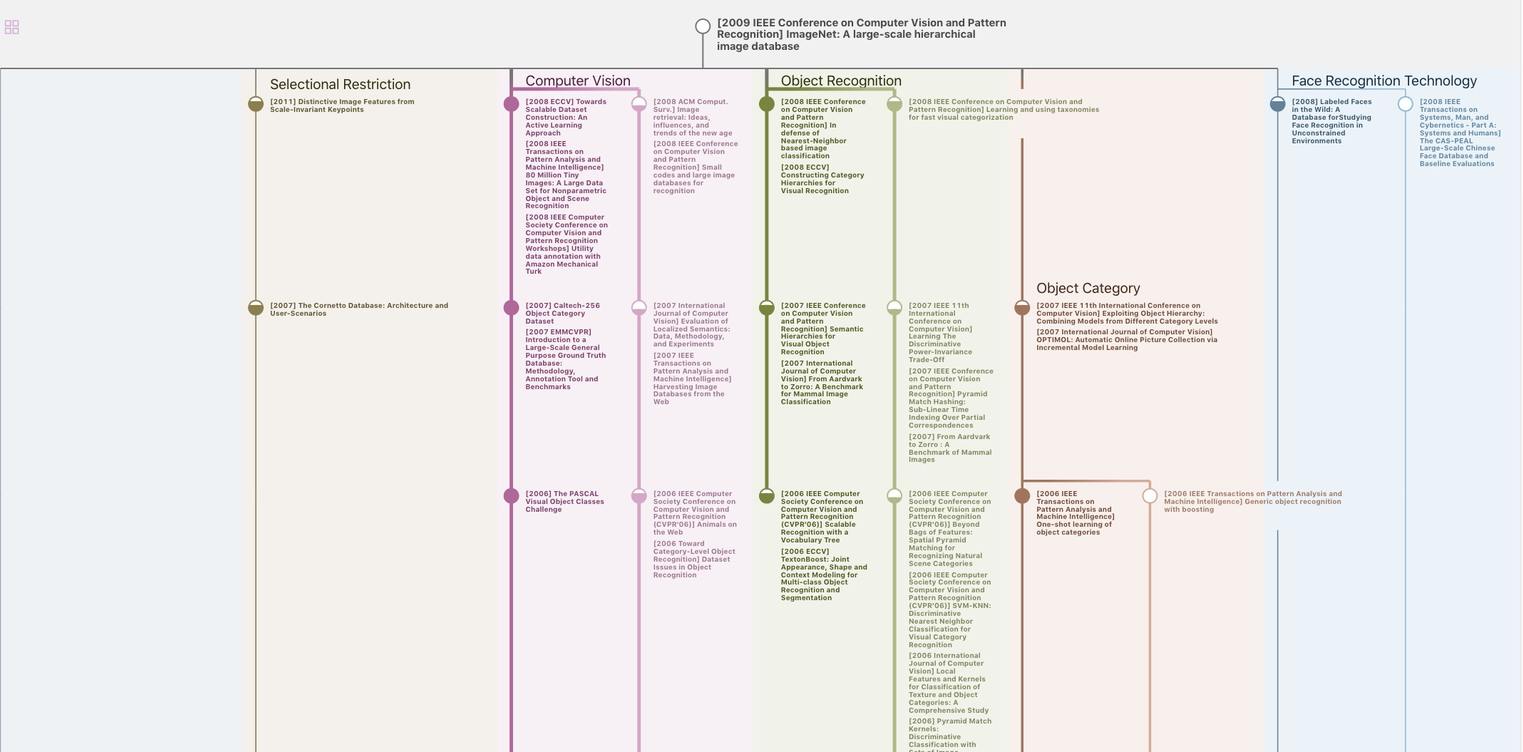
生成溯源树,研究论文发展脉络
Chat Paper
正在生成论文摘要