Model Selection For Estimating Treatment Effects
JOURNAL OF THE ROYAL STATISTICAL SOCIETY SERIES B-STATISTICAL METHODOLOGY(2014)
摘要
Researchers often believe that a treatment's effect on a response may be heterogeneous with respect to certain baseline covariates. This is an important premise of personalized medicine. Several methods for estimating heterogeneous treatment effects have been proposed. However, little attention has been given to the problem of choosing between estimators of treatment effects. Models that best estimate the regression function may not be best for estimating the effect of a treatment; therefore, there is a need for model selection methods that are targeted to treatment effect estimation. We demonstrate an application of the focused information criterion in this setting and develop a treatment effect cross-validation aimed at minimizing treatment effect estimation errors. Theoretically, treatment effect cross-validation has a model selection consistency property when the data splitting ratio is properly chosen. Practically, treatment effect cross-validation has the flexibility to compare different types of models. We illustrate the methods by using simulation studies and data from a clinical trial comparing treatments of patients with human immunodeficiency virus.
更多查看译文
关键词
Causal inference,Cross-validation,Focused information criterion,Model selection,Model selection consistency,Treatment effect estimation
AI 理解论文
溯源树
样例
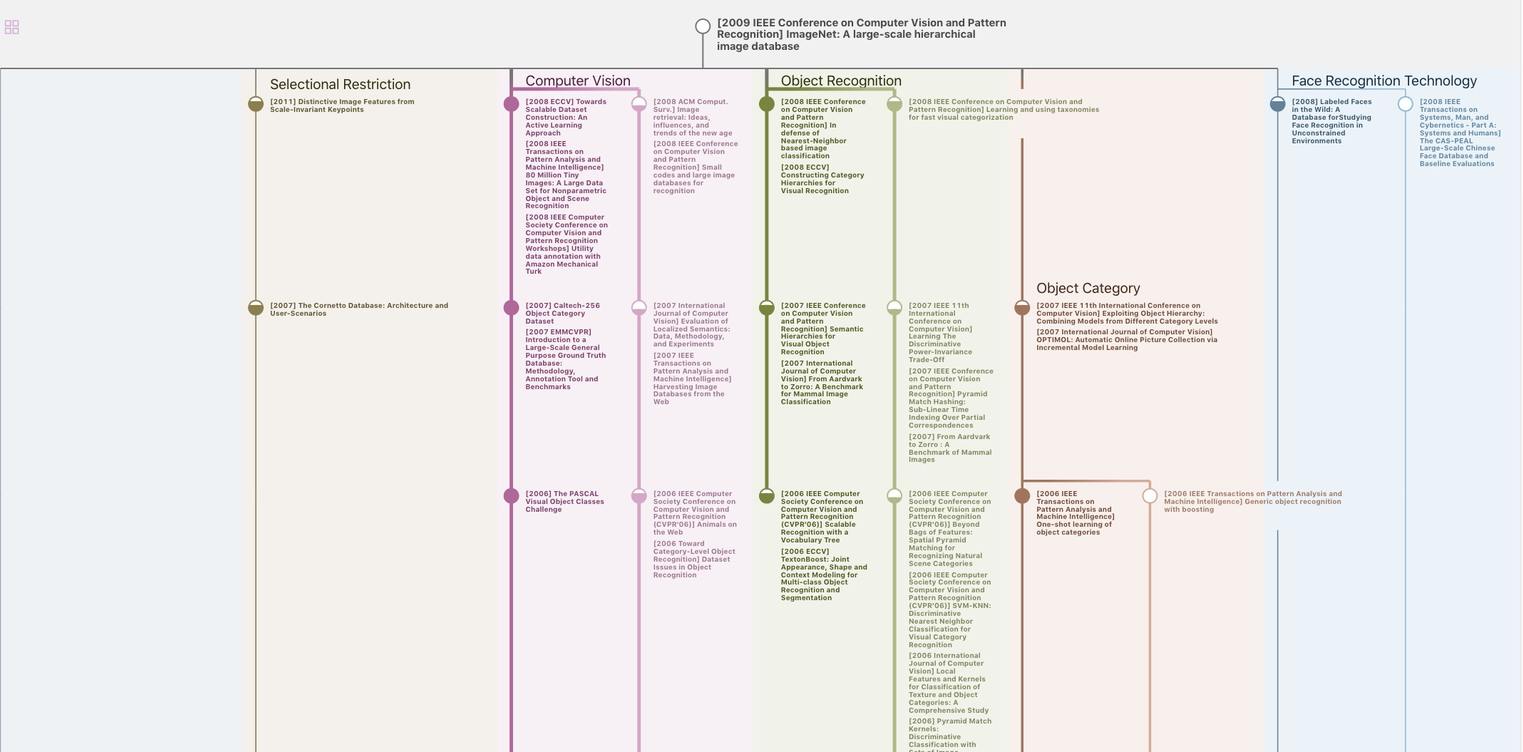
生成溯源树,研究论文发展脉络
Chat Paper
正在生成论文摘要