Using horizon estimation and nonlinear optimization for grey-box identification
Journal of Process Control(2015)
摘要
An established method for grey-box identification is to use maximum-likelihood estimation for the nonlinear case implemented via extended Kalman filtering. In applications of (nonlinear) model predictive control a more and more common approach for the state estimation is to use moving horizon estimation, which employs (nonlinear) optimization directly on a model for a whole batch of data. This paper shows that, in the linear case, horizon estimation may also be used for joint parameter estimation and state estimation, as long as a bias correction based on the Kalman filter is included. For the nonlinear case two special cases are presented where the bias correction can be determined without approximation. A procedure how to approximate the bias correction for general nonlinear systems is also outlined. (C) 2015 Elsevier Ltd. All rights reserved.
更多查看译文
关键词
System identification,State estimation,Parameter estimation,Optimization,Nonlinear systems,Kalman filtering,Moving horizon estimation,Model predictive control
AI 理解论文
溯源树
样例
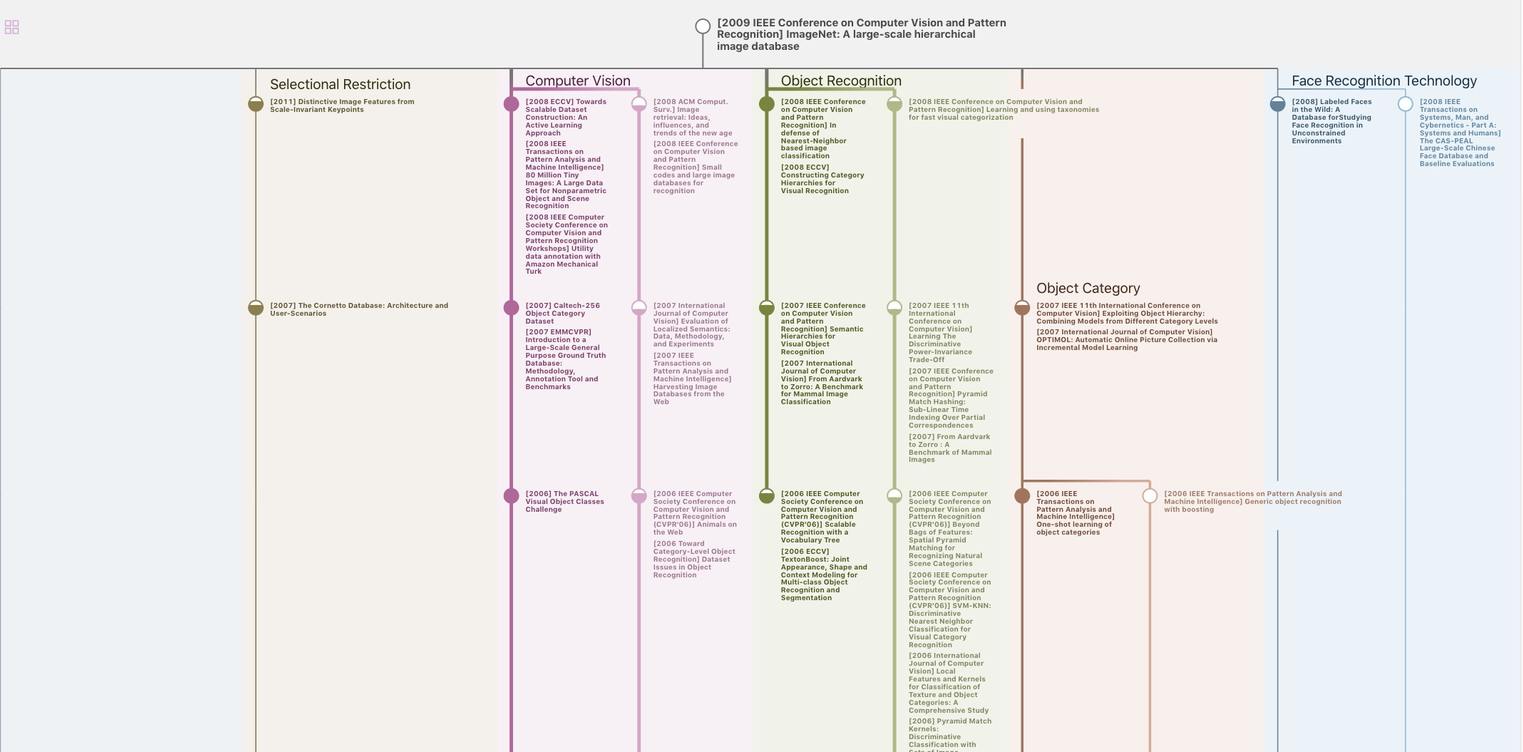
生成溯源树,研究论文发展脉络
Chat Paper
正在生成论文摘要