SHAH: SHape-Adaptive Haar wavelets for image processing
JOURNAL OF COMPUTATIONAL AND GRAPHICAL STATISTICS(2016)
摘要
We propose the shape-adaptive Haar (SHAH) transform for images, which results in an orthonormal, adaptive decomposition of the image into Haar-wavelet-like components, arranged hierarchically according to decreasing importance, whose shapes reflect the features present in the image. The decomposition is as sparse as it can be for piecewise-constant images. It is performed via a stepwise bottom-up algorithm with quadratic computational complexity; however, nearly linear variants also exist. SHAH is rapidly invertible. We show how to use SHAH for image denoising. Having performed the SHAH transform, the coefficients are hard- or soft-thresholded, and the inverse transform taken. The SHAH image denoising algorithm compares favorably to the state of the art for piecewise-constant images. A clear asset of the methodology is its very general scope: it can be used with any images or more generally with any data that can be represented as graphs or networks.
更多查看译文
关键词
Adaptive transformations,Greedy algorithms,Multiscale,Sparsity,Statistical learning
AI 理解论文
溯源树
样例
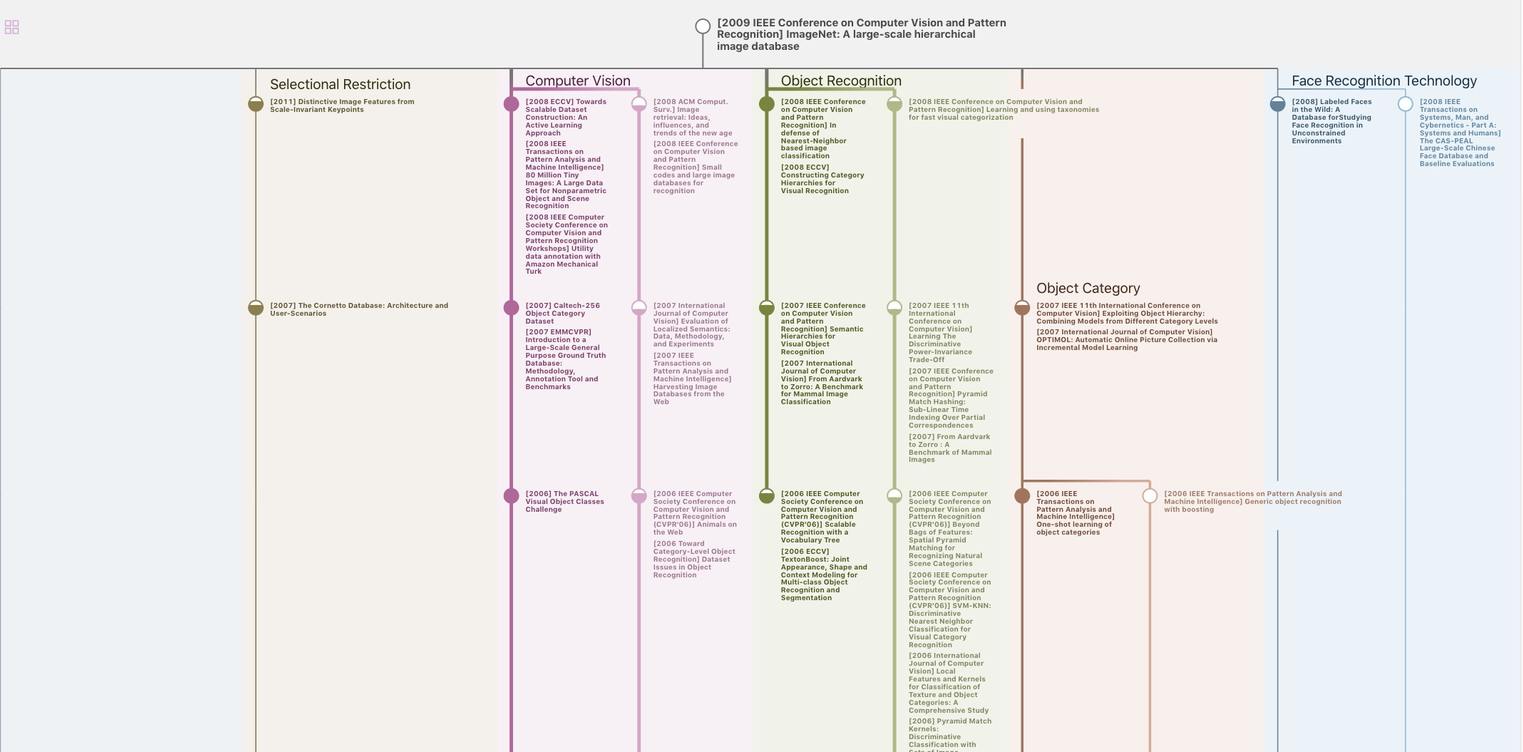
生成溯源树,研究论文发展脉络
Chat Paper
正在生成论文摘要