A Comparison of Artificial Neural Networks, Logistic Regressions, and Classification Trees for Modeling Mental Workload in Real-Time
Proceedings of the Human Factors and Ergonomics Society Annual Meeting(2010)
摘要
The use of eye metrics to predict the state of one's mental workload involves reliable and accurate modeling techniques. This study assessed the workload classification accuracy of three data mining techniques; artificial neural network (ANN), logistic regression, and classification tree. The results showed that the selection of model technique and the interaction between model type and time segmentation have significant effects on the ability to predict an individual's mental workload during a recall task. The ANN and classification tree both performed much better than logistic regression with 1-s incremented data. The classification tree also performed much better with data averaged over the full recall task. In addition, the transparency of the classification tree showed that pupil diameter and divergence are significantly more important predictors than fixation when modeling 1-s incremented data.
更多查看译文
关键词
mental workload,real-time real-time,classification trees,artificial neural networks,neural networks
AI 理解论文
溯源树
样例
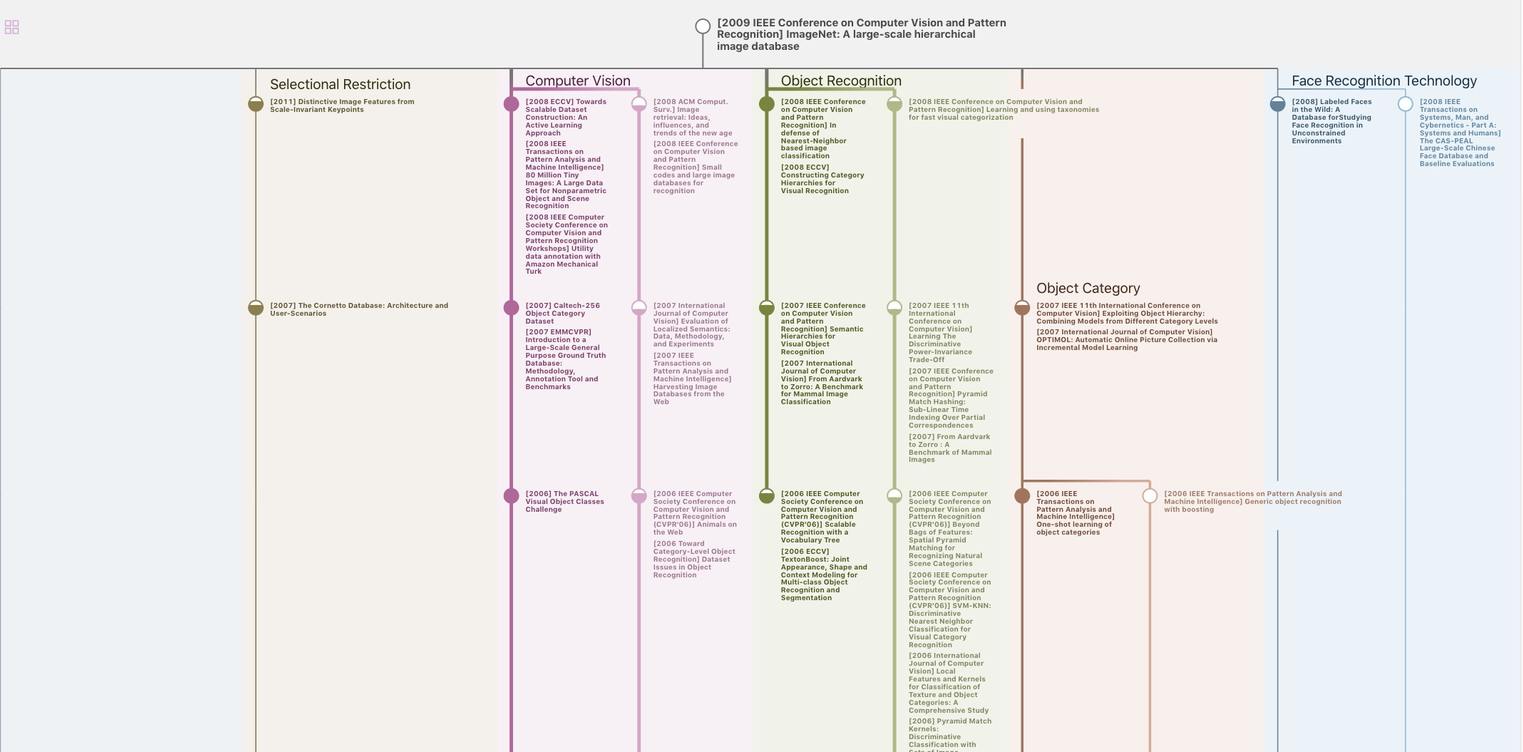
生成溯源树,研究论文发展脉络
Chat Paper
正在生成论文摘要