Online Markov decision processes with policy iteration.
IEICE technical report. Speech(2015)
摘要
The online Markov decision process (MDP) is a generalization of the classical Markov decision process that incorporates changing reward functions. In this paper, we propose practical online MDP algorithms with policy iteration and theoretically establish a sublinear regret bound. A notable advantage of the proposed algorithm is that it can be easily combined with function approximation, and thus large and possibly continuous state spaces can be efficiently handled. Through experiments, we demonstrate the usefulness of the proposed algorithm.
更多查看译文
关键词
reinforcement learning,markov decision process
AI 理解论文
溯源树
样例
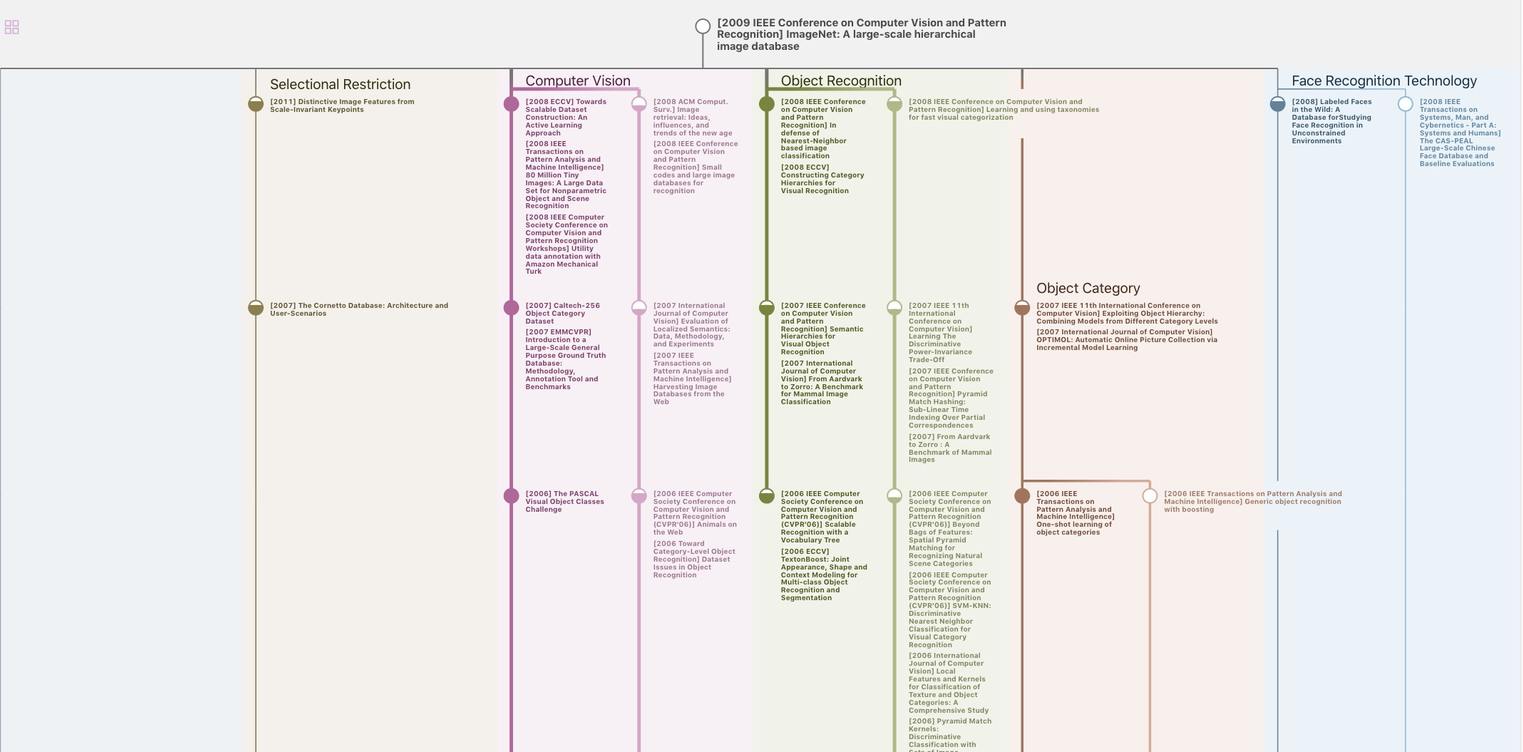
生成溯源树,研究论文发展脉络
Chat Paper
正在生成论文摘要