Study of cover source mismatch in steganalysis and ways to mitigate its impact
Proceedings of SPIE(2014)
摘要
When a steganalysis detector trained on one cover source is applied to images from a different source, generally the detection error increases due to the mismatch between both sources. In steganography, this situation is recognized as the so-called cover source mismatch (CSM). The drop in detection accuracy depends on many factors, including the properties of both sources, the detector construction, the feature space used to represent the covers, and the steganographic algorithm. Although well recognized as the single most important factor negatively affecting the performance of steganalyzers in practice, the CSM received surprisingly little attention from researchers. One of the reasons for this is the diversity with which the CSM can manifest. On a series of experiments in the spatial and JPEG domains, we refute some of the common misconceptions that the severity of the CSM is tied to the feature dimensionality or their "fragility." The CSM impact on detection appears too difficult to predict due to the effect of complex dependencies among the features. We also investigate ways to mitigate the negative effect of the CSM using simple measures, such as by enlarging the diversity of the training set (training on a mixture of sources) and by employing a bank of detectors trained on multiple different sources and testing on a detector trained on the closest source.
更多查看译文
关键词
Steganalysis,steganography,cover source mismatch,machine learning
AI 理解论文
溯源树
样例
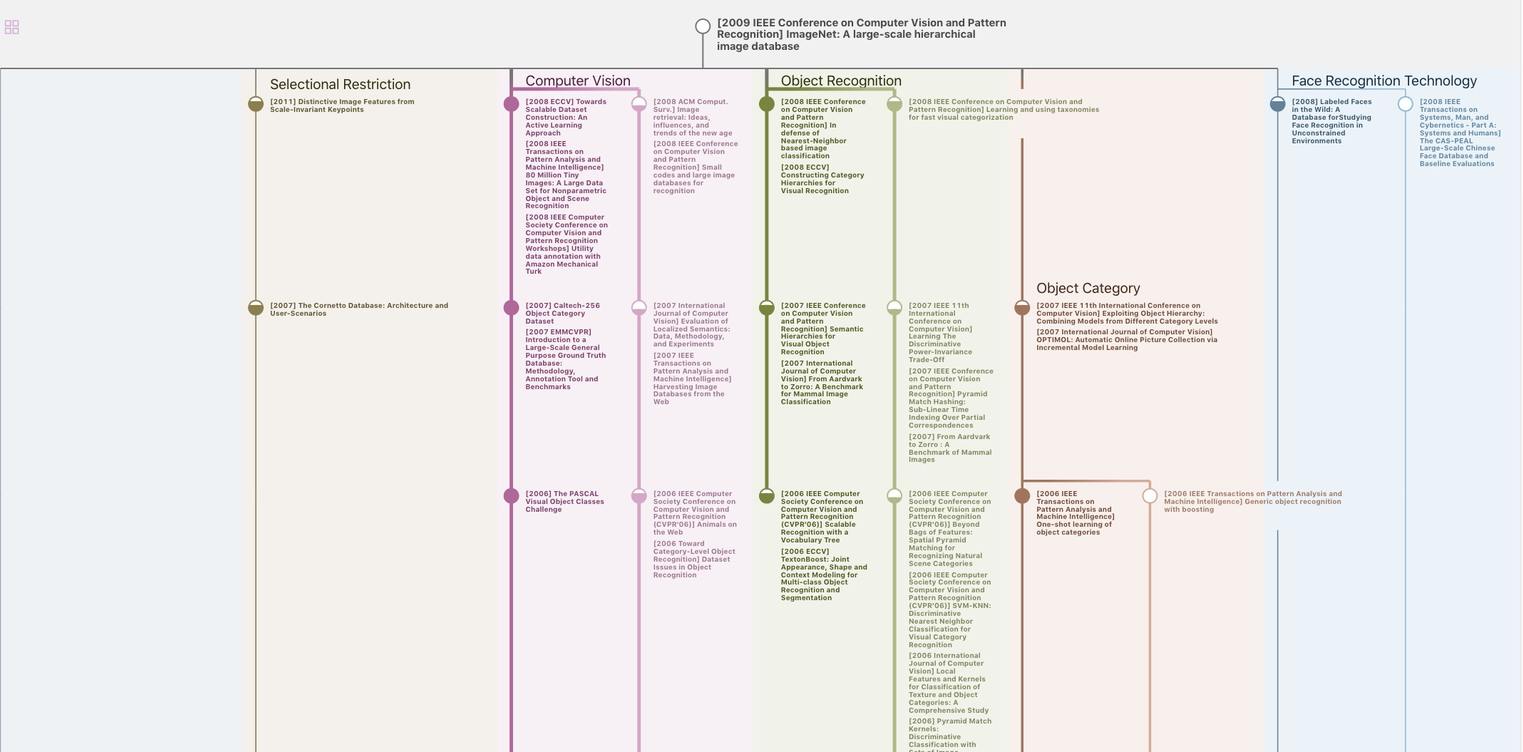
生成溯源树,研究论文发展脉络
Chat Paper
正在生成论文摘要