Unsupervised Learning Of Categories With Local Feature Sets Of Image
2013 FIRST IRANIAN CONFERENCE ON PATTERN RECOGNITION AND IMAGE ANALYSIS (PRIA)(2013)
摘要
By an increase in the volume of image data in the age of information, a need for image categorization systems is greatly felt. Recent activities in this area has shown that the image description by local features, often has very strong similarities among local partials of an image, but these methods are also challenging, because the use of a set of vectors for each image does not permit the direct use of most of the common learning methods and distance functions. On the other hand, measuring the created similarities of the collection of unordered features is also problematic, because most of the proposed methods have rather high time complexities computationally.In this article, an unsupervised learning method of categorization of objects from a collection of unlabeled images is introduced. Each image is described by a set of unordered local features and clustering is performed on the basis of partial similarities existing among these sets of features. For this purpose, by the use of pyramid match algorithm, the set of the features are mapped in multi-resolution histograms and two sets of feature vectors in time-line are calculated in this new distance. These similarities are employed as a criterion distance among the patterns in hierarchical clustering; therefore, the categorization of objects by the use of common learning methods is performed with acceptable accuracy and faster than the existing algorithms.
更多查看译文
关键词
Computer vision, unsupervised learning, Categorization of objects, Pyramid match algorithm, Hierarchical clustering
AI 理解论文
溯源树
样例
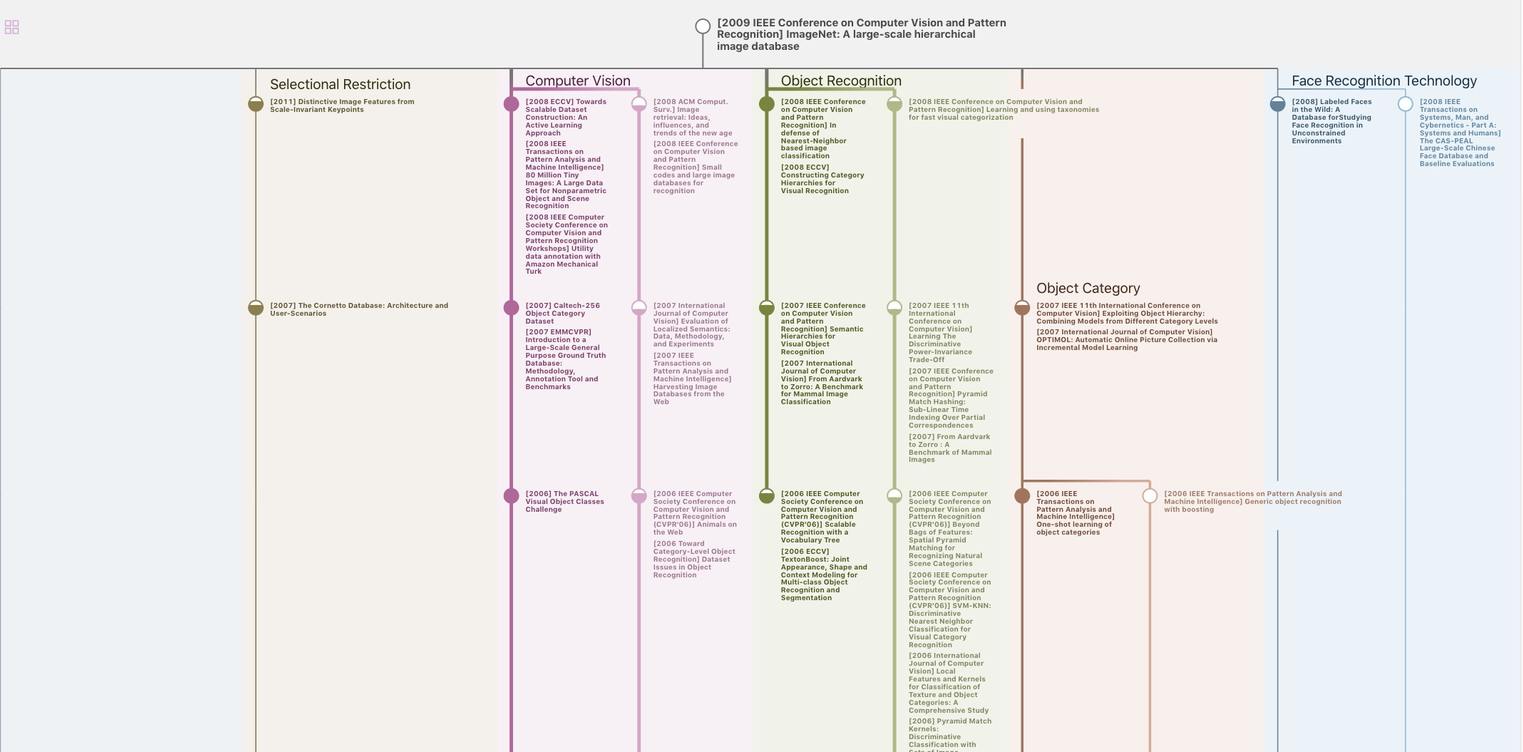
生成溯源树,研究论文发展脉络
Chat Paper
正在生成论文摘要