A Note on Automatic Data Transformation
STAT(2016)
摘要
Modern data analysis frequently involves variables with highly non-Gaussian marginal distributions. However, commonly used analysis methods are most effective with roughly Gaussian data. This paper introduces an automatic transformation that improves the closeness of distributions to normality. For each variable, a new family of parametrizations of the shifted logarithm transformation is proposed, which is unique in treating the data as real valued and in allowing transformation for both left and right skewness within the single family. This also allows an automatic selection of the parameter value (which is crucial for high-dimensional data with many variables to transform) by minimizing the Anderson-Darling test statistic of the transformed data. An application to image features extracted from melanoma microscopy slides demonstrates the utility of the proposed transformation in addressing data with excessive skewness, heteroscedasticity and influential observations. Copyright (C) 2016 John Wiley & Sons, Ltd.
更多查看译文
关键词
Anderson-Darling test statistics,automatic transformation,heteroscedasticity,shifted logarithm transformation
AI 理解论文
溯源树
样例
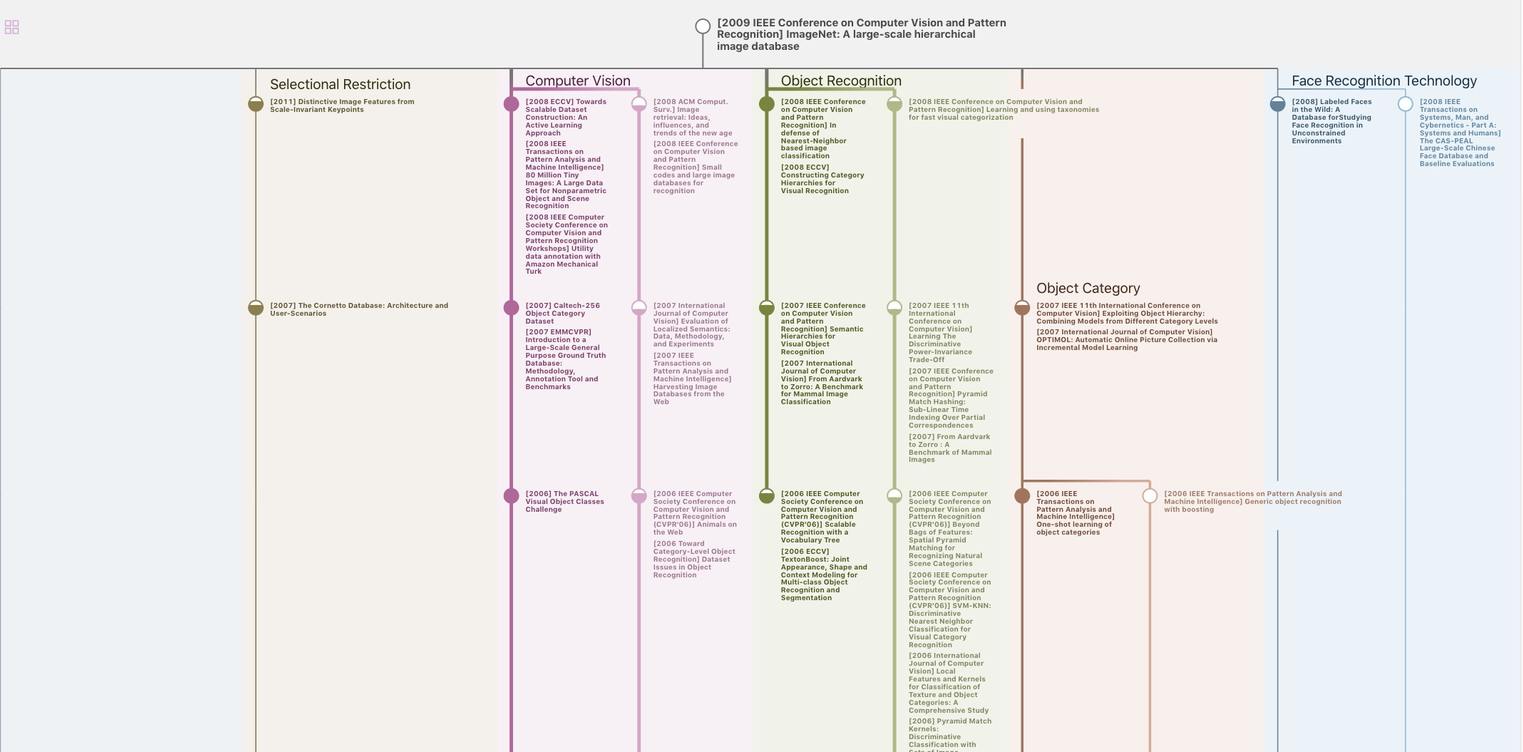
生成溯源树,研究论文发展脉络
Chat Paper
正在生成论文摘要