A baseline algorithm for face detection and tracking in video
PROCEEDINGS OF THE SOCIETY OF PHOTO-OPTICAL INSTRUMENTATION ENGINEERS (SPIE)(2007)
摘要
Establishing benchmark datasets, performance metrics and baseline algorithms have considerable research significance in gauging the progress in any application domain. These primarily allow both users and developers to compare the performance of various algorithms on a common platform. In our earlier works, we focused on developing performance metrics and establishing a substantial dataset with ground truth for object detection and tracking tasks (text and face) in two video domains - broadcast news and meetings. In this paper, we present the results of a face detection and tracking algorithm on broadcast news videos with the objective of establishing a baseline performance for this task-domain pair. The detection algorithm uses a statistical approach that was originally developed by Viola and Jones and later extended by Lienhart. The algorithm uses a feature set that is Haar-like and a cascade of boosted decision tree classifiers as a statistical model. In this work, we used the Intel Open Source Computer Vision Library (OpenCV) implementation of the Haar face detection algorithm. The optimal values for the tunable parameters of this implementation were found through an experimental design strategy commonly used in statistical analyses of industrial processes. Tracking was accomplished as continuous detection with the detected objects in two frames mapped using a greedy algorithm based on the distances between the centroids of bounding boxes. Results on the evaluation set containing 50 sequences (approximate to 2.5 mins.) using the developed performance metrics show good performance of the algorithm reflecting the state-of-the-art which makes it an appropriate choice as the baseline algorithm for the problem.
更多查看译文
关键词
baseline algorithm,face detection & tracking,broadcast news,performance evaluation
AI 理解论文
溯源树
样例
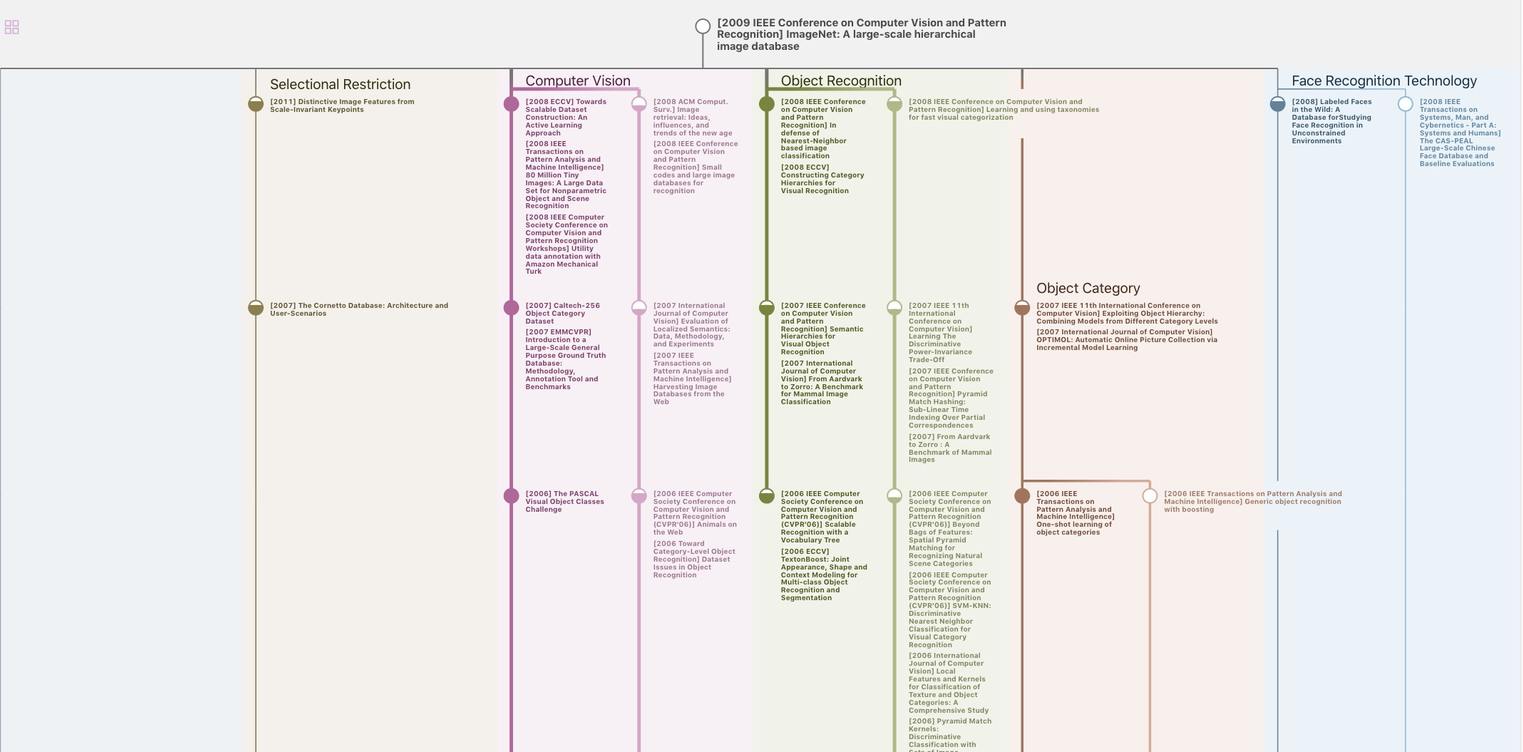
生成溯源树,研究论文发展脉络
Chat Paper
正在生成论文摘要