Sparse Approximate Inference for Spatio-Temporal Point Process Models
JOURNAL OF THE AMERICAN STATISTICAL ASSOCIATION(2016)
摘要
Spatio-temporal log-Gaussian Cox process models play a central role in the analysis of spatially distributed systems in several disciplines. Yet, scalable inference remains computationally challenging both due to the high-resolution modeling generally required and the analytically intractable likelihood function. Here, we exploit the sparsity structure typical of (spatially) discretized log-Gaussian Cox process models by using approximate message-passing algorithms. The proposed algorithms scale well with the state dimension and the length of the temporal horizon with moderate loss in distributional accuracy. They hence provide a flexible and faster alternative to both nonlinear filtering-smoothing type algorithms and to approaches that implement the Laplace method or expectation propagation on (block) sparse latent Gaussian models. We infer the parameters of the latent Gaussian model using a structured variational Bayes approach. We demonstrate the proposed framework on simulation studies with both Gaussian and point-process observations and use it to reconstruct the conflict intensity and dynamics in Afghanistan from the WikiLeaks Afghan War Diary. Supplementary materials for this article are available online.
更多查看译文
关键词
Conflict analysis,Expectation propagation,Latent Gaussian models,Log-Gaussian Cox process,Sparse approximate inference,Structure learning,Variational approximate inference
AI 理解论文
溯源树
样例
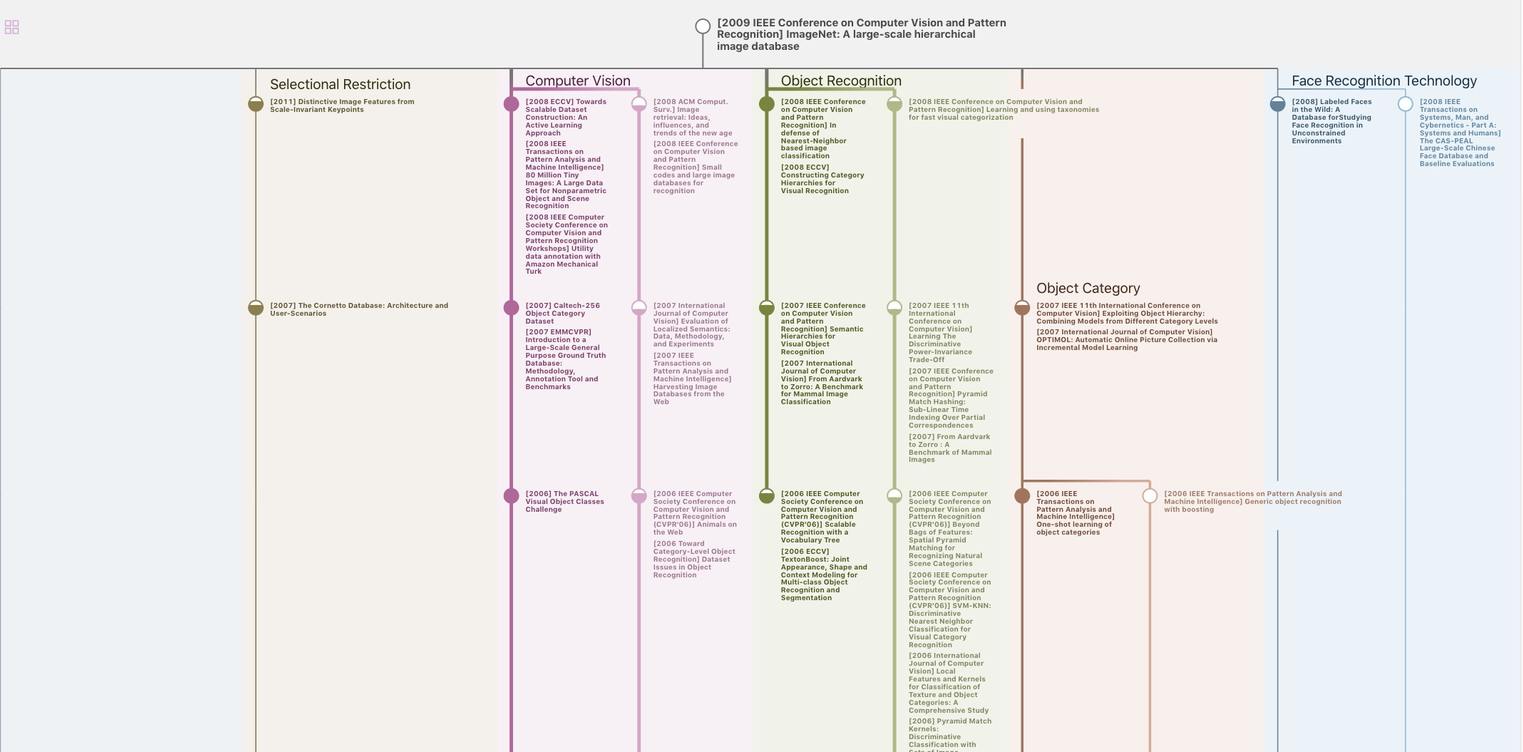
生成溯源树,研究论文发展脉络
Chat Paper
正在生成论文摘要