Entity Resolution for Uncertain Data
mag(2012)
摘要
Entity resolution (ER), also known as duplicate detection or record matching, is the problem of identifying the tuples that represent the same real world entity. In this paper, we address the problem of ER for uncertain data, which we call ERUD. We propose two different approaches for the ERUD problem based on two classes of similarity functions, i.e. context-free and context-sensitive. We propose a PTIME algorithm for context-free similarity functions, and a Monte Carlo algorithm for context-sensitive similarity functions. Existing context-sensitive similarity functions need at least one pass over the database to compute some statistical features of data, which makes it very ine ffi cient for our Monte Carlo algorithm. Thus, we propose a novel context-sensitive similarity function that makes our Monte Carlo algorithm more e ffi cient. To further improve the e ffi ciency of our proposed Monte Carlo algorithm, we propose a parallel version of it using the MapReduce framework. We validated our algorithms through experiments over both synthetic and real datasets. Our performance evaluation shows the e ff ectiveness of our algorithms in terms of success rate and response time.
更多查看译文
AI 理解论文
溯源树
样例
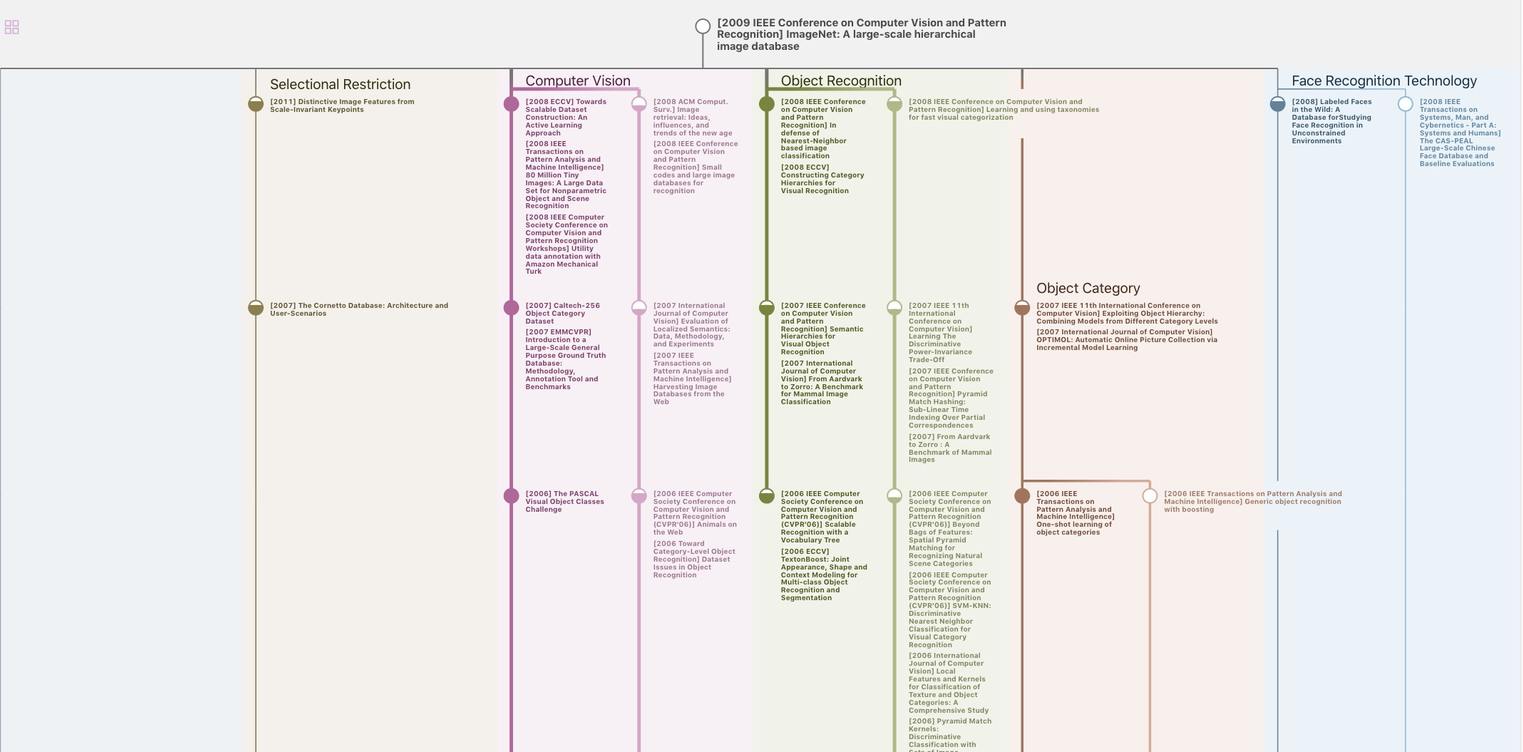
生成溯源树,研究论文发展脉络
Chat Paper
正在生成论文摘要