Oc-Wad: A One-Class Classifier Ensemble Approach For Anomaly Detection In Web Traffic
2015 23RD IRANIAN CONFERENCE ON ELECTRICAL ENGINEERING (ICEE)(2015)
摘要
In recent years, web-based attacks have made up a substantial portion of all security attacks because web-based vulnerabilities are so common and so easy to exploit. To counter these attacks, many anomaly detection systems have been proposed that are able to detect both known and unknown attacks launched against web-based applications. However, most of them suffer from a large number of false alarms. In this paper, we address this problem by presenting OC-WAD, a novel approach to construct an ensemble of one-class SVM classifiers for anomaly detection in web traffic. OC-WAD uses a novel binary artificial bee colony algorithm, called BeeSnips, to prune the initial ensemble of one-class SVM classifiers and to find a near-optimal sub-ensemble. It is motivated by the observation that the fusion of multiple one-class classifiers can considerably decrease the false alarm rate without a significant change in the detection rate. The results of experiments carried out on a real dataset show that OC-WAD can detect web-based attacks with a high detection rate and an acceptable false alarm rate.
更多查看译文
关键词
anomaly detection, web-based attack, artificial bee colony algorithm, one-class classifier, classifier ensemble
AI 理解论文
溯源树
样例
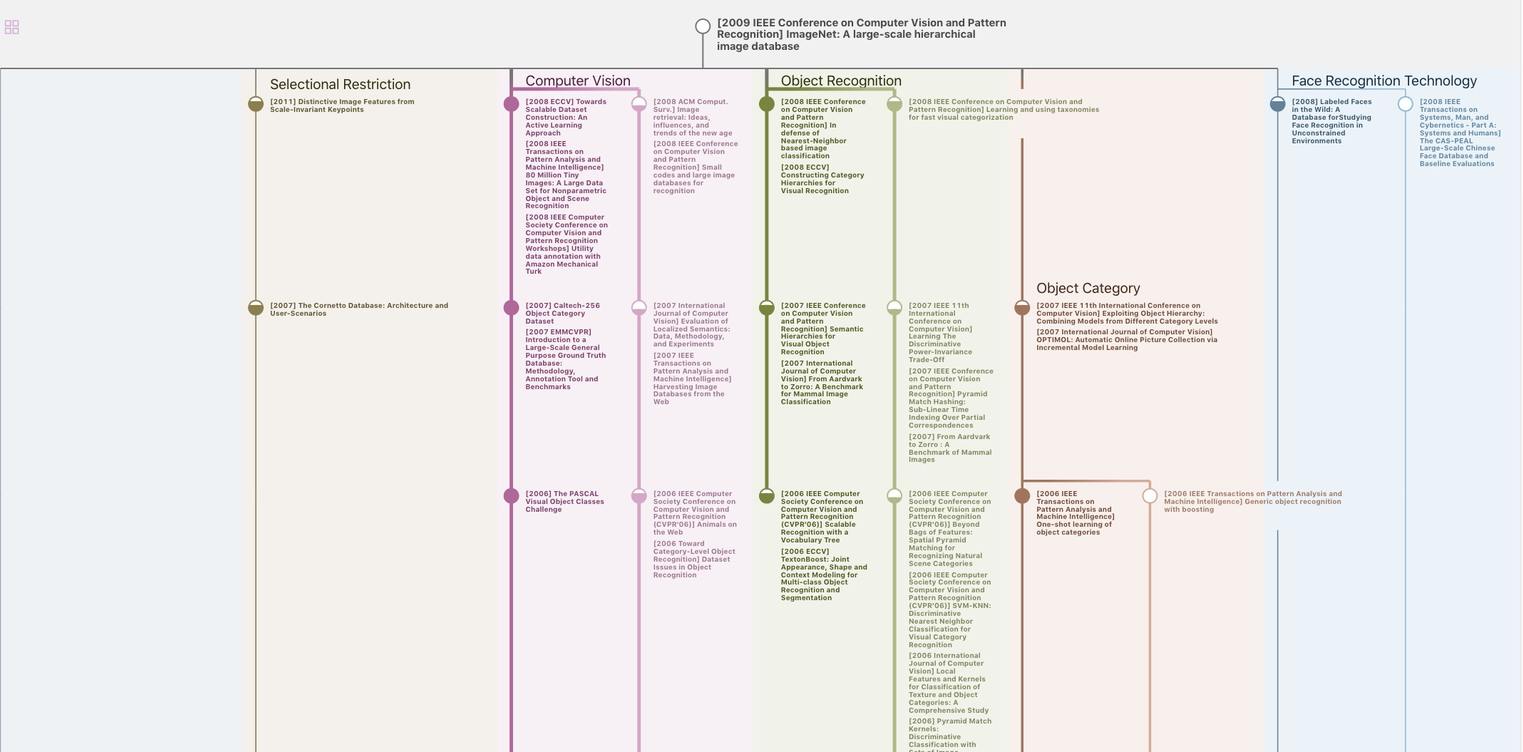
生成溯源树,研究论文发展脉络
Chat Paper
正在生成论文摘要