Modeling And Learning Behaviors
HANDBOOK OF INTELLIGENT VEHICLES, VOLS 1 AND 2(2012)
摘要
In order to safely navigate in a dynamic environment, a robot requires to know how the objects populating it will move in the future. Since this knowledge is seldom available, it is necessary to resort to motion prediction algorithms. Due to the difficulty of modeling the various factors that determine motion (e.g., internal state, perception), this is often done by applying machine-learning techniques to build a statistical model, using as input a collection of trajectories array through a sensor (e.g., camera, laser scanner), and then using that model to predict further motion.This section describes the basic concepts involved in current motion learning and prediction approaches. After introducing the Bayes filter, it discusses Growing Hidden Markov Models, an approach which is able to perform lifelong learning, continuously updating its knowledge as more data are available. In experimental evaluation against two other state-of-the-art approaches, the presented approach consistently outperforms them regarding both prediction accuracy and model parsimony.The section concludes with an overview of the current challenges and future research directions for motion modeling and learning algorithms.
更多查看译文
AI 理解论文
溯源树
样例
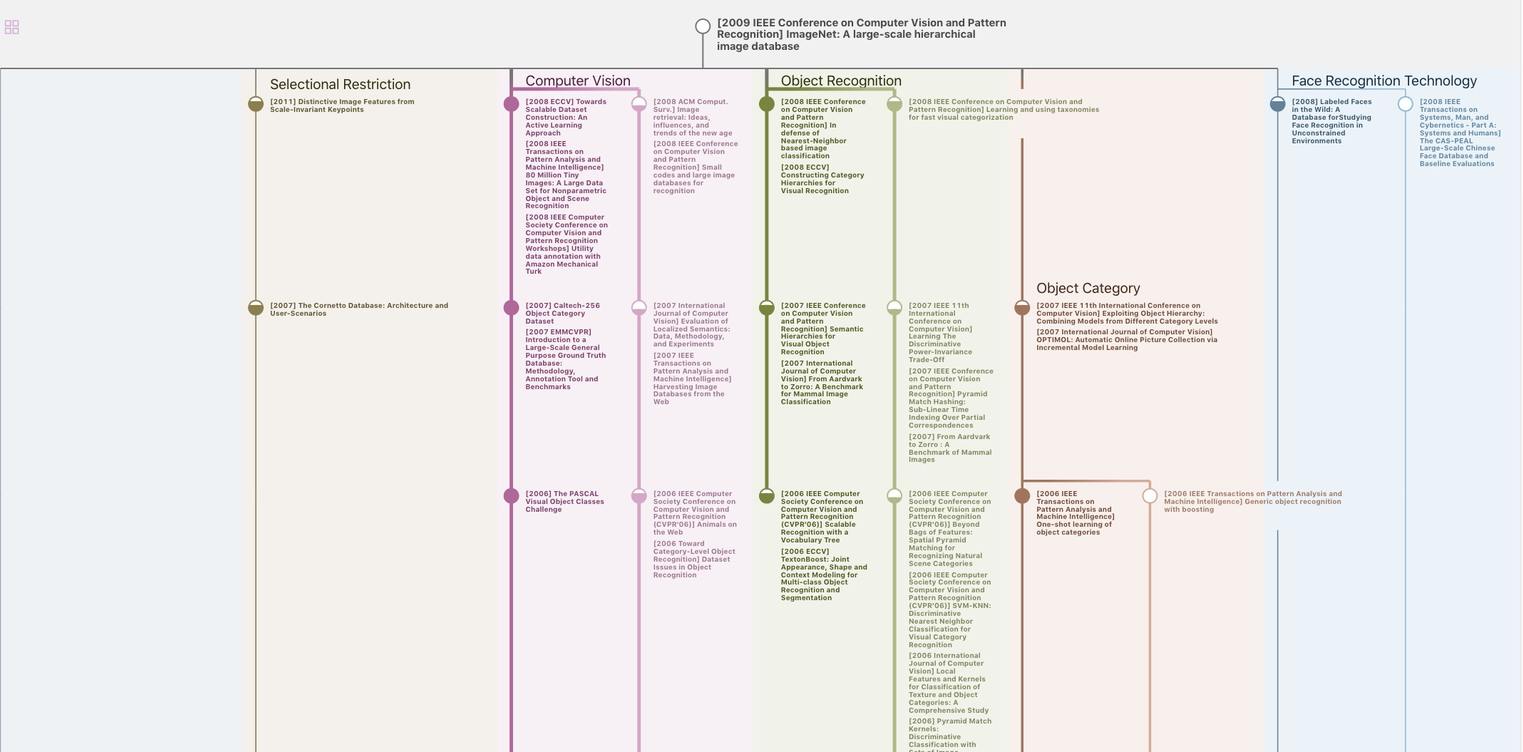
生成溯源树,研究论文发展脉络
Chat Paper
正在生成论文摘要