Internet traffic classification using multiple classifiers
2015 7th Conference on Information and Knowledge Technology (IKT)(2015)
摘要
In this work, we propose a novel scheme for internet traffic classification using combination of three different classifiers. The proposed classification scheme consists of three steps. In the first step, in order to achieve discrete features, the size of the first four packets of each flow is discretized based on an entropy-based algorithm. In the next step, three classifiers including K-NN, SVM and Naive Bayes are employed to determine the label of unknown flows. In the last step, the outputs of three classifiers are combined using four combiner schemes including Dynamic Classifier Selection by Local Accuracy (DCS-LA), Naive Bayes (NB), Majority Voting (MV) and Oracle in order to make final decision on the label of unknown flows and achieve the highest possible accuracy. We conduct experiments on a dataset including only 50 training flow per application to evaluate the effectiveness of our classification scheme. The results indicate that our proposed internet traffic classification scheme is able to achieve a high level of accuracy.
更多查看译文
关键词
Internet traffic classification,multiple classifiers,entropy-based algorithm,Naive Bayes classifiers,SVM,K-NN classifiers,dynamic classifier selection by local accuracy,DCS-LA,majority voting,Oracle
AI 理解论文
溯源树
样例
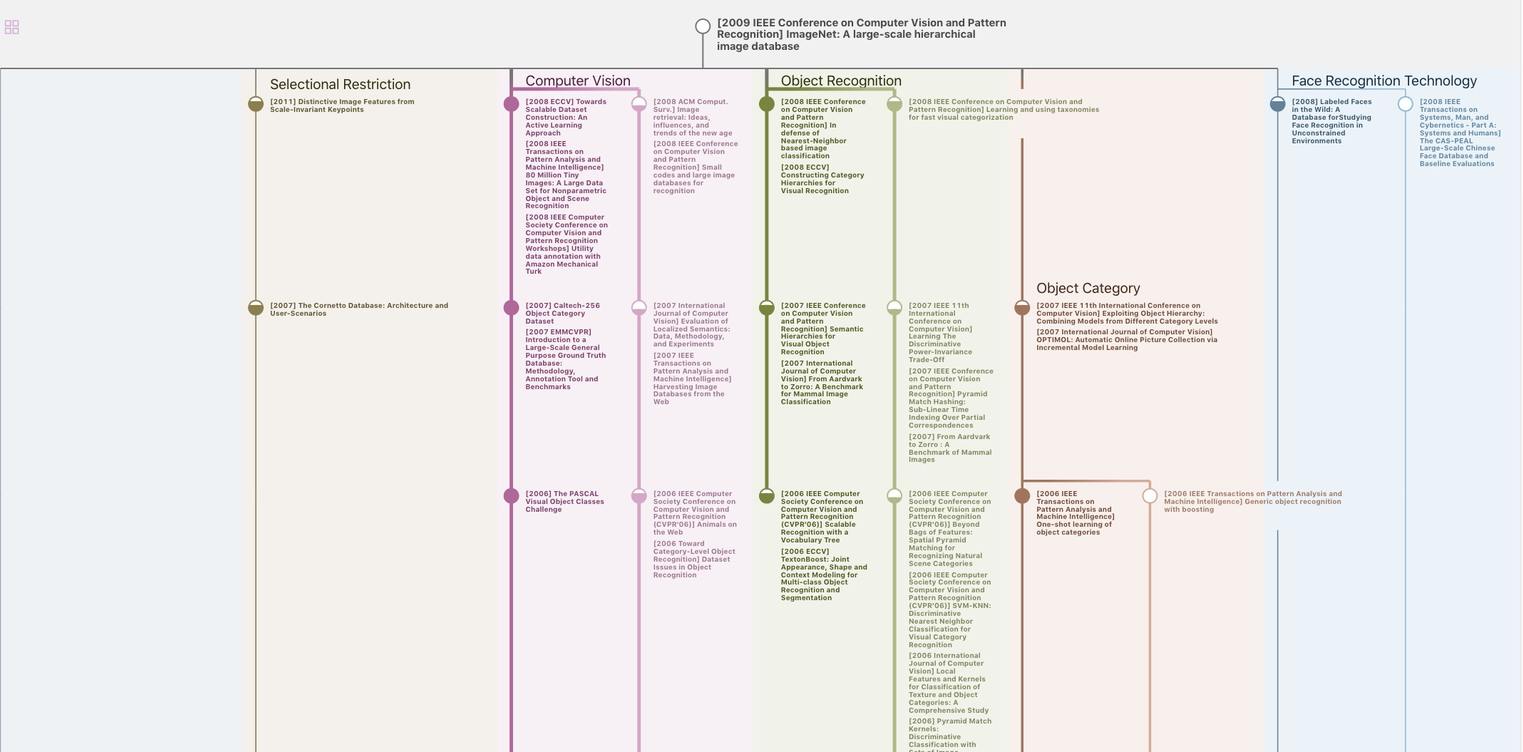
生成溯源树,研究论文发展脉络
Chat Paper
正在生成论文摘要