A Matrix-Based Incremental Attribute Reduction Approach under Knowledge Granularity on the Variation of Attribute Set
2015 10th International Conference on Intelligent Systems and Knowledge Engineering (ISKE)(2015)
摘要
Attribute reduction in rough sets is a key step to discover interesting patterns in decision systems with numbers of attributes available. Moreover, data processing tools have been developed rapidly in recent years, and then the information system may increase quickly in attributes with time in real-life applications. How to update attribute reduction efficiently becomes an important task in knowledge discovery related tasks. The attribute reduction of information systems may alter with the attributes increasing. This paper aims for investigation of incremental attribute reduction algorithm based on knowledge granularity in information systems on the variation of attribute set. Matrix-based incremental mechanisms to calculate the new knowledge granularity are first introduced. Then, the corresponding incremental algorithm is presented for attribute reduction based on the calculated knowledge granularity when multiple attributes are added to a decision table. Finally, experiments performed on UCI data sets and the complexity analysis show that the proposed matrix-based incremental method is effective and efficient to update attribute reduction with the increase of attributes.
更多查看译文
关键词
Decision table,knowledge granularity,attribute reduction,incremental learning,rough set theory
AI 理解论文
溯源树
样例
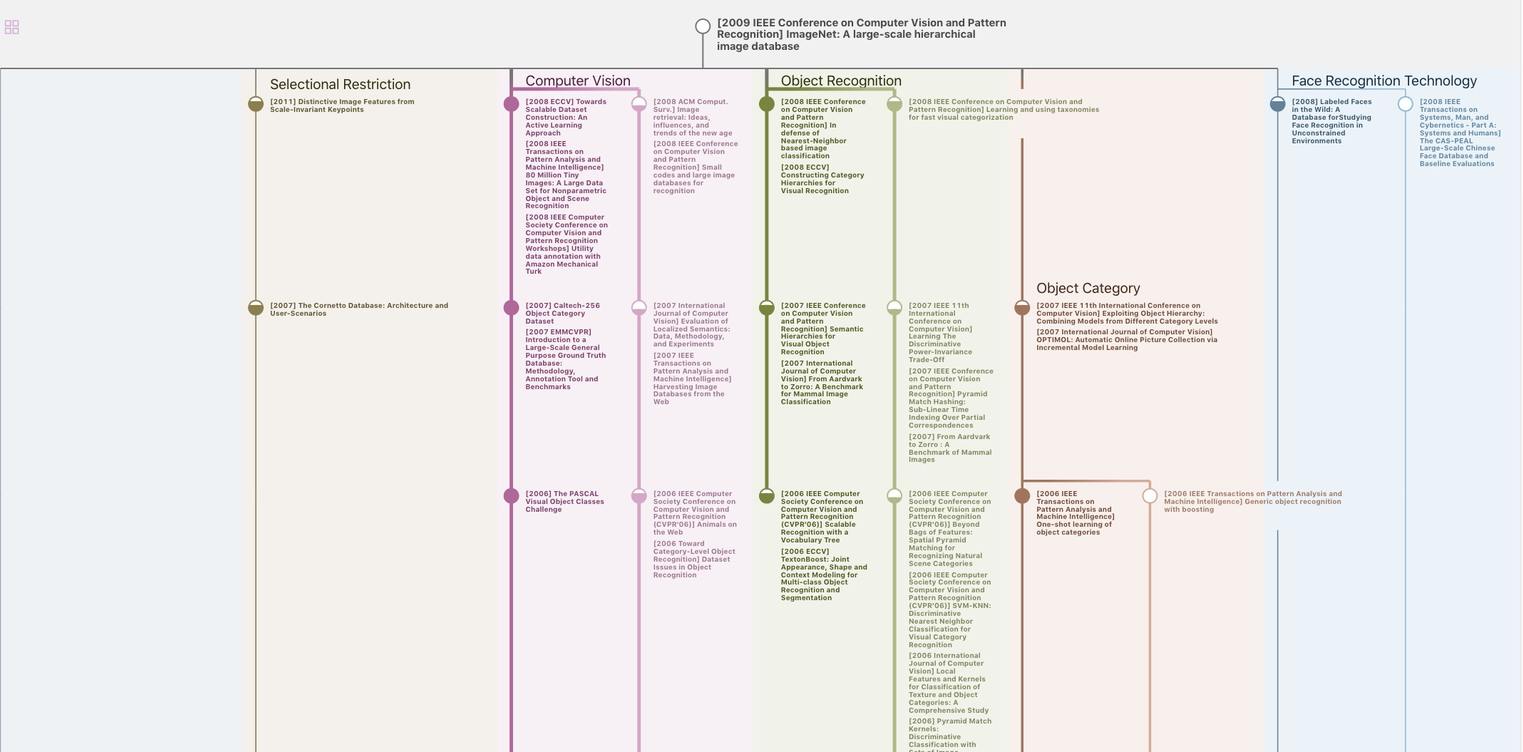
生成溯源树,研究论文发展脉络
Chat Paper
正在生成论文摘要