Statistical Machine Learning for HCI
2013 INTERNATIONAL CONFERENCE ON COMPUTER SCIENCES AND APPLICATIONS (CSA)(2010)
摘要
This chapter introduces the main concepts of statistical machine learning, as they are pivotal in most algorithms tailored for multimodal signal processing. It provides introduction to machine learning and how it is used in classification, regression, and density estimation. Following this introduction, two particularly well-known models is presented, together with their associated learning algorithm: support vector machines (SVM), which are well known for classification tasks; and hidden Markov models (HMM), which are tailored for sequence processing tasks such as speech recognition. The most well-known machine learning approach is the SVM. It has been widely used in the last decade in various applications. Although its main successes are for classification problems, extensions of the main approach to regression, density estimation, ranking, and many others have been proposed. SVMs have been used in many successful applications, and several off-the-shelf implementations are now readily available on the Web. The main limitation remains its training computational complexity, which grows at least quadratically with the number of examples that hence limits the use of SVMs to problems with less than a few hundreds of thousands training examples. Whereas, HMMs have been the preferred approach for many sequence processing applications, including most speech processing problems such as speech recognition, speaker verification, keyword detection, and speaker segmentation, for the last 20 years or so.
更多查看译文
关键词
statistical machine learning,machine learning,hci
AI 理解论文
溯源树
样例
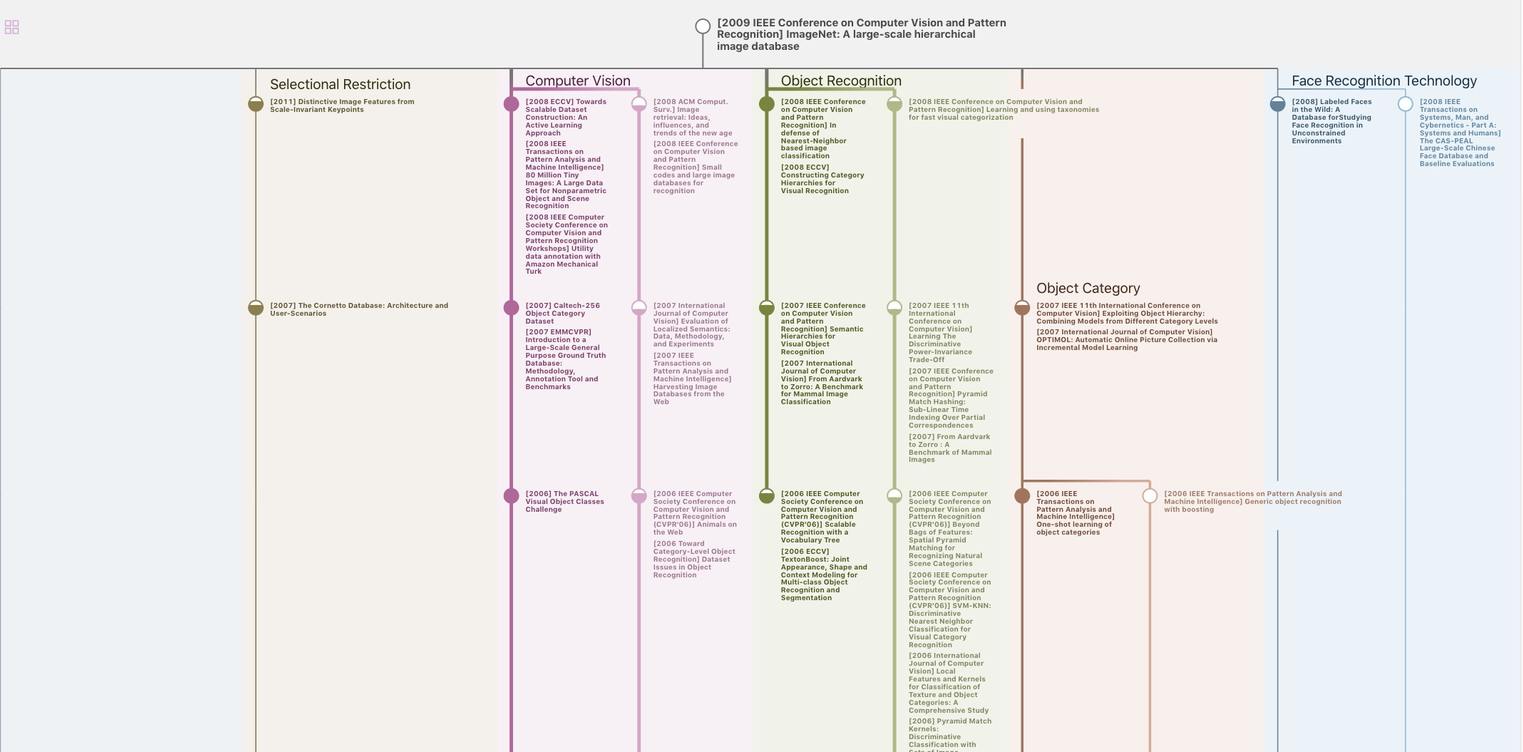
生成溯源树,研究论文发展脉络
Chat Paper
正在生成论文摘要